AI is everywhere. It’s the biggest buzzword in tech, drawing massive attention and hype. But here’s the uncomfortable truth – most people talking about AI don’t really understand it.
For many teams, AI has become the default answer, a shiny tool that promises innovation. Yet, the real challenge isn’t just about using AI but about using it well.
Are we solving meaningful problems? Are we enhancing customer experiences in ways that matter?
Every company is racing to showcase AI-powered features. But before you join the race, it’s worth asking: Should you even be using AI? Do you fully understand what it is, how it works, and when it makes sense to apply it?
Now is the perfect time to pause and reflect.
AI isn’t just another trend – it’s a transformational shift in technology, moving faster than anything we’ve seen before. The teams who take the time to understand it deeply will shape the next generation of products, and those who don’t may struggle to keep up.
1. Don't start with AI, start with the problem
One of the biggest mistakes PMs make is deciding to "use AI" first and then scrambling to find a problem for it. This is the wrong approach.
Early in my career, I made a classic mistake – one that many product managers still make today.
I got excited about building with AI first and only later started looking for a problem to solve.
I dove into creating AI-powered features that looked impressive on paper, but when they reached real users, the excitement didn’t translate. The core customer pain-points remained unaddressed, and the AI capabilities, however advanced, went largely unnoticed.
That experience stuck with me.
It taught me that the real power of AI (or any technology) isn’t in how sophisticated it looks, but in whether it solves the right problem.
These days, I don’t start with “How can we use AI?” – I start with “What is the real customer problem?”
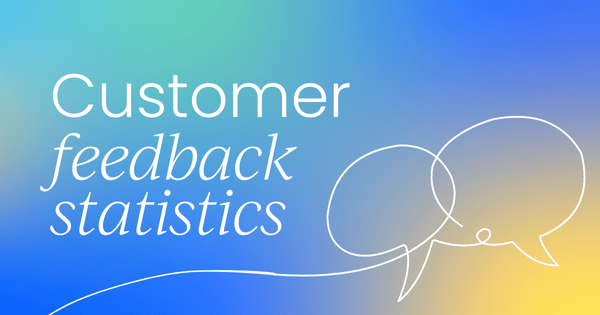
Why is starting with AI such a mistake?
- Misaligned resources: You end up forcing a solution that may not address actual customer needs
- Wasted investment: Building AI capabilities is expensive and time-consuming
- Feature bloat: You’ll add complexity without adding value
- Missed opportunities: While focusing on shiny AI features, you might overlook simpler solutions that could have a greater impact
Instead:
- Define the right problem – This is where PMs create the most value. If you can do this well, you'll be in high demand.
