Tetiana Telenczak, Director of Product Management, Merck Group, gave this presentation at the Product-Led Summit.
Hi, I’m Tetiana Telenczak. Currently, I'm responsible for the global data analytics platform at a company called Merck. Merck is a classic example of a large corporate organization. It has business in life science, healthcare, and electronics.
We have around 65,000 employees, which means that around 10% of these employees are monthly active users of our platform. It also means that every 10th employee works with data in their day-to-day job.
I don't have a typical product management career path. In the past, I worked as a customer success team lead at an AI tech company called Dataiku, which also sells a data science platform. For those of you who are thinking of buying one, it's a really great product.
Before that, I used to run a lot of analytics projects as a consultant at PwC. I worked with a lot of large organizations that were trying to be data-driven.
My presentation today is a combination of market observations and my personal experiences working with clients in Germany, Switzerland, and Austria.
- The road to becoming a data-driven company
- The product manager universe in data science
- Every building block of the analytics journey needs product thinking
- Building an analytics solution for an external market
- The organizational set-up of an AI product company
The road to becoming a data-driven company
So let's have a look at a typical journey of a German corporate company that wants to be data-driven. You normally have someone in the management team who’s heard about AI. Currently, everyone talks about generative AI. Last year, it was data mesh, and a couple of years ago, it was neural networks. For those who are in this field, there’s always something new coming.
So, someone in the management team heard about it. Maybe even your competitors are doing this right now. So you make the decision that you need AI in the company.
Very often, it starts with two initiatives. You hire data scientists, sometimes dozens of data scientists, and you start to build a data lake. You want to be a data-driven company, so you put all the data you have in one place.
And then it goes further and you realize that it's just beginning. So then you hire data engineers because you realize that you need to build data pipelines or you need someone who’s responsible for integration between the systems.
Or you realize as a data scientist, I can’t just work with open source software and then just run my model locally on a machine. I need a proper enterprise stack.
This is a very hard journey for a traditional company. At this point in time, you’ve already burned several million euros because you’ve hired people and launched initiatives. You may work with a consultancy firm because you need some external consultants to get the job done.
And it goes further because you also need a data architect to define what your stack should look like, and so that the data engineers get guidance on which ways they can build pipelines.
Or you have a huge data lake. But do you know where your data is? Do you know how to access it? Do you follow all the data protection regulations? What if your use case has personally identifiable information (PII)?
At this point in time, management in the company is getting very overwhelmed because they ask themselves, “What is my return on investment? There are so many technical companies like Google and Meta who are always so successful with AI, Why am I failing? I don't have any value from this community or tech experts in my company.”
Here’s a real-life example. I took this post from LinkedIn, and it's from January 2023. It's not a corporate company, but it's the German Bundeskanzleramt, which is the Federal Chancellery.
They decided, in a similar way to other companies, that they wanted to be data-driven and build a data analytics department.
They were looking for a chief data officer, a manager, a data scientist, a data engineer, and a data analyst, so someone who also has some domain knowledge. A data architect is missing there, but I assume they’ll come back to that later.
But in my opinion, there's one other role that’s missing.
When management asks, “What’s the value of this initiative? What’s the value of AI?” This missing role is the only person who can answer those questions: it’s the product manager.
This person, in the same way as a product manager in any other software company or on any other product, has the same responsibility. It's the person who defines the proper scope, talks to the users, gets senior stakeholder alignment, and is responsible for overall success, whatever that means, just for this product to be successful and have high adoption among users.
The product manager universe in data science
For those of us who are closer to the AI space, there’s a statistic that says 80-90% of all analytics use cases will never make it to production.
There are two main reasons why a particular analytics use case isn’t successful. The first reason is wrong scoping. The second one is the inability to operationalize.
If we talk about the inability to operationalize, it's mostly a technical issue because you need to have a proper stack. But you also need to have people who know how to do it. So it’s a technology and skills issue.
