In this article, I’ll dive headfirst into the world of experimentation, the impact they can have on growth, and share key learnings taken from real-world examples of experiments at HubSpot.
I’ll discuss why running experiments is so important, our obsession with documentation, and how it can help with communication, the experiment process at HubSpot, and a step-by-step account of the process in action.
My name's David Ly Kim, I've been at HubSpot for four years going on five, I started off in marketing, now I'm in product.
In this article, I'm going to talk about growth strategy.
Imagine this...
Before I dive in, I want you to imagine this, you're looking at some chart, it might be traffic, leads you're generating, the number of free users you're getting to your product. Whatever it is, it's something that you want to be going up and to the right.
But it's looking flat and you're wondering, what's happening here? I need to do something, I need to change something or I'll either get fired or my company's not going to succeed.
You're thinking I read some blog posts that said I need to run some experiments. This is a Google search for growth experiments, where you'll get multiple blog posts of all the different experiments you should be running on your website and on your product.
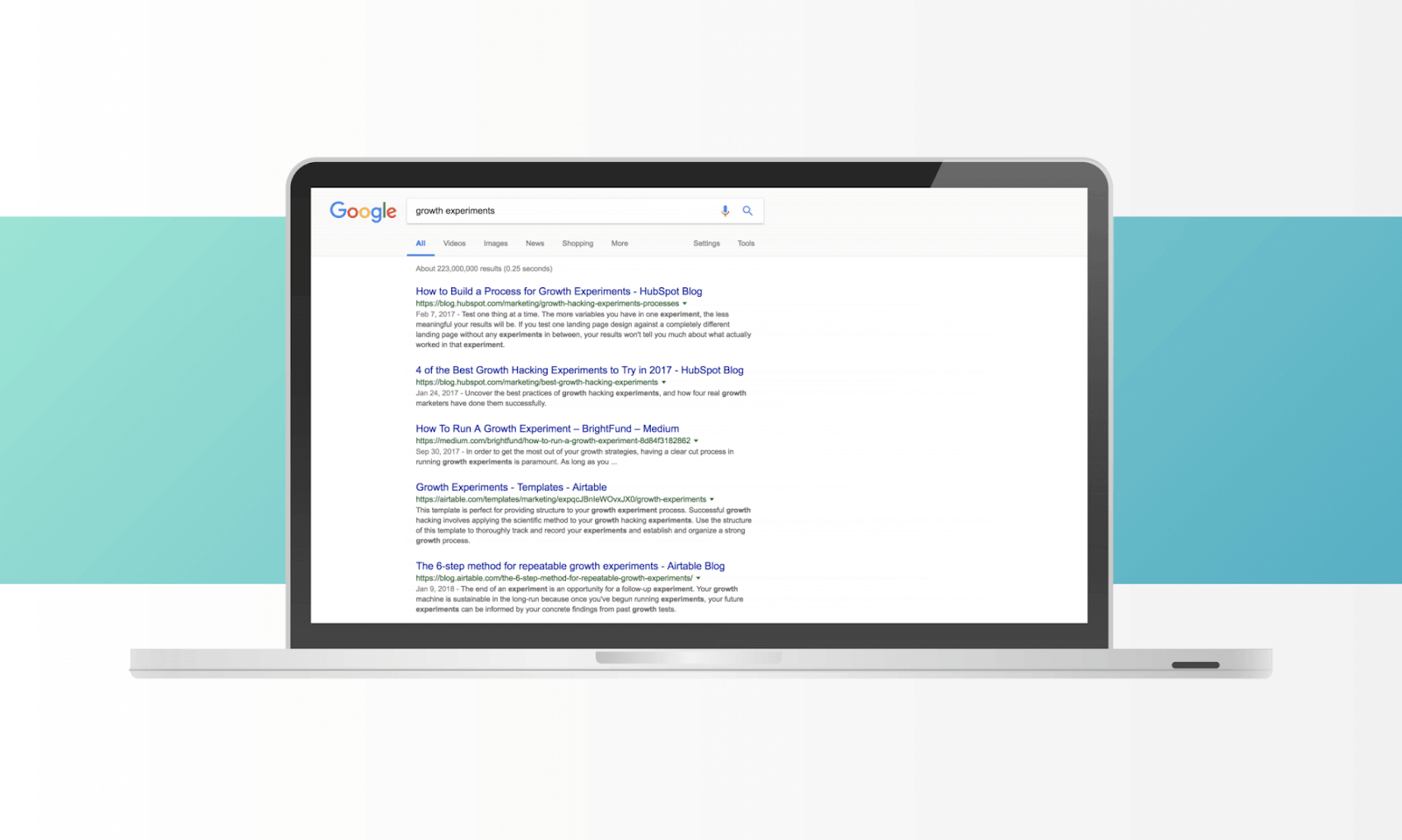
You're thinking, I've got to start somewhere and so you think well, there's this button on my website, I want people to sign up, what if I just made that red? You don't really know what to expect but you do know that you need to start testing some things, changing some things on your website, and you run it for a couple of weeks.
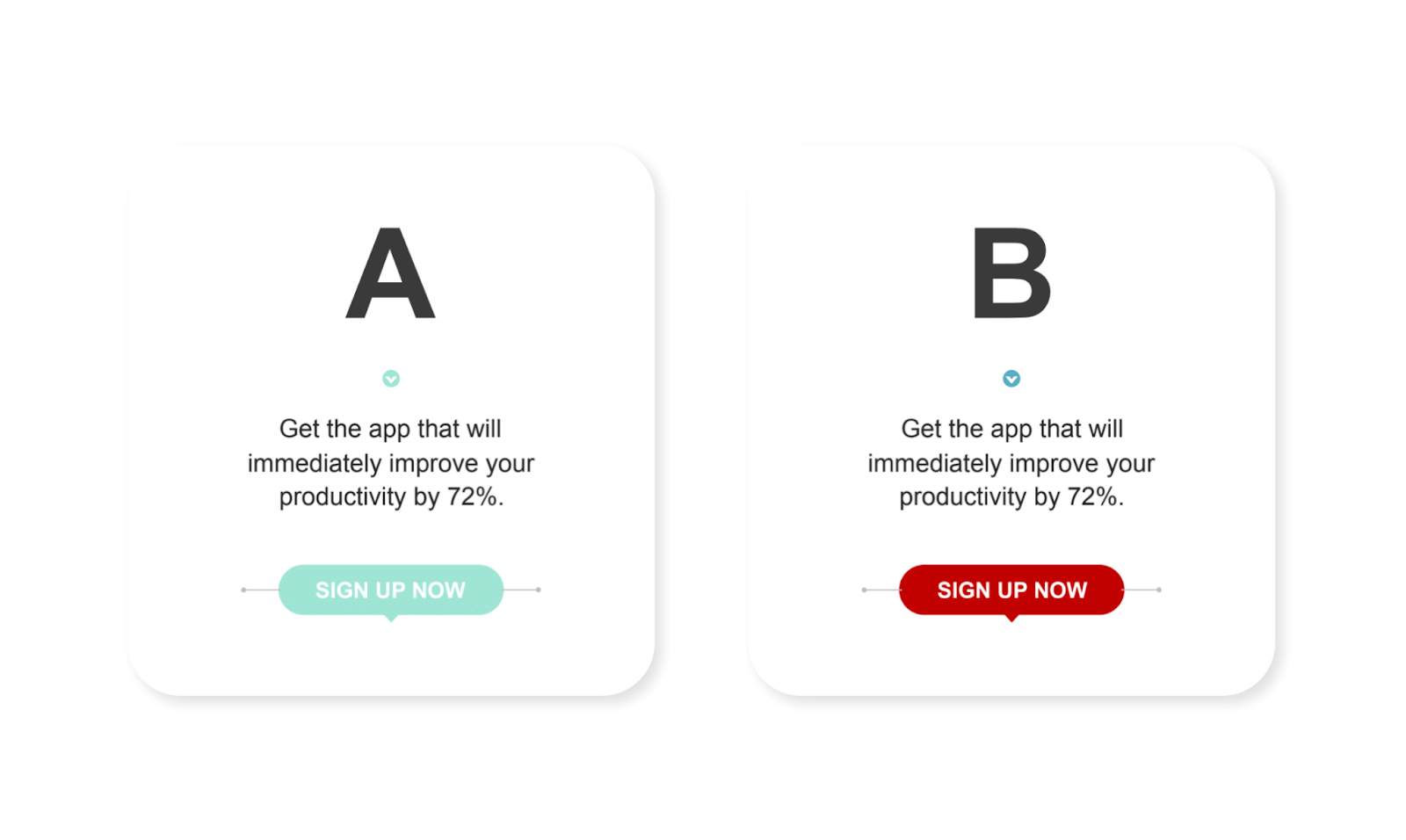
A couple of weeks later, your graph still looks like this…
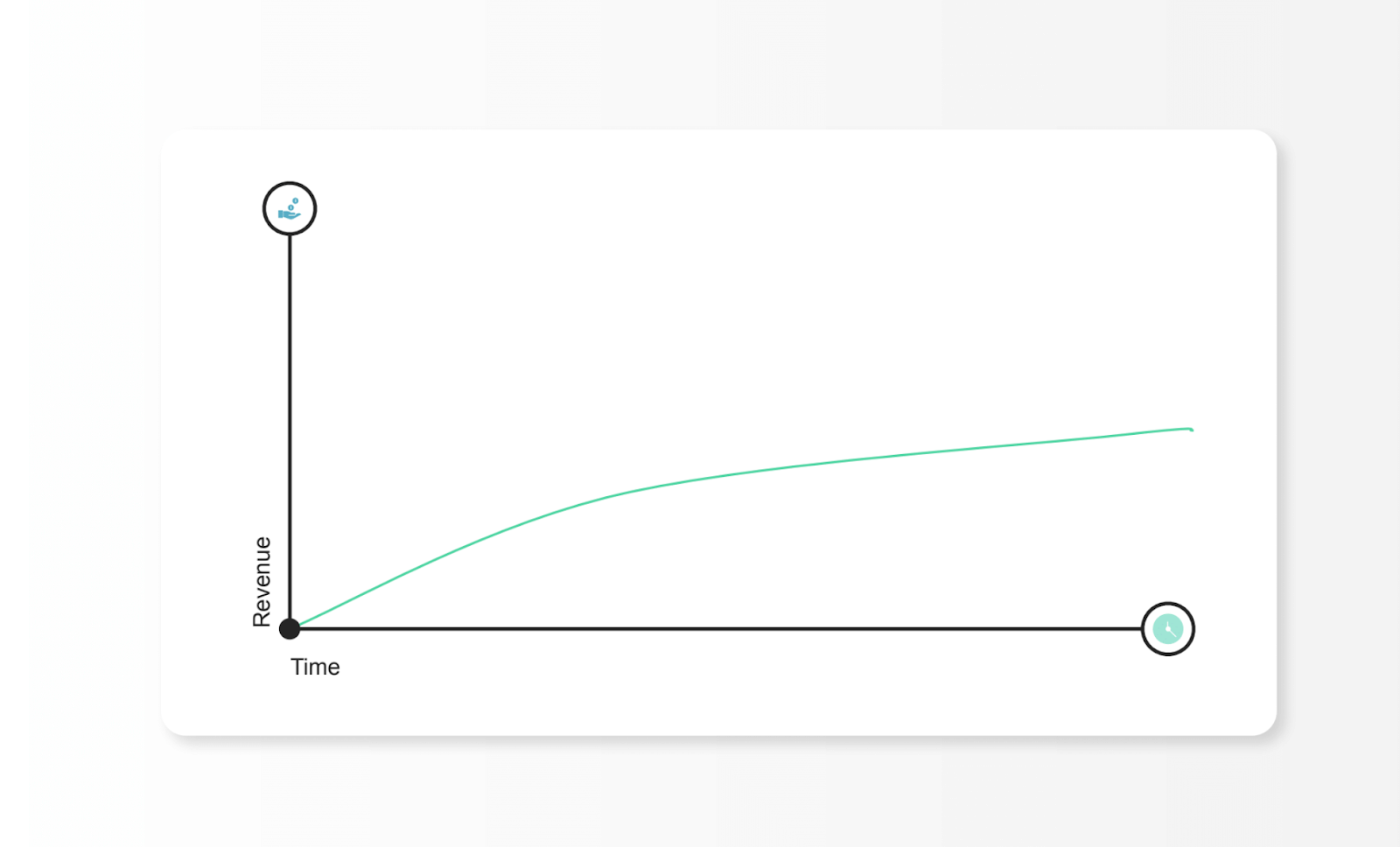
Something's going on. If this continues, it's not going to look very good.
You're thinking a lot of those blog posts say 90% of what I do is going to fail so I need to keep running experiments. You keep running experiments and still, nothing really changes, you're still getting that same chart week after week, and it's been a couple of months at this point, you start to wonder, maybe these experiments are the wrong experiments, maybe I'm running this wrong, maybe I need to ask someone else for help or hire a consultant.
Really you need to start asking what's happening here?
What’s happening?
The reason why this is generally what happens when people start getting into experimentation is people tend to focus on the tactics and solutions before really understanding what the problem is.
If you think about button color change, what are they trying to solve by changing that button color? It's because people probably aren't converting on the website so they're thinking maybe changing a button color would solve that.