- Understand your users deeply – No AI can replace product intuition and customer empathy.
- Own core product skills – AI won't rescue a poorly defined strategy.
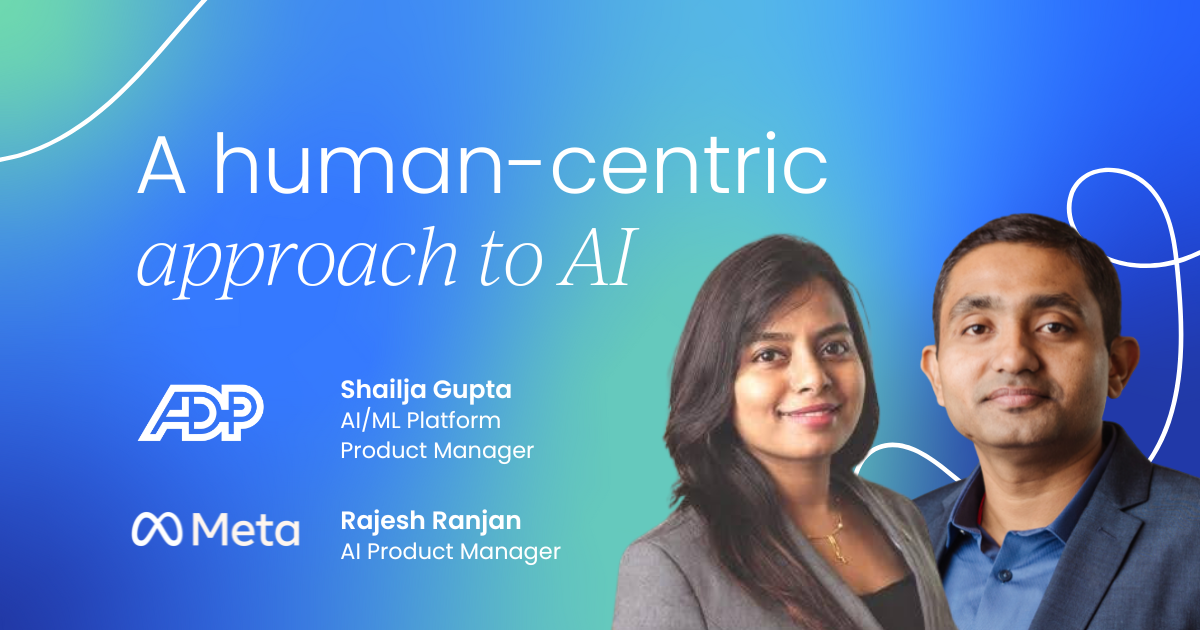
2. AI is a tool: Use it only when it makes sense
AI is not a magic wand. It’s only useful if it provides a real advantage.
Ask yourself:
- Does AI actually improve the user experience?
- Would a simpler solution be more efficient?
- Do we have the right data to train and optimize AI models?
Not everything needs AI. The best PMs are strategic thinkers, not AI hype chasers.
Good AI use case: eCommerce product recommendations
Imagine an eCommerce platform with hundreds of millions of products – from fashion to electronics to home decor. A static, one-size-fits-all product listing wouldn’t work.
This is where AI truly makes sense – by using machine learning models to analyze:
- User behavior and browsing history
- Purchase patterns and preferences
- Similar user data to predict relevant products
For example, when shopping online on a site with millions of items, customers often feel overwhelmed by choices. A well-implemented recommendation engine can dramatically increase conversion rates because it solves a genuine user pain point.
Bad AI use case: AI-powered customer assistant voice bots
On the flip side, AI can completely undermine the customer support experience when applied without proper oversight.
We've all been caught in the frustrating cycle: you're trying to resolve an urgent issue, but an AI voice bot keeps you trapped in an endless loop of irrelevant options and scripted responses.
I've personally abandoned hotel reservations and switched mobile carriers after hitting a wall with these systems. In today's market, customer patience is a finite resource, and poor service experiences drive customers straight to competitors.
What goes wrong?
- Misinterpreted intent: The AI fails to understand the nuance or context of customer questions, offering generic solutions to specific problems.
- Emotional tone-deafness: These systems lack the emotional intelligence to recognize and respond appropriately to customer frustration, often exacerbating negative experiences.
- Inflexible decision trees: Bots frequently lack the judgment to know when a problem exceeds their capabilities and when to route customers to human associates.
- Cost-cutting motivation: Many implementations prioritize reducing support costs over improving customer experience, resulting in systems optimized for containment rather than resolution.