And wrong scoping can be nicely addressed by a product manager.
I tried to gather all the different position descriptions I could see on the market, and this is my overview.
So you have a platform product manager, someone like me, who’s responsible for the central stack. You also have platform product owners. The way we do it at Merck is there’s normally someone who’s responsible for particular technologies within the larger stack.
You could have someone who’s called a data product manager, an AI product manager, or an AI use case owner.
You also start seeing a product manager for external data platforms. This is very new and very special because it's about situations where several companies are interested in sharing the data together because they have a particular use case that brings value, and you need several parties to work together in a particular stack.
It's very complicated. I don't know any examples where such external platforms are already profitable. But I do believe that there’s definitely a future for this topic.
And of course, you might have someone who’s a technical project manager or AI project manager, but in reality, these people would potentially also have a product manager, depending on the company culture, what kind of product cultures they have, and so on.
There is a little bit of structure behind it. I come from Germany, and we really love to put structure into everything.
Every building block of the analytics journey needs product thinking
If you look at the analytics value chain, you start with raw data, and you process this data so that data scientists can work with it later. It's a very annoying process. No one likes it. But everyone needs it in order to build use cases later.
You build your first dashboards or reports. Most of you, when you work on your KPIs for product management, look at some reports and try to understand what the trend is. Where is it going?
Then you train particular machine learning models. And if it's something where you see there’s value, you put this use case into production.
Behind that, you have a platform, and as discussed before, you could also have external data platforms.
And for each of these elements, you need to have a product manager.
If I talk about the German and Swiss markets, it's only in the last two or three years that you can see companies like Merck or Volkswagen, so more traditional companies rather than tech companies, starting to publish roles that need product managers for their platform or a product manager for a particular high-value use case.
And it was only a couple of months ago that I saw the first position from Munich Re, an insurance company in Munich, that published a product manager for data for the first time.
What does it mean?
It means when you have your data, you can’t start working with it straight away. So you prepare it in accordance with all the product management practices. So this data set can be used either by data advanced business users or by data scientists to build a nice use case later.
And what's happened since is that a lot of companies have started to do AI without approaching all the steps in this analytics value chain.
According to Harvard Business Review, if you properly prepare data and treat that data like a product, you can reduce the time it takes to implement it in new use cases by as much as 90%. So it’s much easier and much faster.
But we don't like to touch it. We only want to make fancy AI and generative AI with ChatGPT, and then whatever comes next.
As I mentioned before, you actually need to build on it. You start with derived data, you understand data as a product, you build a nice dashboard, and you identify opportunities for machine learning, deep learning, or whatever you work with. You put it into production, and you might even think about building an external solution later as a large corporation.
Building an analytics solution for an external market
If I work at Merck, I have a fancy use case, and I think it’d be a great product for an external market, what do I do?
I have examples from the press.
Siemens is a huge German corporate company. They have software called MindSphere, which is focused on industry 4.0.
Volkswagen also launched its own software company. In the past, it was called Car.Software Org. They’ve now rebranded it to CARIAD.
Merck also has two joint ventures together with Palantir, in the areas of semiconductors and pharmaceuticals.
Also in the automotive area, there’s an initiative called Catena-X, which is basically sharing data between the value chain actors. Whereas with BMW, you don't build the car by yourself, you have your suppliers. If you want to change your process, you also need to change the processes of your supplier. So the idea is to have a central pool of data.
The organizational set-up of an AI product company
There’s a lot happening in the market because a lot of companies want to enter the software business and understand that this is the future. But if you look at the organizational set-up of a classic AI start-up, a company that sells some kind of AI software, if you're in the early stage, obviously, you'll have a person that’ll do 10 buckets from this list:
If it's 50-70 people, you’ll probably try to have one person per bucket, and then it grows further and further.
