In the ever-evolving landscape of artificial intelligence, product managers (PMs) play a pivotal role in driving innovation and ensuring AI-powered products meet customer needs and market demands. However, as we continue on in 2023, AI product managers face a set of unique challenges that demand creativity, adaptability, and a deep understanding of both AI technology and user behavior.
So what kind of key hurdles are AI PMs likely to encounter this year?
1. Data privacy and ethical concerns
AI product managers must grapple with heightened concerns about data privacy and ethics in AI applications. As regulations like GDPR and CCPA evolve and public awareness of data misuse grows, navigating the ethical and legal aspects of AI becomes increasingly complex. Product managers need to ensure that their AI systems are not only technically robust but also respect user privacy and adhere to ethical guidelines.
2. Data bias and fairness
Bias in AI algorithms has been a persistent issue, and it's not going away. AI product managers must actively work to identify and mitigate bias in their AI models to ensure fairness and equity. Achieving a balance between algorithmic accuracy and fairness is a formidable challenge that requires constant vigilance and fine-tuning.
3. Talent shortage
The demand for AI talent continues to outstrip the supply, making it challenging to recruit and retain skilled professionals. AI product managers must navigate a competitive job market and seek innovative ways to build and lead diverse, high-performing teams.
4. Rapid technological advancements
AI is evolving at breakneck speed, with new techniques, models, and technologies emerging almost daily. Keeping up with these advancements is a significant challenge for AI product managers, as they need to evaluate which new tools and methodologies are worth integrating into their products to maintain a competitive edge.
5. User expectations and trust
Users have high expectations for AI-powered products, and building trust in AI systems is a long-term challenge. Product managers must not only meet user expectations but also ensure that AI systems are transparent, reliable, and user-friendly to foster trust among consumers.
6. Interdisciplinary collaboration
AI product managers must work closely with cross-functional teams, including engineers, data scientists, UX designers, and legal experts. Effective communication and collaboration across these disciplines are crucial for success but can be challenging due to varying expertise and priorities.
7. Scalability and cost management
Scaling AI products can be expensive and challenging. AI product managers must balance innovation with cost-effectiveness, seeking ways to deploy AI solutions efficiently and ensure they remain financially sustainable in the long run.
8. Security Threats:
As AI systems become more integrated into critical infrastructure and services, they become attractive targets for cyberattacks. AI product managers must prioritize security measures to protect against data breaches, algorithm manipulation, and other security threats.
AI product managers in 2023 face a complex and dynamic landscape characterized by ethical, technical, and organizational challenges. To thrive in this environment, they must embrace a mindset of continuous learning, stay abreast of regulatory changes, prioritize fairness and ethics, and foster interdisciplinary collaboration.
Building trust in AI
As an AI product manager, building trust in AI systems is paramount. Trust is the foundation upon which users and stakeholders will rely on your products. To achieve this, transparency is key. Be open about how your AI algorithms work, what data is used, and how decisions are made. Strive for fairness by constantly monitoring and addressing bias in your models. Consistency and reliability are also vital; ensure that your AI systems consistently deliver accurate results. User education is another essential component – help users understand the capabilities and limitations of your AI products.
Finally, robust data privacy and security measures are non-negotiable. By demonstrating transparency, fairness, reliability, and a commitment to safeguarding user data, you can establish the trust necessary for the success and adoption of AI products.
Ever-increasing expectations
The role of product managers is largely fluid as it is. And as expectations are always increasing, it’s essential that product managers continue to develop a fundamental understanding of how to correctly leverage AI and ML.
As both expectations and regulations change over the coming years, uncertainty will undoubtedly still surround many AI initiatives. This is why having the ability to keep learning and making incremental improvements is critical going forward.
But challenges are meant to be conquered, all to get to a place where AI is used to really benefit consumers and not just the bottom line.
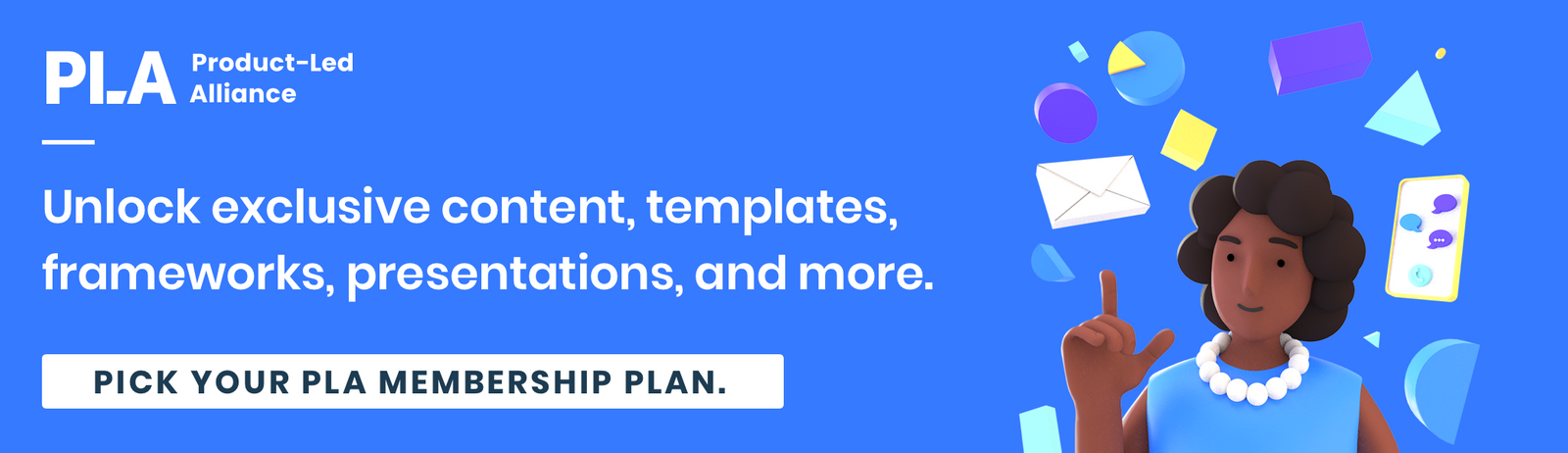