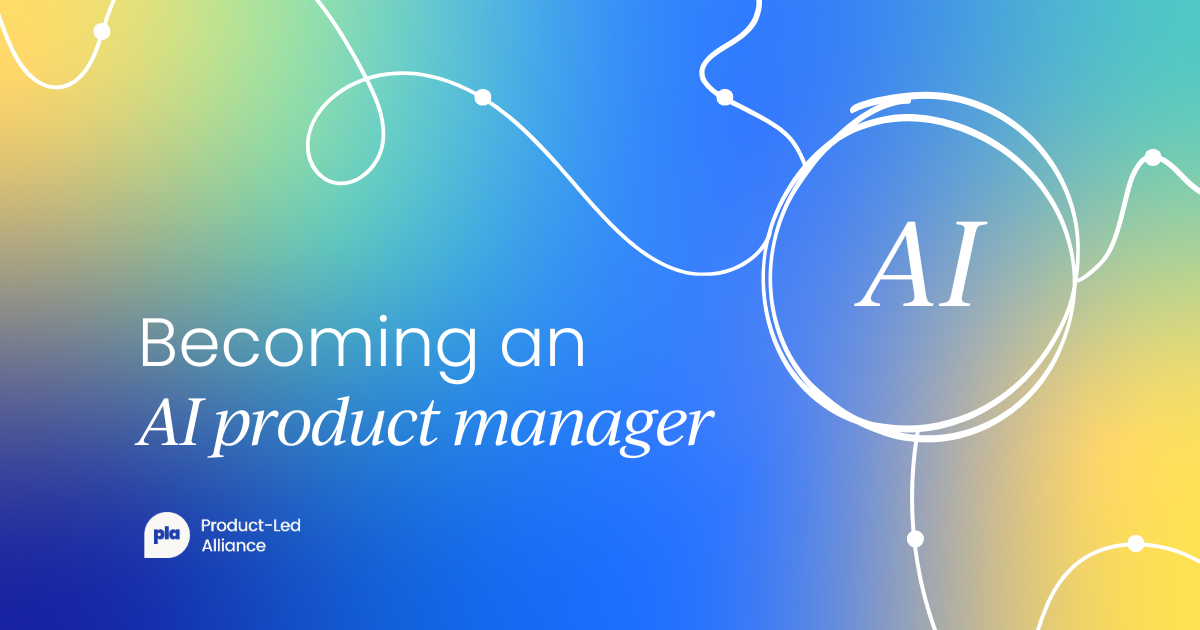
3. Common AI myths that can mislead PMs
“We need to use AI to stay competitive” – Wrong. You need to solve the right problems to stay competitive. AI is a means, not the goal.
“AI will replace PMs” – Nope. AI can automate tasks, but it can’t define strategy, prioritize problems, or understand human behavior like a great PM can.
“AI works like magic” – Far from it. AI is only as good as the data it’s trained on. Bad data leads to bad AI.
“You need to be an AI expert” – False. You don’t need a PhD, but you do need AI literacy. Learn the basics, collaborate with your DS team, and get hands-on with AI tools.
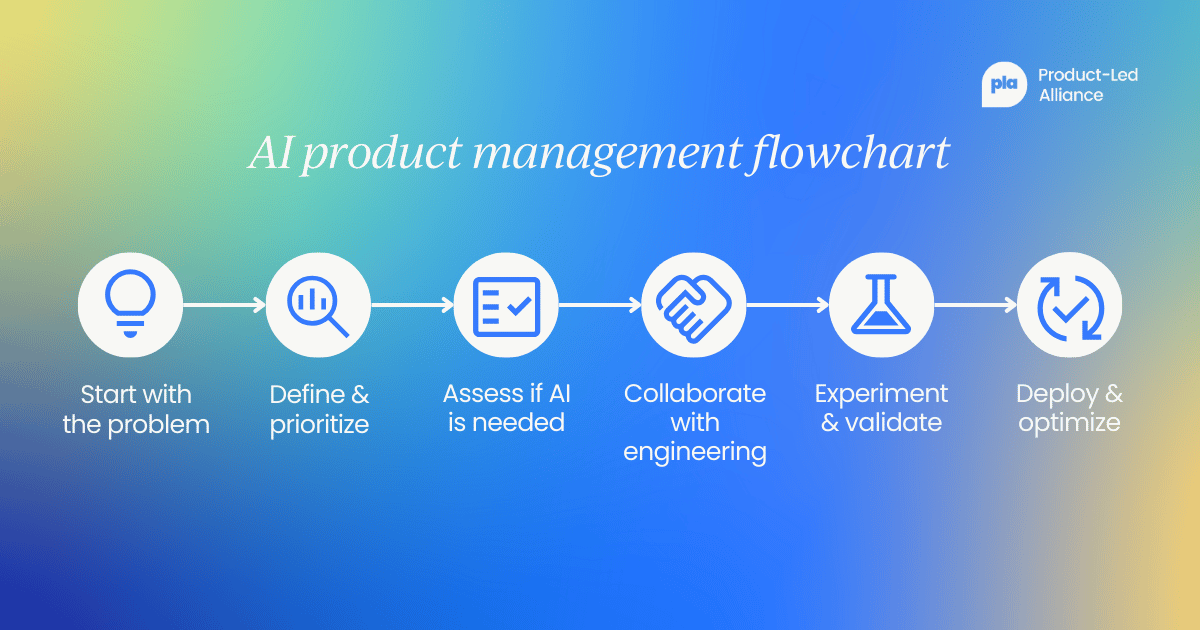
4. Collaborate with your engineering and data science partners
Once you determine AI is viable, work closely with:
- Data science teams to explore ML models and feasibility.