But if you pull it all the way back to that problem of saying, people are not converting on a website, that opens up a bunch of different things you could be trying out to improve that conversion rate. We always need to start with problems.
Example problems
This is going to be extremely simple, but it's easy to forget about starting from here.
- You get a lot of traffic, but no one's actually signing up or becoming a lead.
- You might get people who sign up for your product, but they never end up using it.
- You might get people that will actually use your product, but they don't pay you.
- You might get leads that get on calls with you, but they don't pay you.
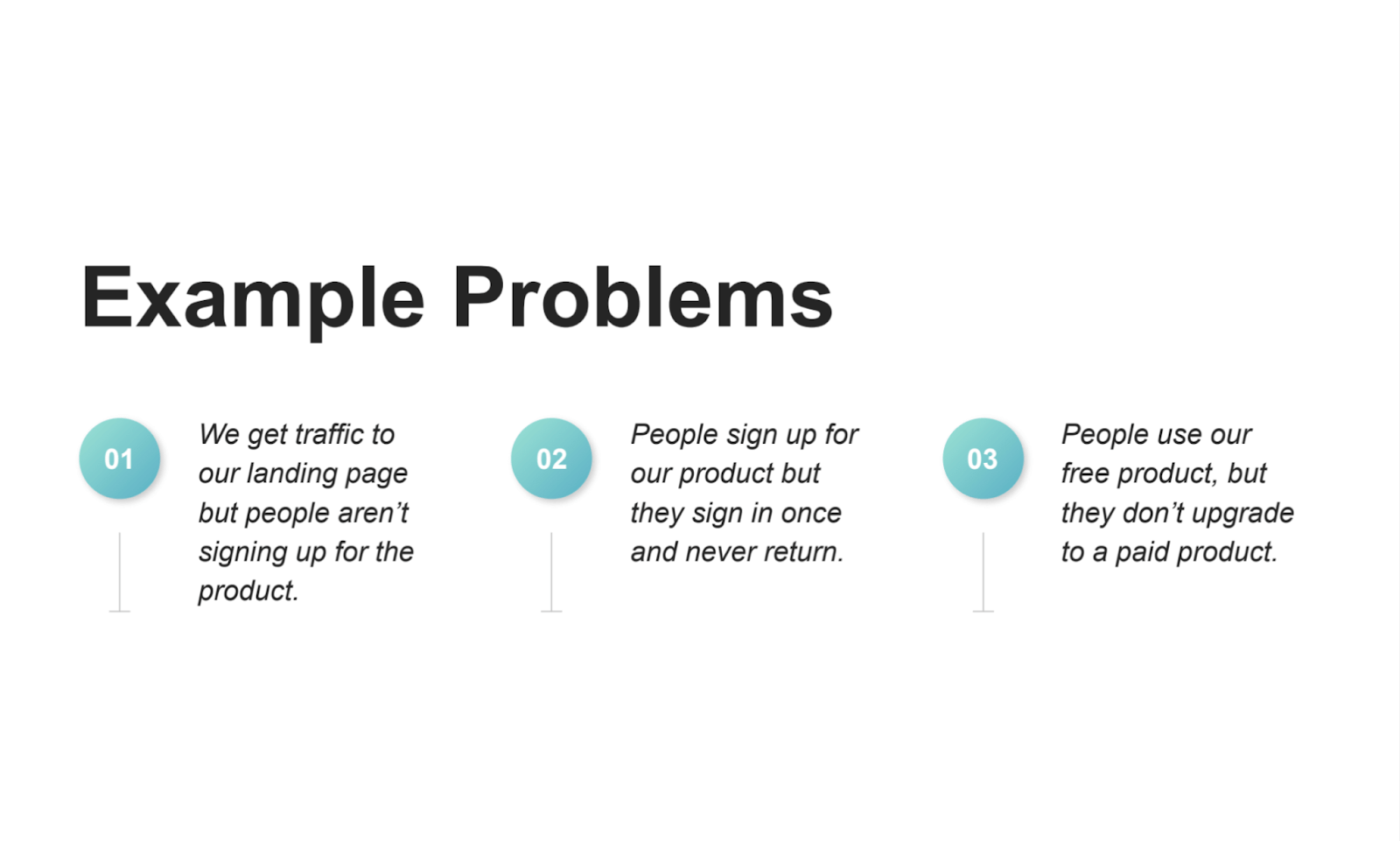
When you start with these problems, that opens up a world of different solutions that you can use to tackle these problems.
There’s a better way to grow
At HubSpot, one of the biggest changes we made was going from a heavily marketing sales business to a freemium business, where it required a lot of partnership between marketing and product.
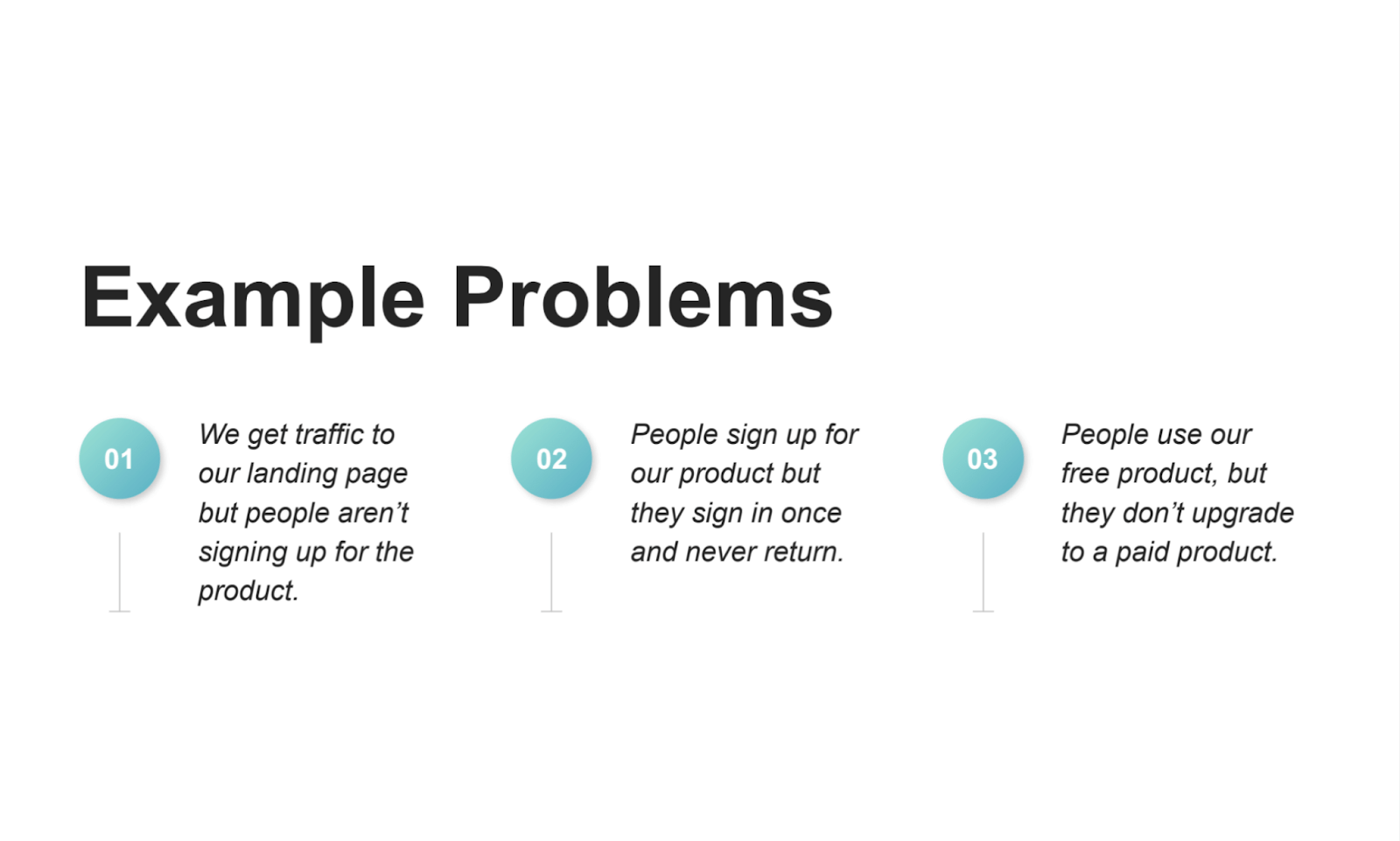
This article is going to make it seem easy. It was actually very difficult and it requires a lot of communication and a lot of people willing to collaborate, and I'll share a little bit about how we collaborated.
What I’ll cover in this article
In this article, I'll go through:
- Why run experiments?
- Our obsession over documentation and how that actually helps with communication,
- That process of experimentation. And,
- I'm going to talk about some real experiments that we ran.
Why we run experiments
Experiments are a tool that helps us understand how to move a metric. I specifically say it's a tool because it's one of many tools in our toolkit to determine how to make business decisions.
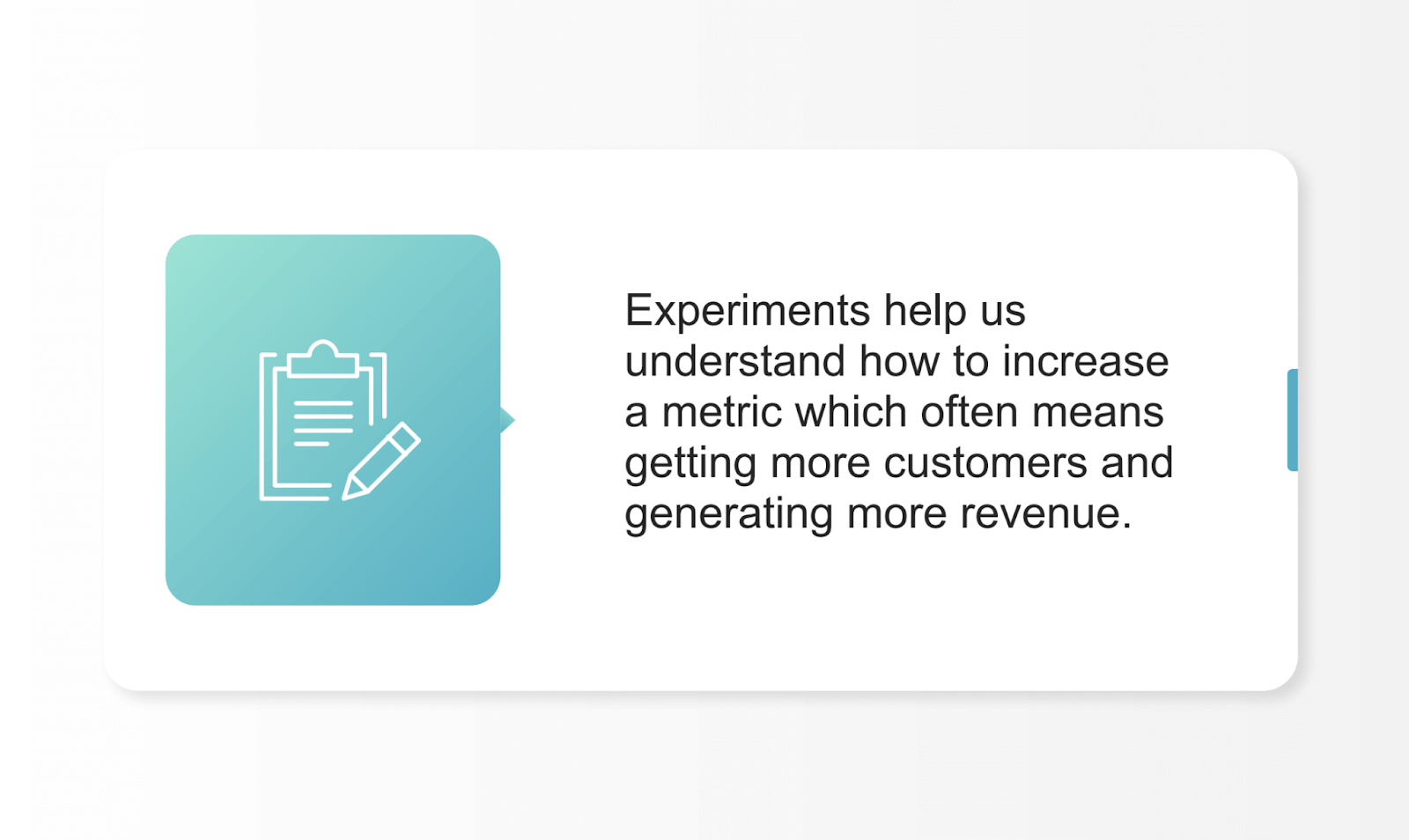
You might be looking at data and decide that you want to make a decision on that. You might say this data is not easy to trust because you're not sure what the source is or there might be seasonality so you might want to experiment to prove this is the right decision to make.
Think of experiments not as your only solution, but as one of many.
Anyone can do a tactic
The challenge here with growth tactics is anyone can do a tactic. If you think about any sort of tactic, they go through their life cycle where someone discovers it, everyone starts doing it, and it stops working.