So if I talk now about Merck, and I take the sales team, do I have people at Merck who understand how to use the software? They understand how to sell the medicine or the drugs, but software is very different. If I have a fancy app, what do I do? How do I get these 50 people in my team to launch this product externally?
I have an example, which is the Lewis app at Merck. I really like this app. There’s a recent technology called optical character recognition (OCR), and you use it for text recognition. For example, if you’ve ever dealt with automated invoicing processing, you’d use it there because you need to recognize the text so that you can structure it later.
And then, within OCR, there’s a very small domain called optical chemical structures recognition. Within these algorithms, you can basically process chemical formulas in a machine-readable format.
It's a very nice idea because if you're a chemist who works on a new drug or you want to develop some new technical structure, you’ll need it because you want to look for examples in the library of available formulas, or you’d like algorithms to suggest components for your chemical structure.
It's a very nice app, and when I saw that our global data science director built it, I said, “Phillip, let's sell it. I believe in it, and I know how to sell it.”
But if you come back to the organizational set-up, how would Philip and I build up this team? This is one of the reasons why a lot of corporate companies fail when they launch their first external software product. They don't have this knowledge. They try to use their existing employees and change their focus to work with software. But it doesn't work like that.
I do believe that, in this case, we need to start with proper product management. And even at Merck or Volkswagen, there are a lot of teams who are now thinking about building external software products.
In my organization, I'm constantly repeating, “Start with proper product management. Start building a true product management capability, and they’ll be the driving force.”
And step by step, you build up other capabilities in order to be successful in the software market. But it's very tough, and it's a very long journey.
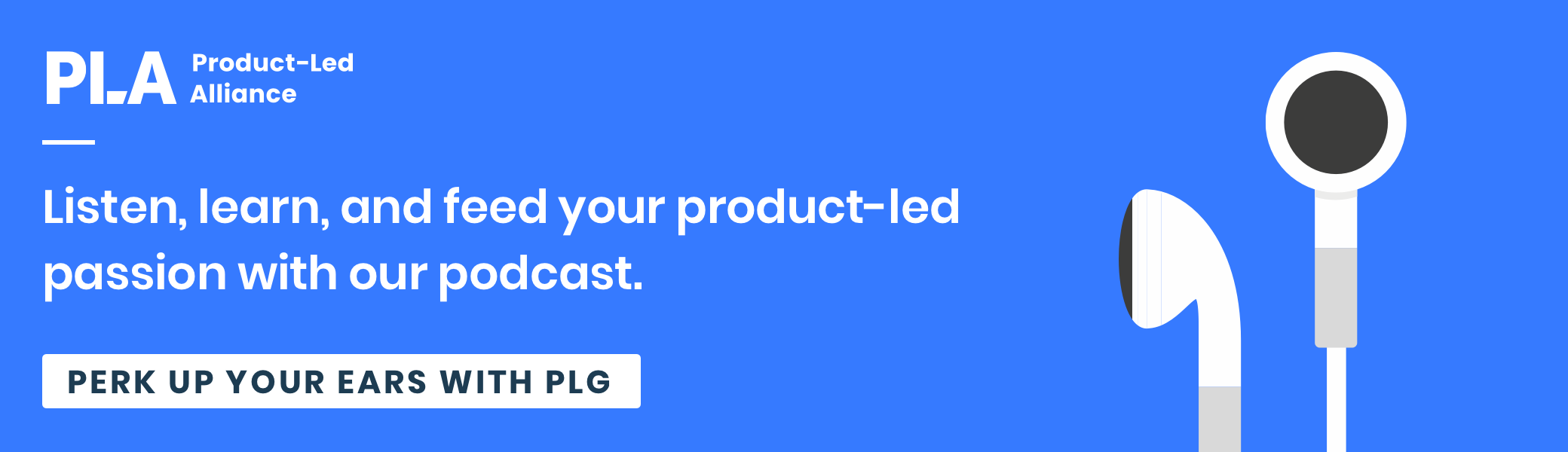