- Data engineers to identify the right data sources.
- Engineering teams to ensure scalable and efficient integration.
AI PMs who can challenge assumptions and structure ideas will drive the best solutions.
In my experience leading AI initiatives across multiple e-commerce platforms, these best practices made all the difference:
1. Create a shared knowledge base
Develop a central repository (like a wiki or shared document) outlining business objectives, technical constraints, and user needs that all teams can reference.
2. Establish clear decision frameworks
Agree on prioritization criteria upfront. Determine which factors (accuracy, speed, cost, etc.) take precedence and establish shared north star metrics that align all teams.
3. Set up regular knowledge-sharing sessions
Have technical teams provide AI/ML fundamentals workshops for business stakeholders, while product and design teams offer customer journey immersion sessions for technical teams.
4. Develop common vocabulary
Create a glossary of terms to ensure engineers, data scientists, product managers, and designers are using consistent language when discussing AI capabilities and limitations.
5. Use prototypes as communication tools
Instead of lengthy requirements documents, build simple prototypes to demonstrate how AI will integrate into the customer experience, making abstract concepts tangible for all stakeholders.
This approach helps bridge the knowledge gap between teams.
Engineers gain deeper understanding of customer problems, while product and business teams develop better intuition about technical limitations and possibilities.
The result is a more effective AI implementation that truly delivers on business and customer needs.
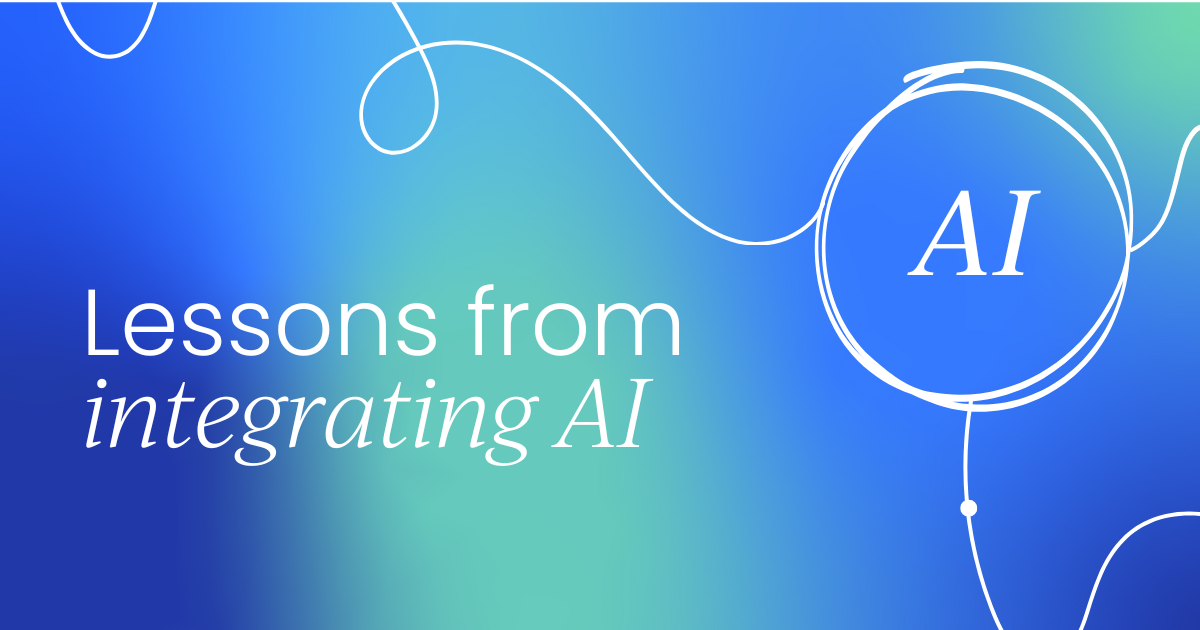
5. Build AI responsibly: Don’t break user trust
AI isn’t just about innovation; it’s also about responsibility. As a product manager, you play a key role in ensuring AI enhances user experience without compromising trust. This means paying close attention to:
- Privacy & Security – Protect customer data rigorously.