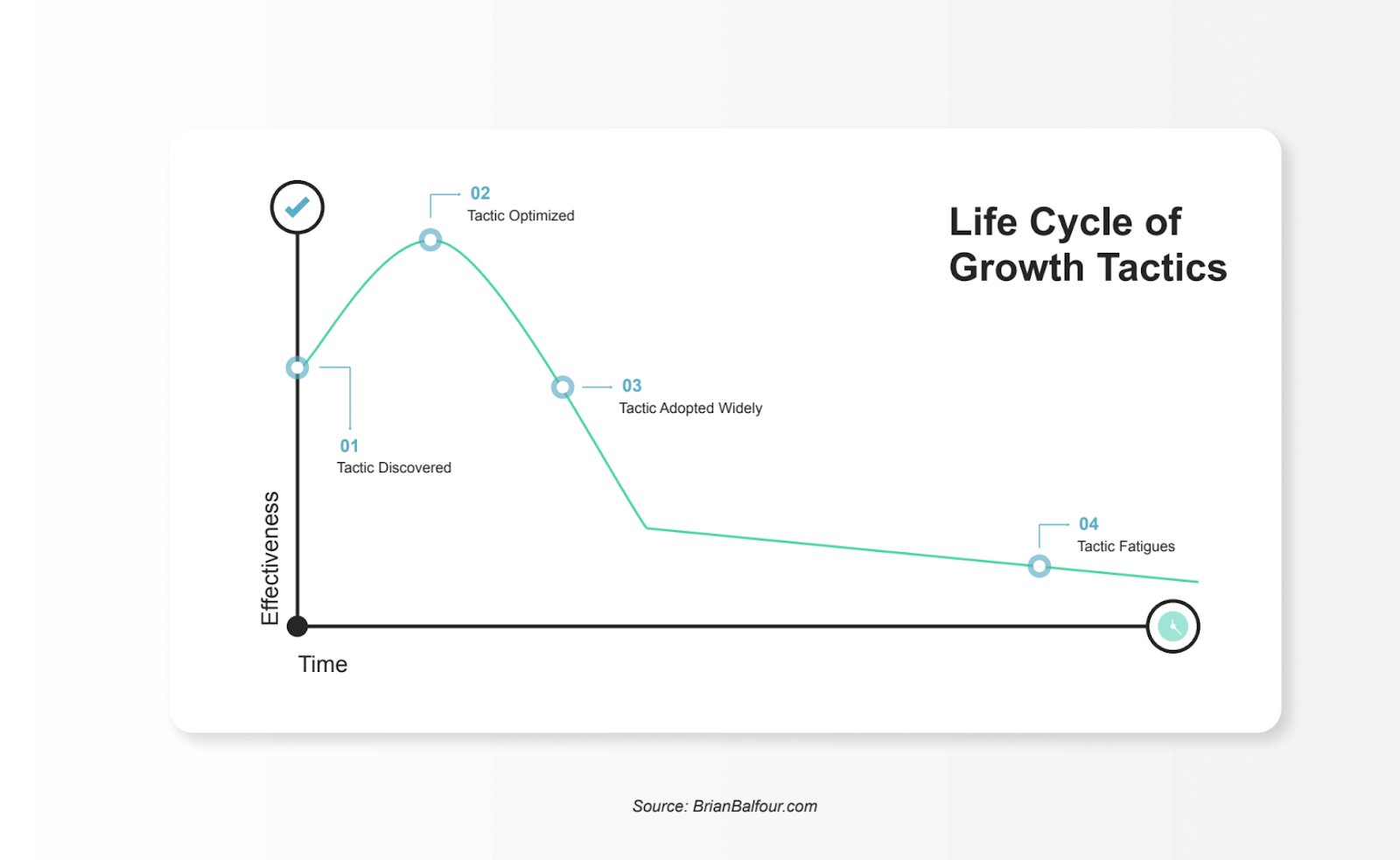
An example here is email marketing. Back in the early 2000s email marketing was probably getting 60-70% open rates but now you're getting huge email inboxes with 10,000 unread emails, no one really wants to open emails anymore, you get lucky if you get a 20% open rate.
If you think about pop-up forms, for instance, I don't know how long you've been in marketing but back when pop-up forms first started, they were hailed as having a 70% conversion rate. Now, I don't know about you, but the moment I see a pop-up appear I look for the X button immediately.
Some websites even resort to cheat tactics where they hide the X button and make it white on a white background. These are silly tactics that are not going to actually help you and provide a bad user experience.
How do you avoid this life cycle of growth tactics and instead, do things that help your users?
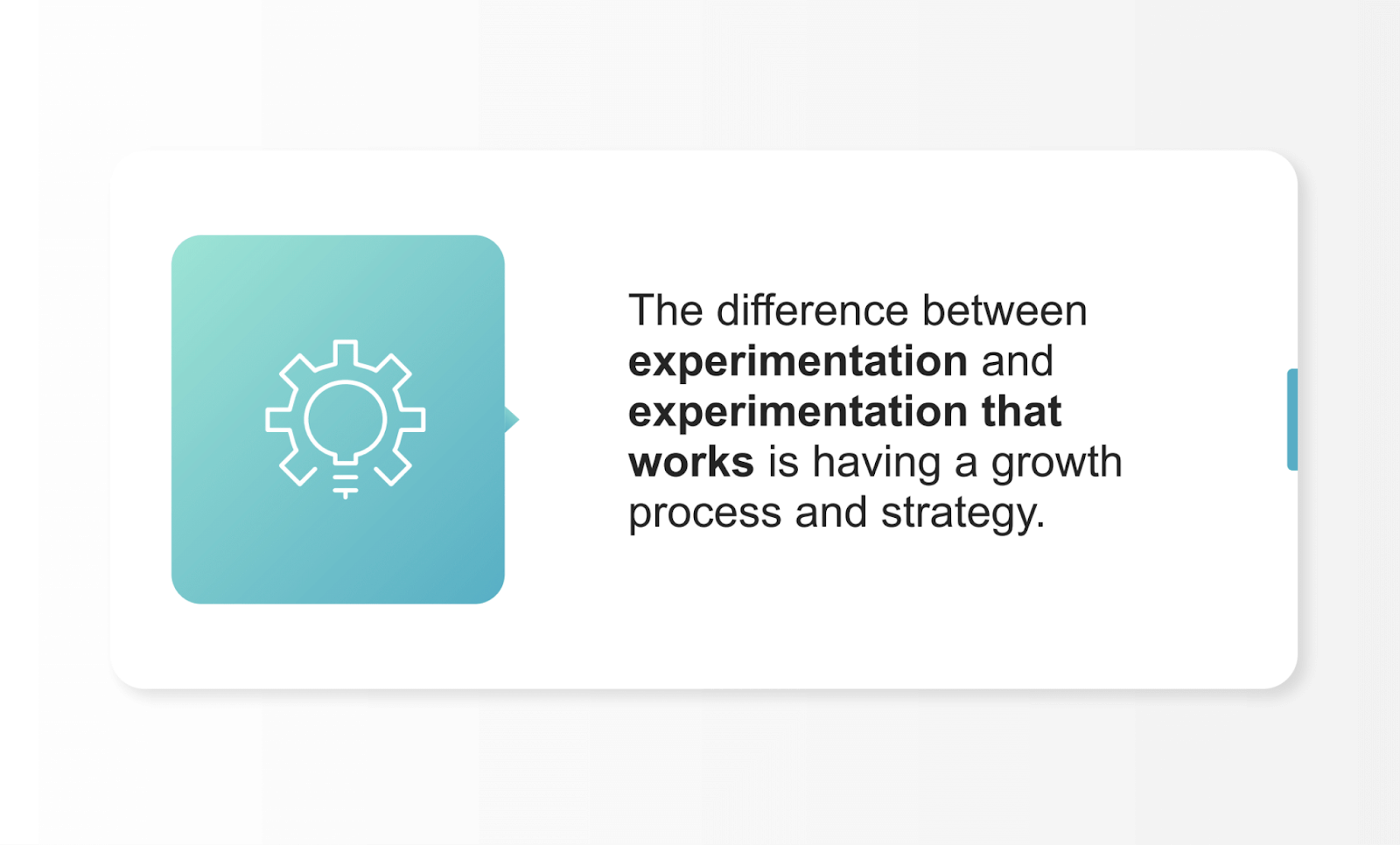
That's the difference between experimentation and experimentation that works, it's having a process and strategy for why you're doing those experiments in the first place.
Tal Raviv from Patreon said without the scientific method, we would just be growth hacking, we'd just be trying a bunch of random things from loud logs, but not truly understanding our users.
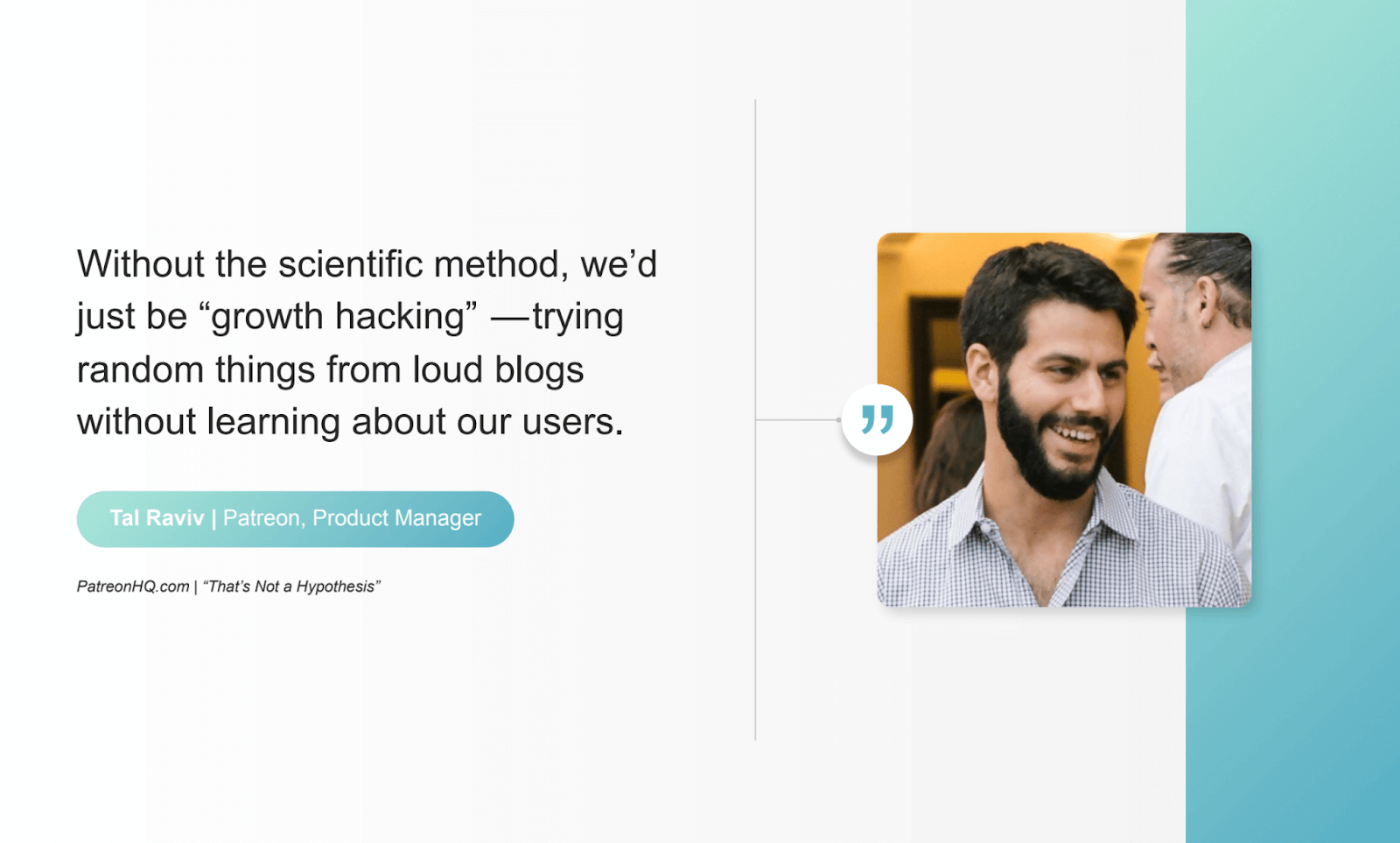
How do you avoid growth hacking?
Back to that first example of changing a button color, what makes us think our user is going to click a red button versus a green button? There's nothing that we're learning there.
Obsession over documentation
To avoid growth hacking, at HubSpot, we focus very strongly on documentation. That's how we create this really, really fine filter for high-quality experiments.
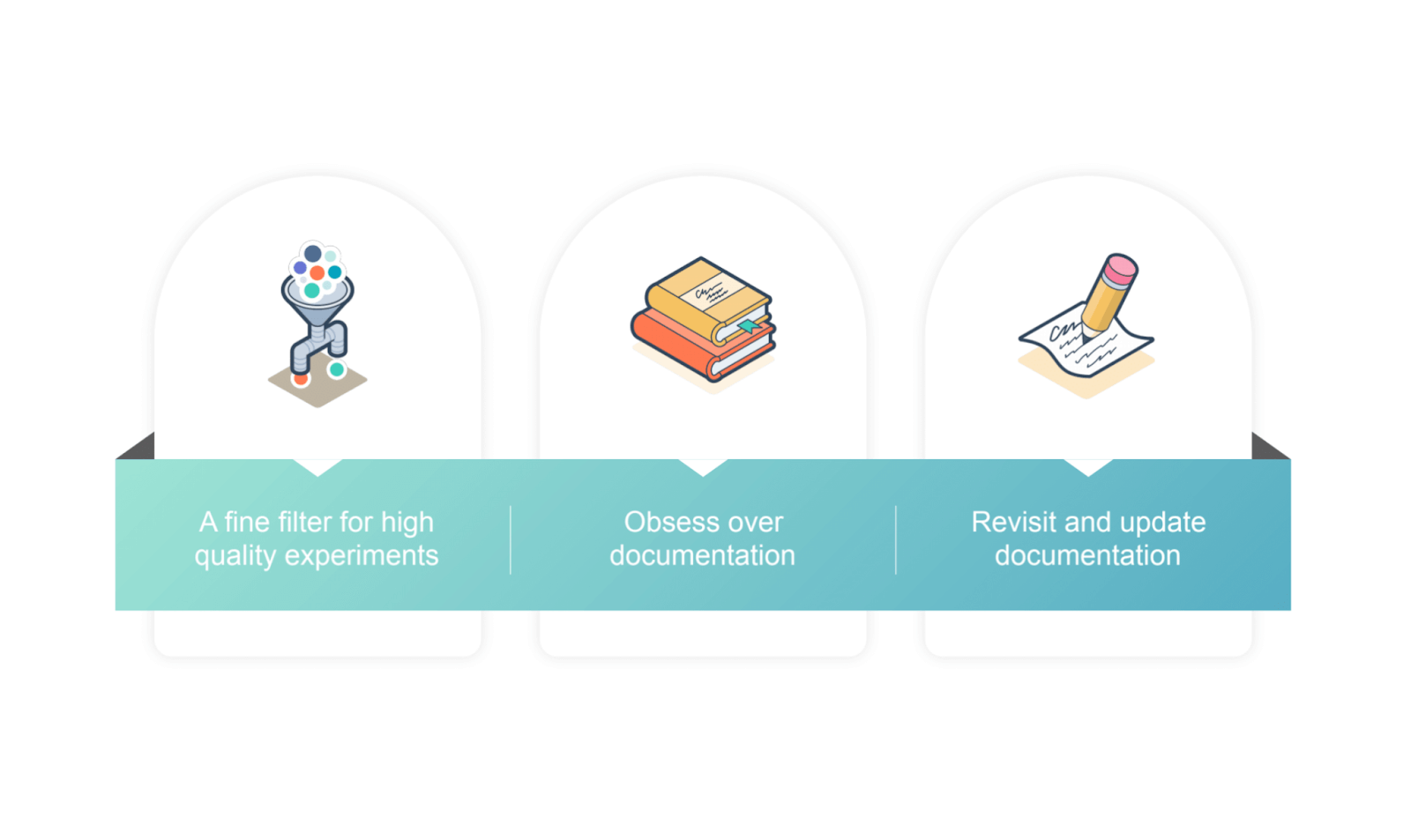
As you start running experiments, you're going to make mistakes, we made a ton, you're going to run a lot of things that don't work, you're going to have a few experiments that actually work. But there's going to be a certain point where you say, "Hey, we ran something like this six months ago, didn't work, what makes you think it's worth running this time?"
So you can continue filtering out things that might not work, and focus on things that could.
An example: Social proof in sign up flow
This is an example of documentation we've actually done at HubSpot, I'll walk through this experiment later in the article.
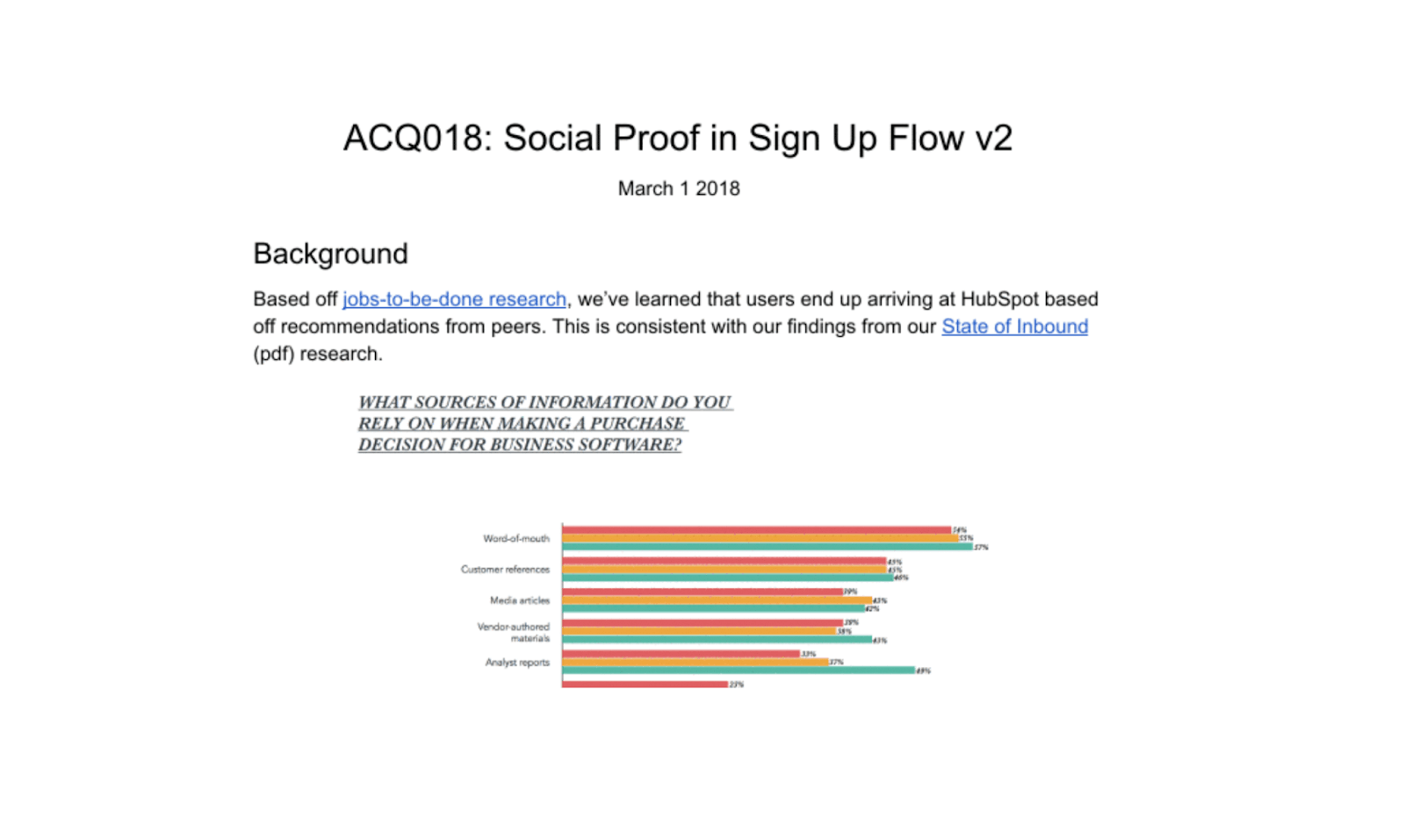
You can see it started off with a hypothesis, all the designs we did, it goes into the learnings and results and all the follow up we did as well.
Our experiment process
In a document, we go through every single one of these items.
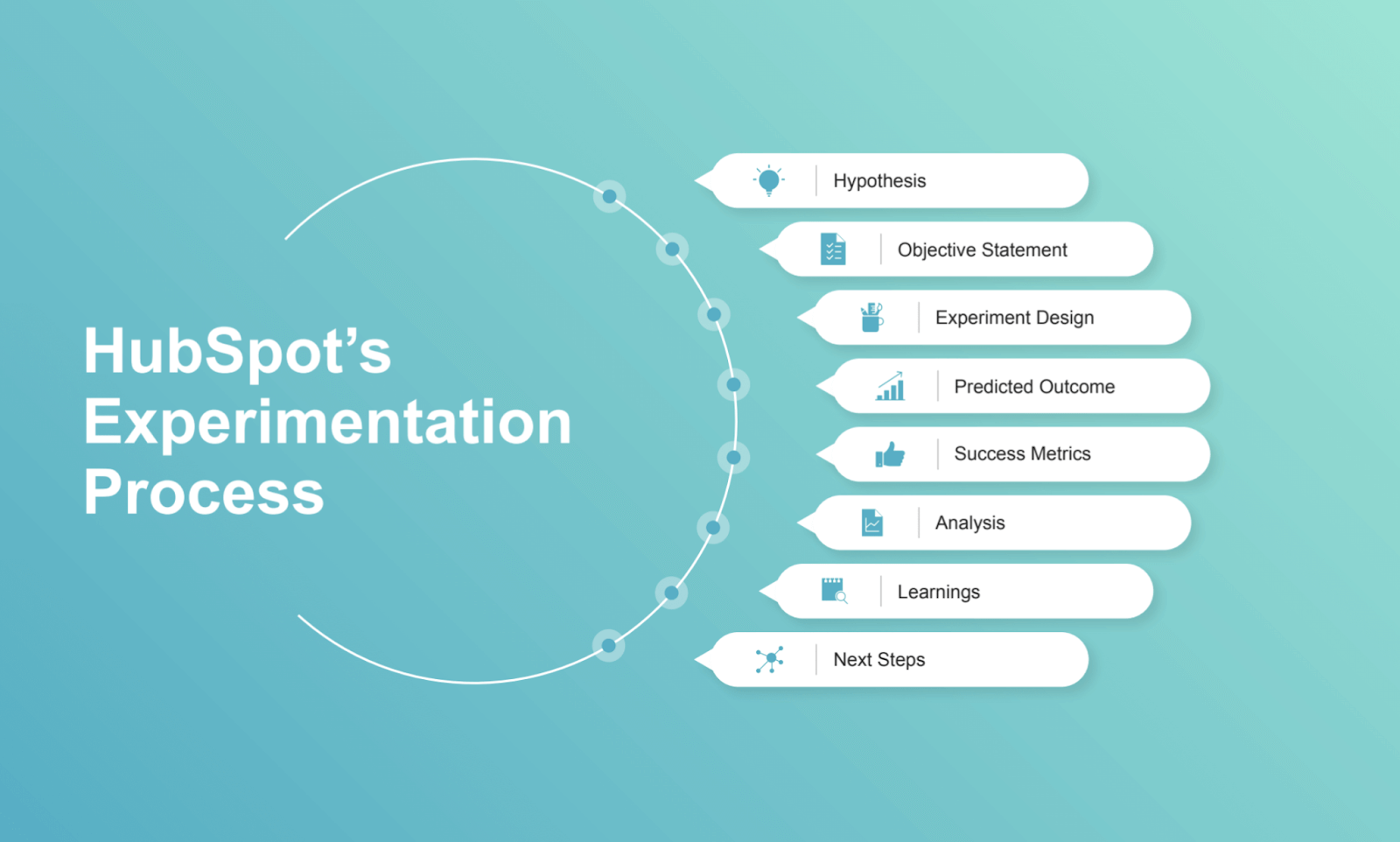
There's a hypothesis, objective statement, experiment design, predicted outcome of each idea, success and how we define that, analysis of the experiment, learnings, and so on.
Hypothesis
Every experiment should start with a hypothesis. The mistake folks tend to make with a hypothesis is it's a prediction instead. They say, 'if I do x, then y will happen' but that's a prediction.