- Ethical AI – Strive for bias-free, fair, and explainable models.
- Transparency – Help users clearly understand how AI-driven decisions affect them.
Giving customers control over their data, for example, by providing simple tools to manage what information is collected and how it’s used, can significantly boost trust and credibility.
When done right, responsible AI not only drives engagement but also fosters long-term customer loyalty.
6. Measure if it’s working: The AI feedback loop
AI models are not static. They need to evolve based on real-world data and feedback. To measure success:
- Define clear success metrics: Not just accuracy, but actual business impact.
- A/B test rigorously: AI behavior often differs in production vs. testing.
- Continuously improve: AI should adapt and get better over time.
Without measurement, AI is just a black box. Make sure you can validate its impact.
Ask the hard questions:
- Is this AI feature actually improving core metrics?
- Are users getting measurable value, or just novelty?
- What's the ROI compared to simpler solutions?
- Are there unintended consequences we need to address?
Don't fall into the trap of assuming AI features are valuable just because they're AI. And most importantly, listen to your users, not the hype.
Your most valuable feedback won't come from tech blogs or competitors - it'll come directly from your users.
Create tight feedback loops by:
- Watching how your users actually use (or don't use) your AI features
- Asking customers directly what's working and what isn't
- Tracking which AI recommendations users follow vs. ignore
- Identifying when customers are frustrated or confused
Then do something with that feedback. AI systems need constant refinement based on real-world use.
Final thoughts
AI is becoming foundational to product development. New talent is entering the field with AI skills from day one.
If you don’t level up, you risk being left behind.
AI isn't going to save your product if you don't understand your users. It won't magically fix a broken value proposition. And it definitely won't be a substitute for good product fundamentals.
But used thoughtfully, it can make good products dramatically better. It can solve problems that were previously unsolvable. And it can create experiences that feel magical to your users.
The best PMs of tomorrow won’t just use AI. They’ll master its application, strategy, and responsible deployment.
Are you ready to be one of them? It starts by asking the right questions.
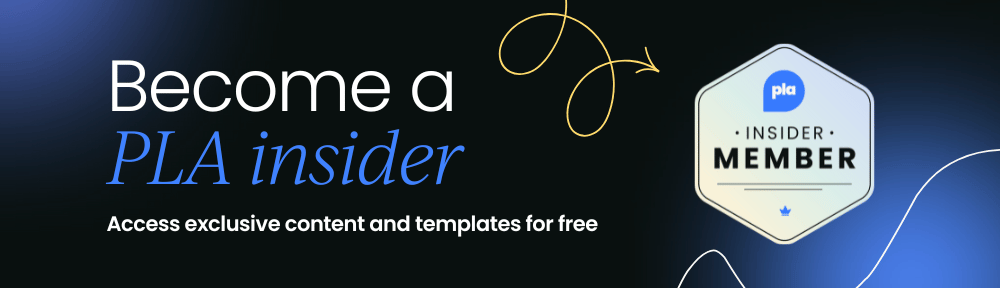