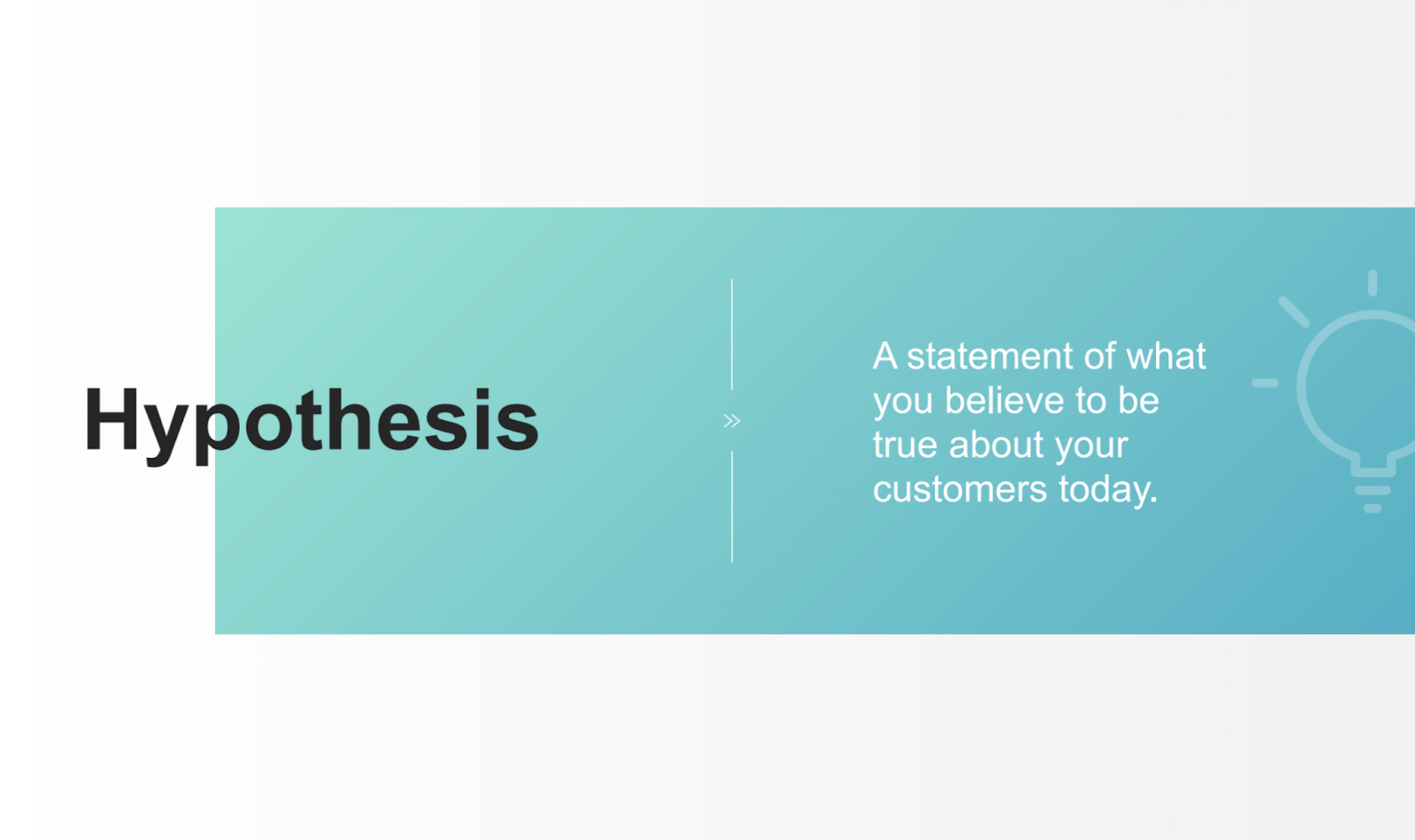
The hypothesis is just a statement of what you believe to be true about your users or customers, whoever you're thinking about. Don't mistake prediction with hypothesis.
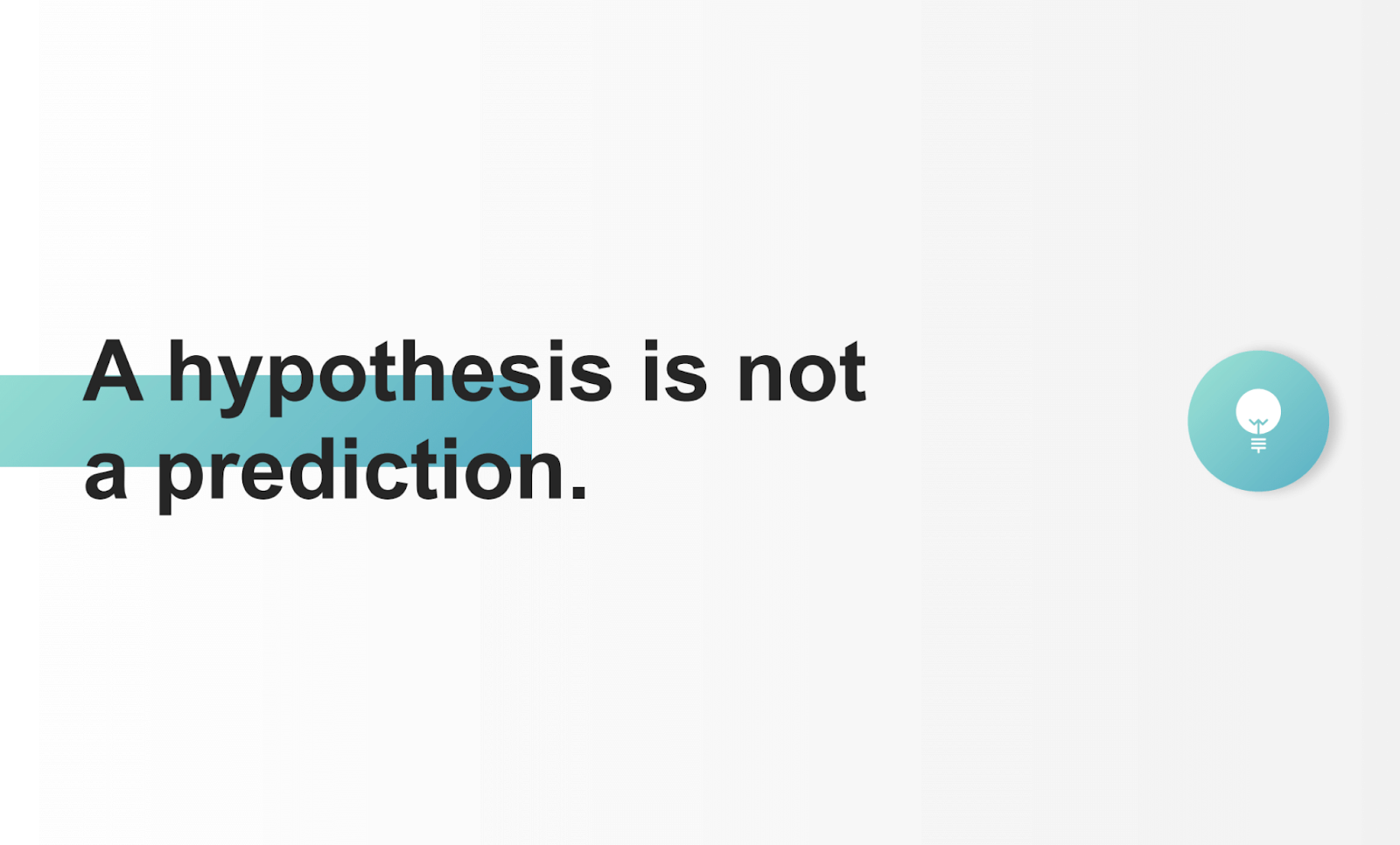
Example hypothesis
In one of our experiments, the hypothesis was we believe people are overwhelmed by all the questions in our signup flow.
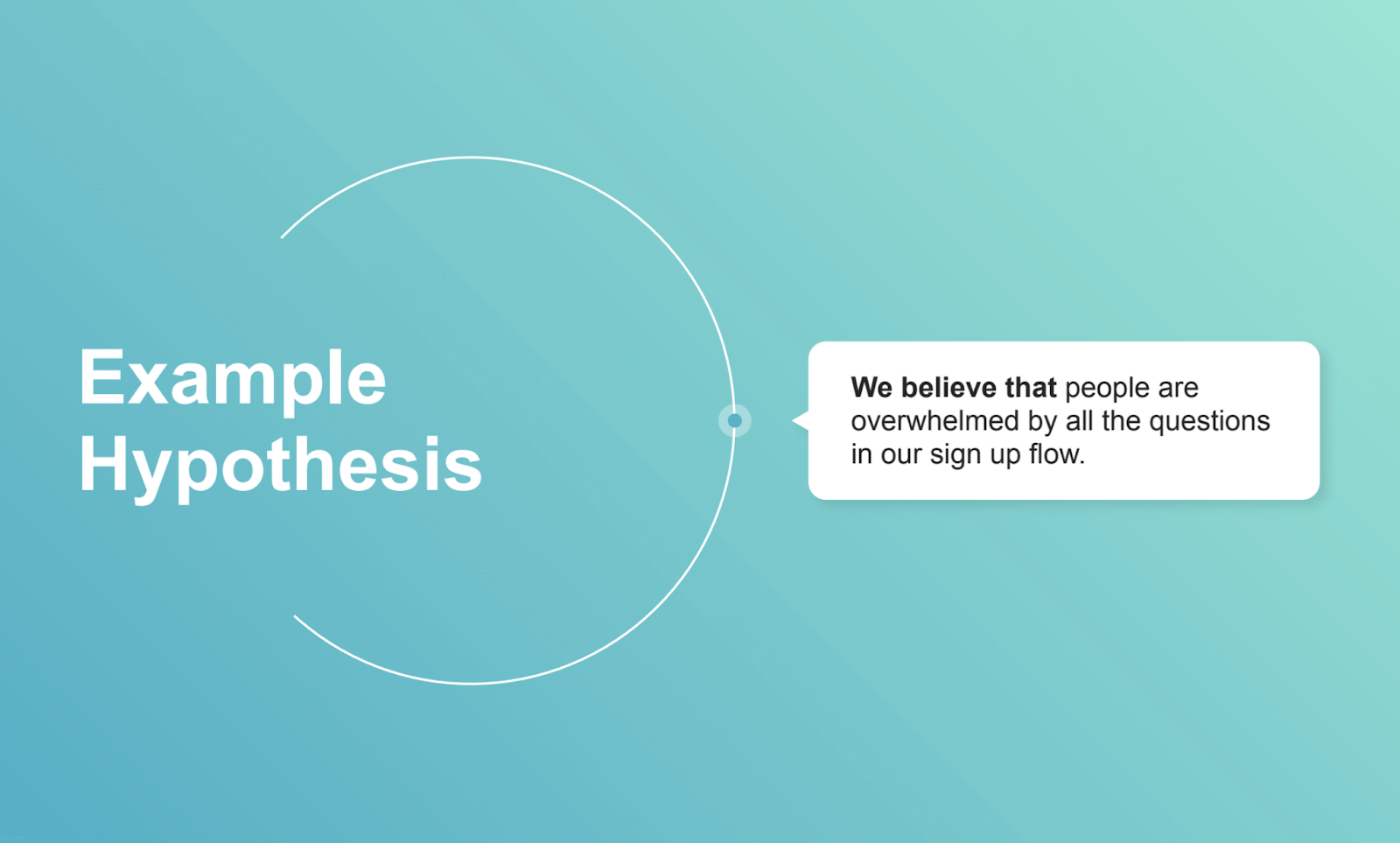
If you've ever gone through HubSpot signup flow in the last couple of years, we ask a lot of questions, there are at least eight screens you have to go through to make a free account and we realized we should probably change something about that.
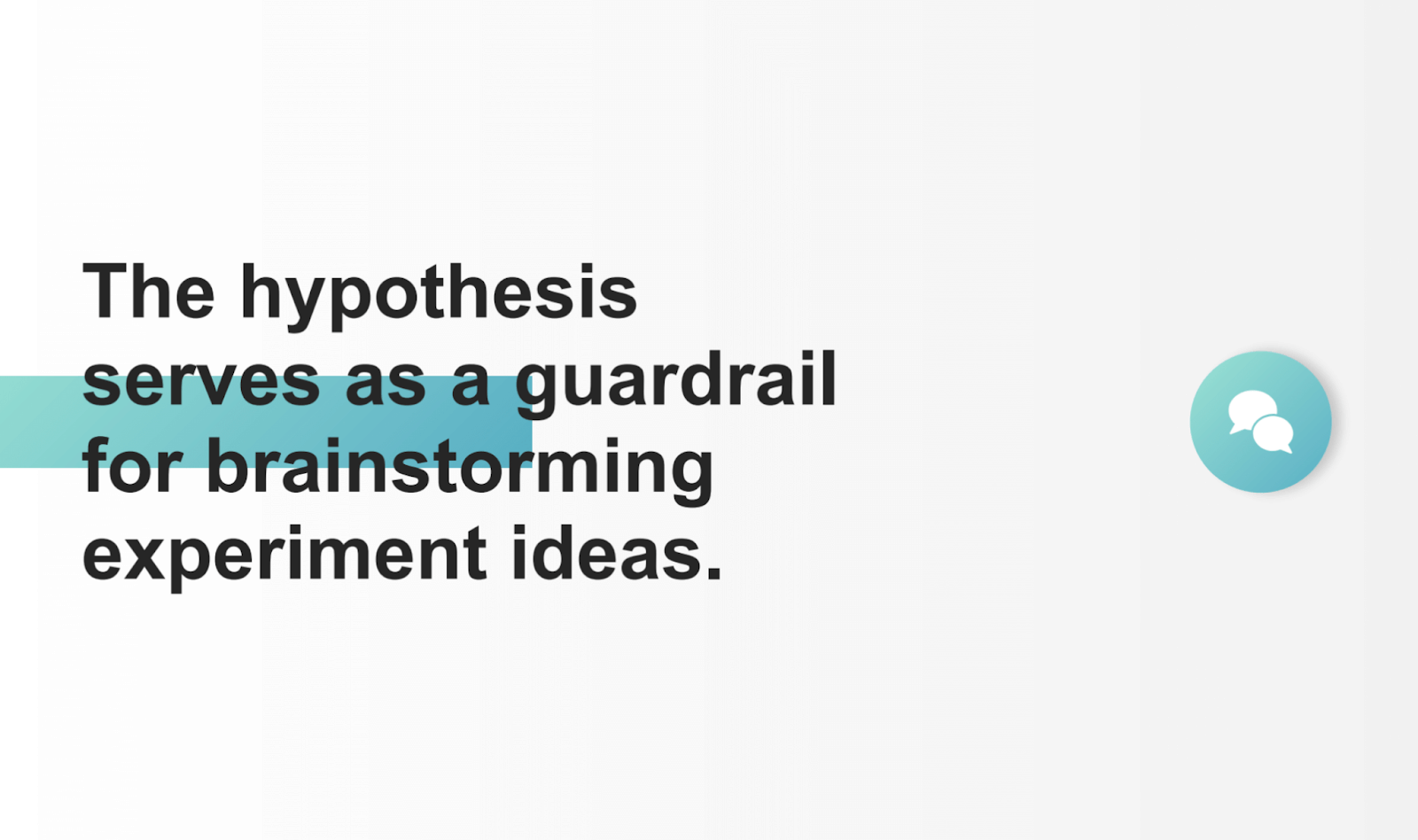
Prediction
Once you have the hypothesis, that is what allows you to start brainstorming ideas around that. Once you have those ideas, you can make predictions based on what you think those ideas would result in.
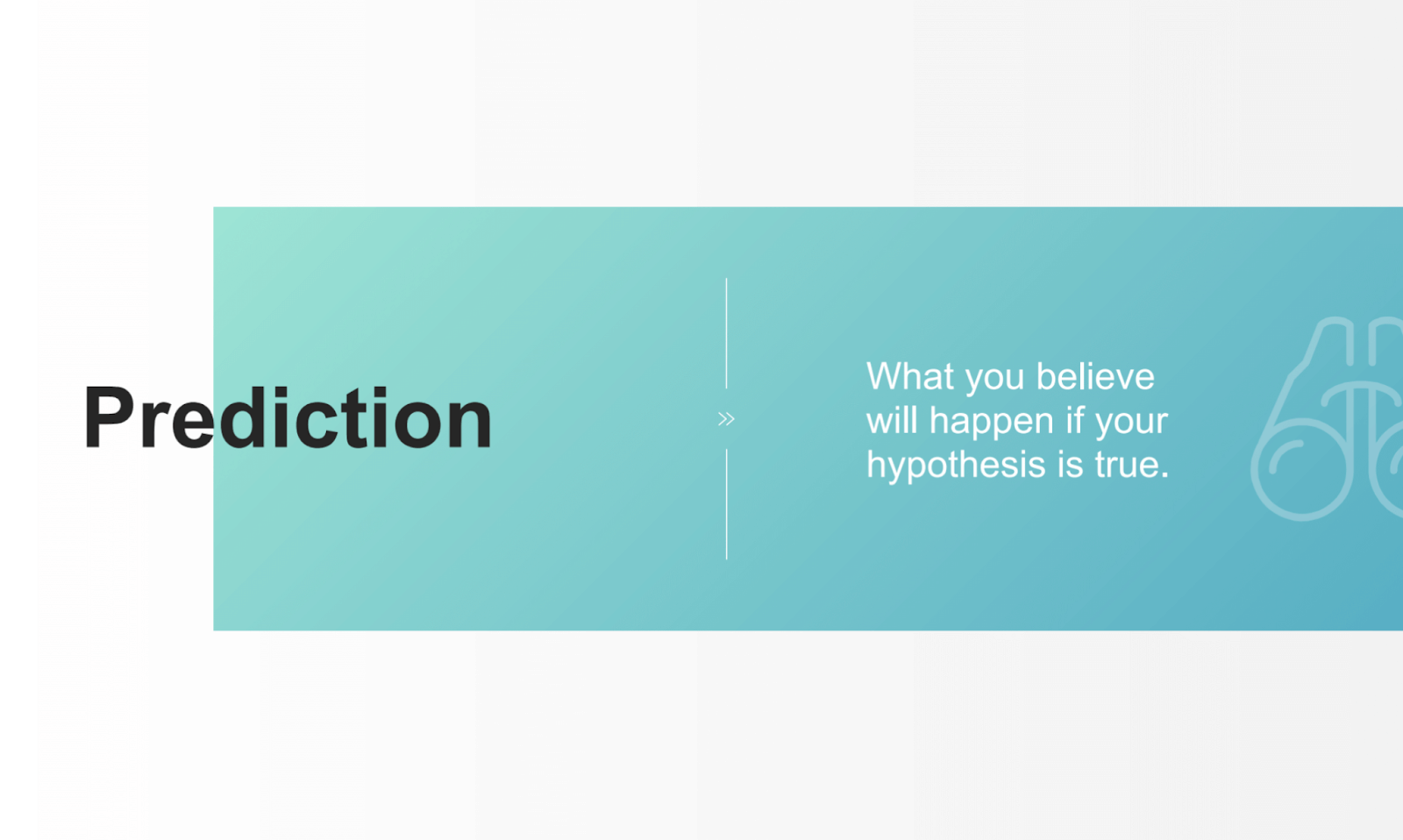
Example prediction
An example is if our hypothesis is true, then if we show social proof on the signup flow that might make people feel more comfortable and finish the signup process.
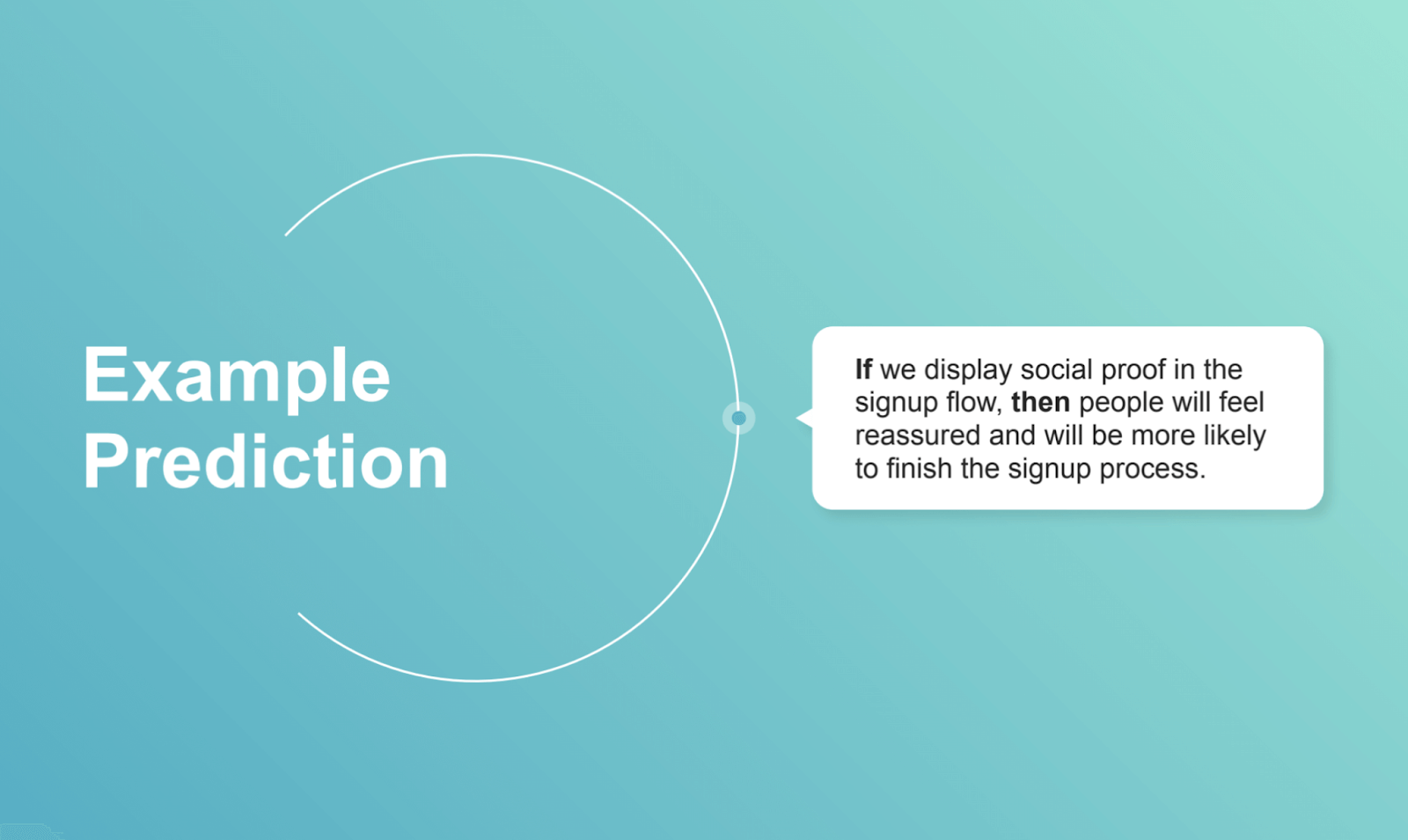
Again, it started off with the problem that we think the signup flow is too long, people are not finishing it, we think our users are overwhelmed by all that, what can we actually do?
The solution is maybe social proof. That's an idea and we finally worked our way down to the solution to think about.
Brainstorming in action
I want to give an example of how we could brainstorm if you're thinking about improving the conversion rate. Here's someone starting off at the homepage at HubSpot.
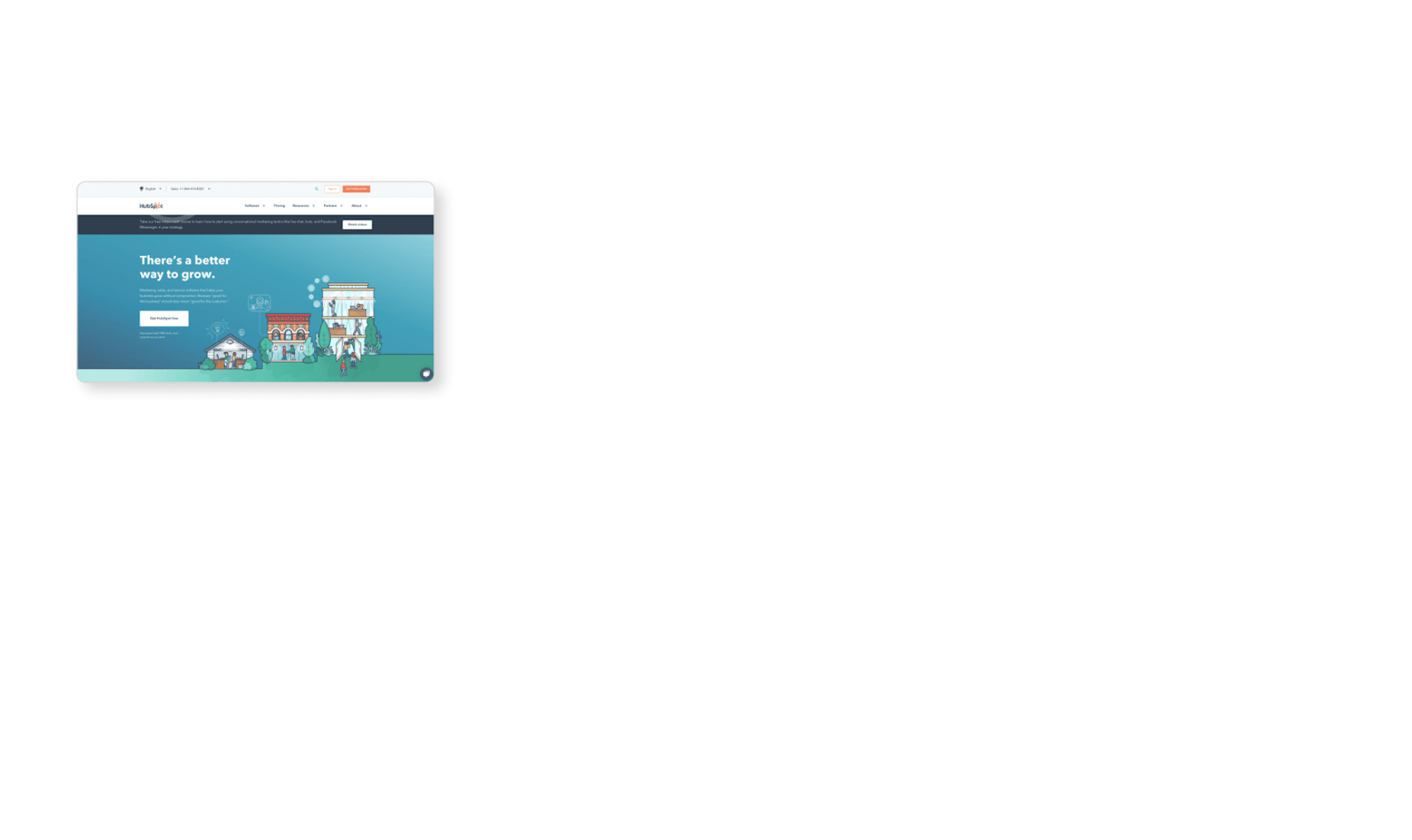
They click to get started and they learn about our products.
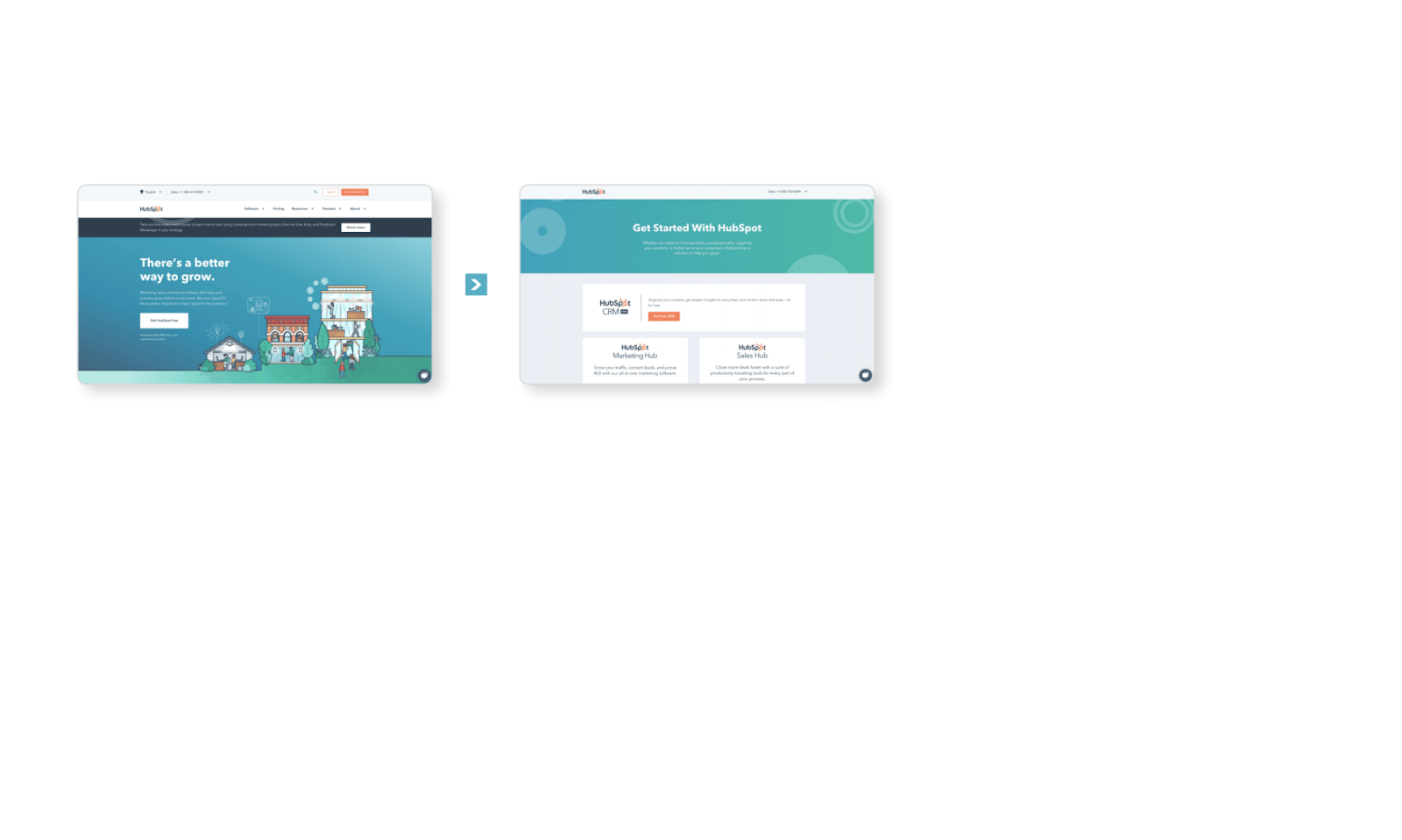
They decide to sign up where that first screen is giving their name, email password. Pretty normal.
Then we asked for information about their website...
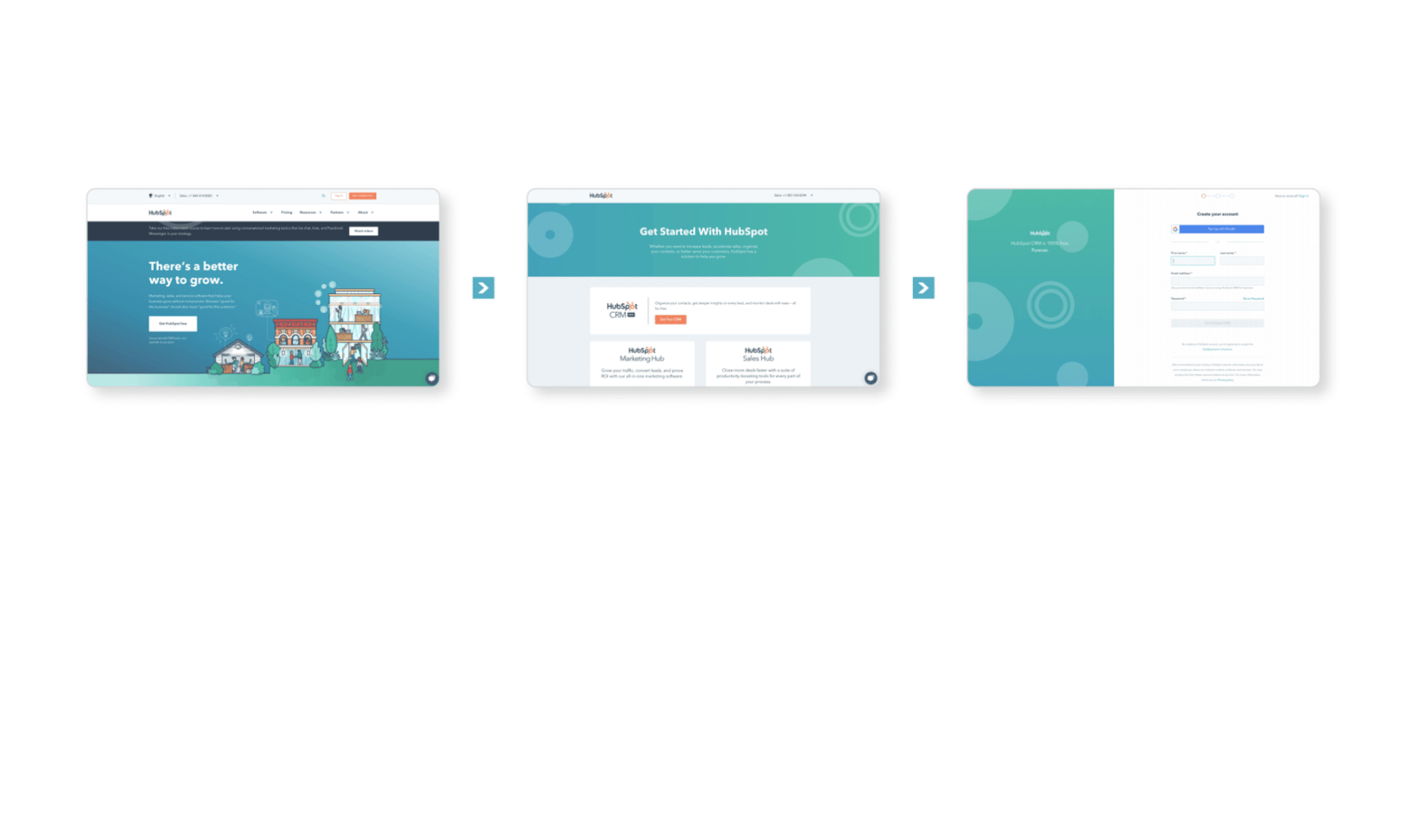
We ask for some more information about their role.
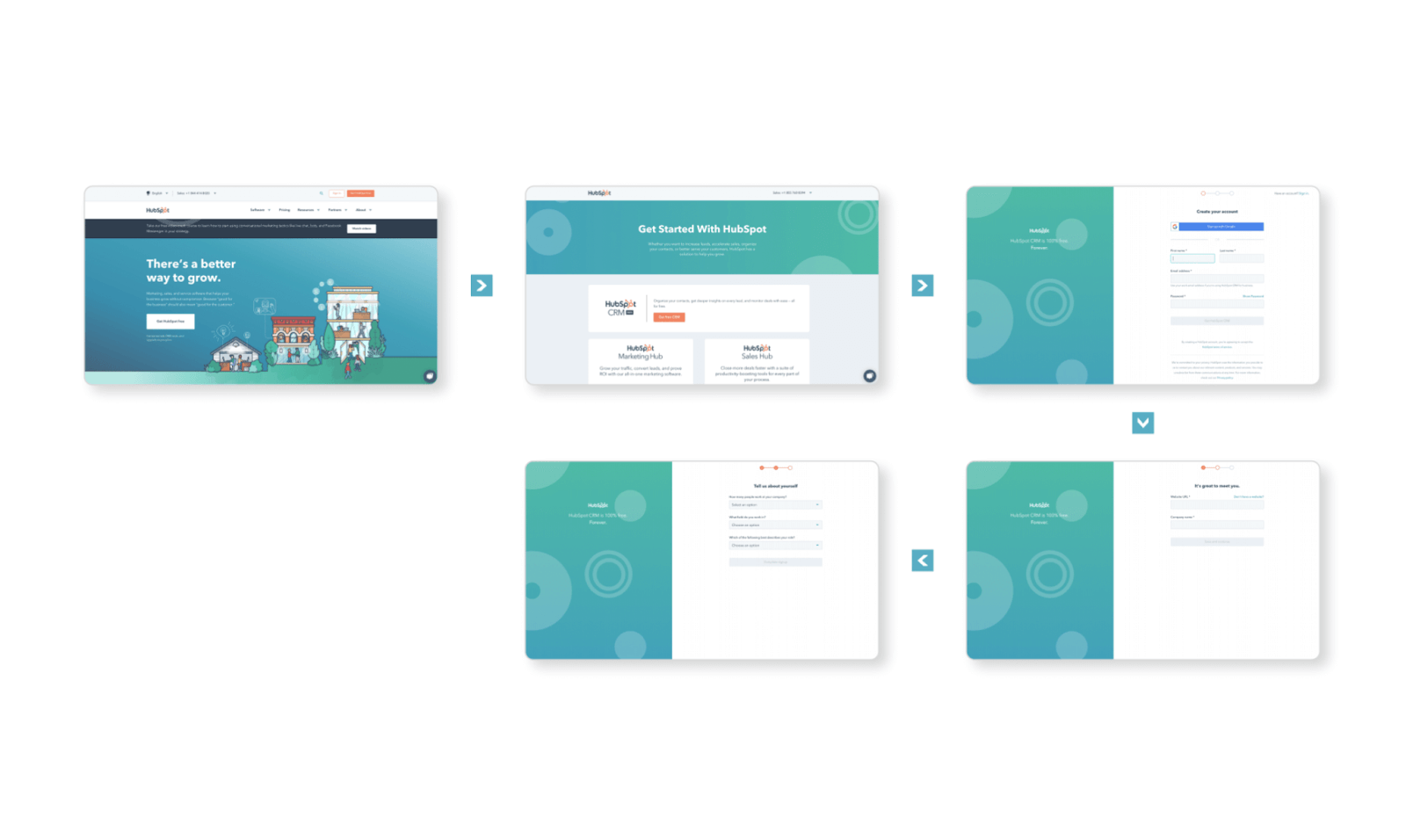
Finally, they get into their product.
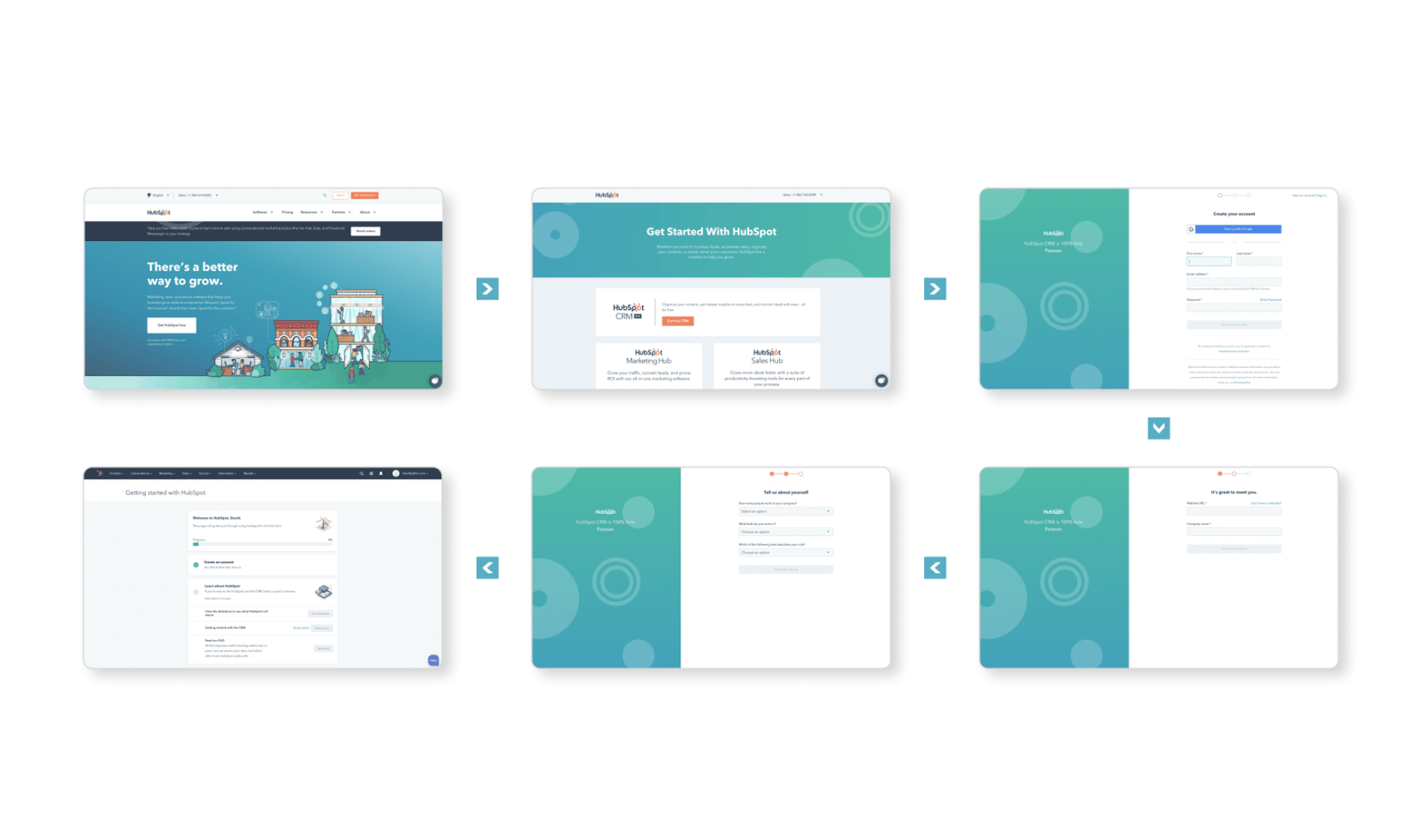
Now, when we were brainstorming about this and thinking these are too many screens, what can we do to improve this?
We were thinking if they're overwhelmed, maybe we can combine the first two steps so it feels like there are fewer steps and it's less effort?
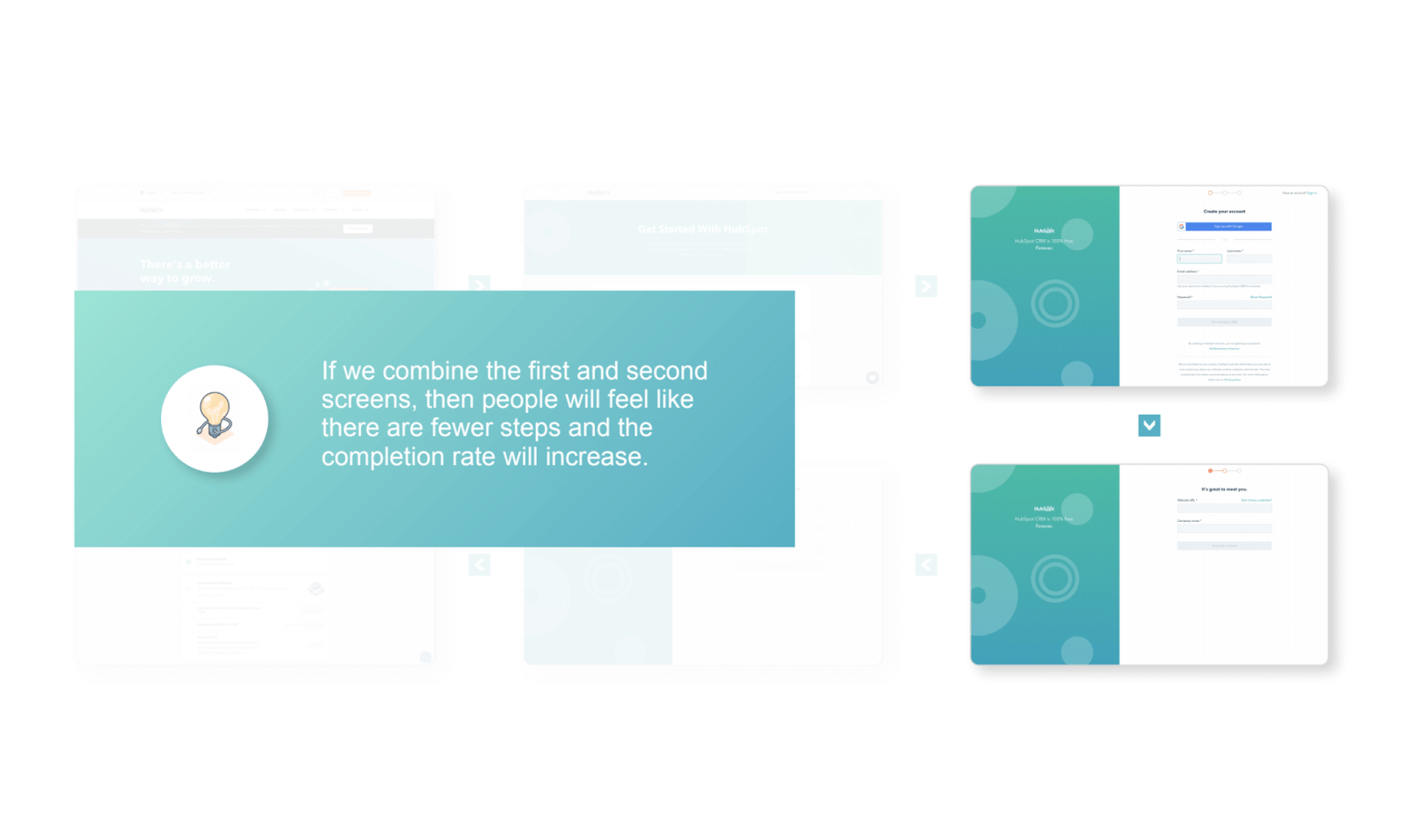
Or what if we just removed a step? What if we can ask this question later on?
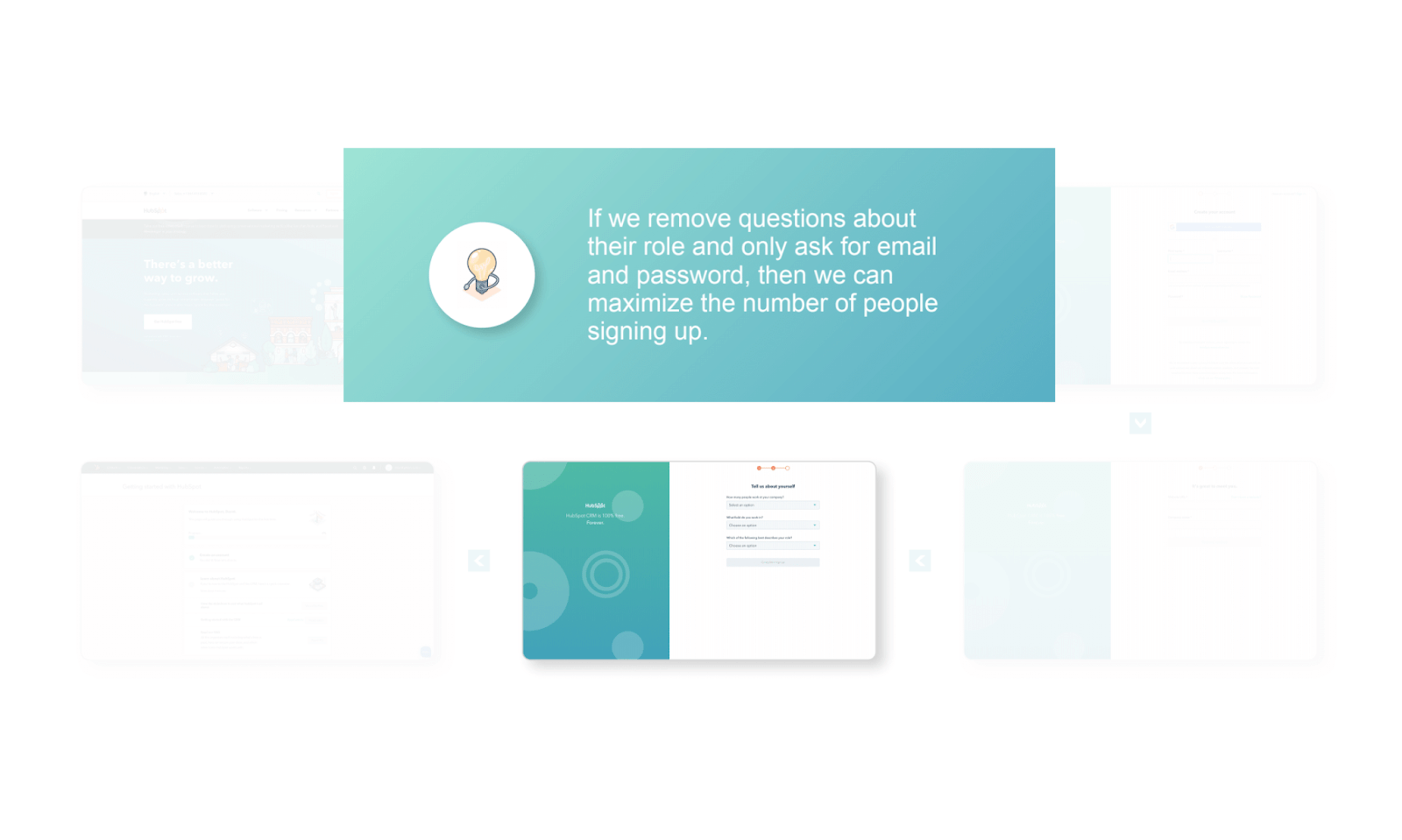
Or what if we just show social proof?
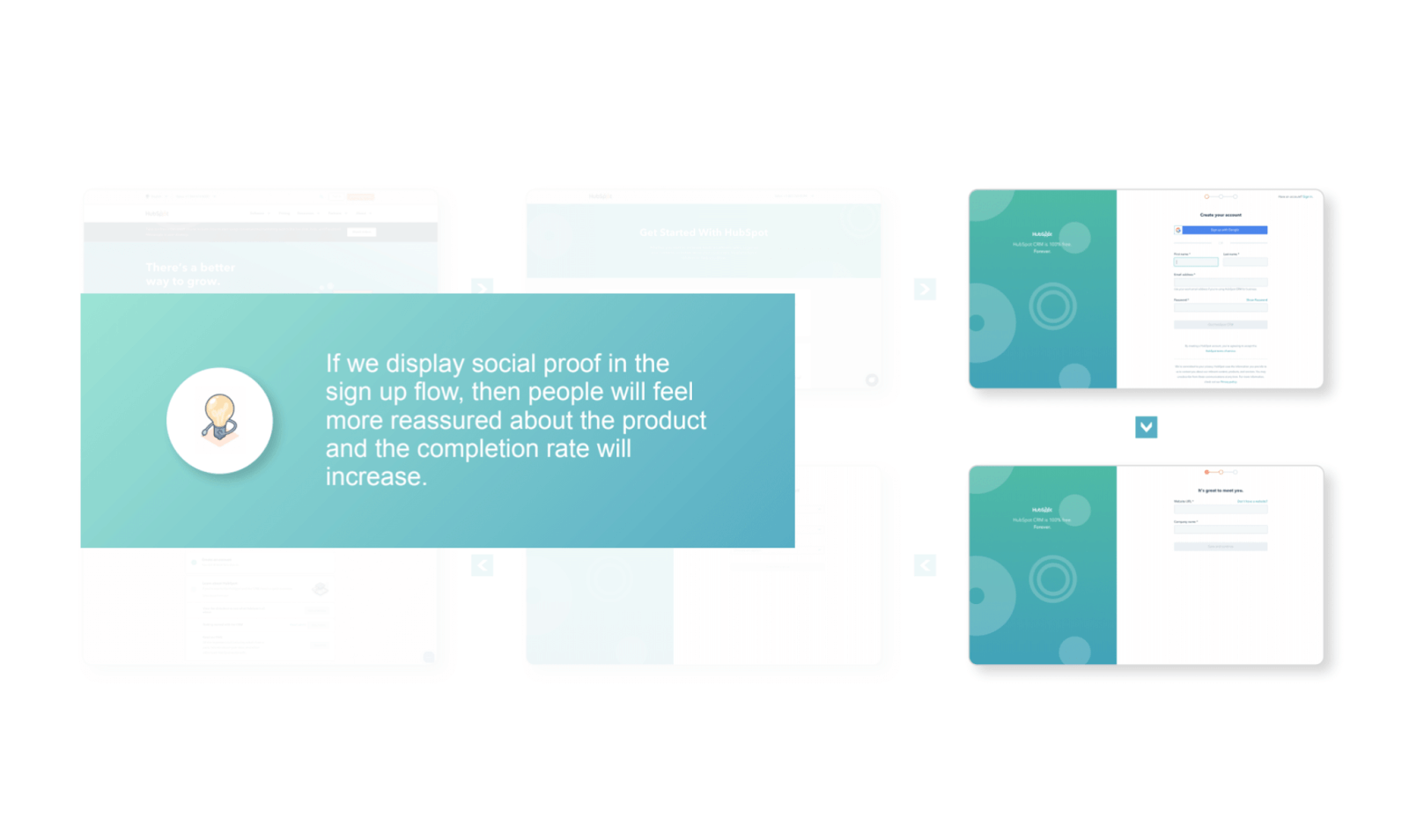
This is going to be the example that I'll focus on throughout this article.
Impact & effort
Taking a few steps back to when you have your hypothesis and start brainstorming ideas, you want to map those in terms of impact and effort.
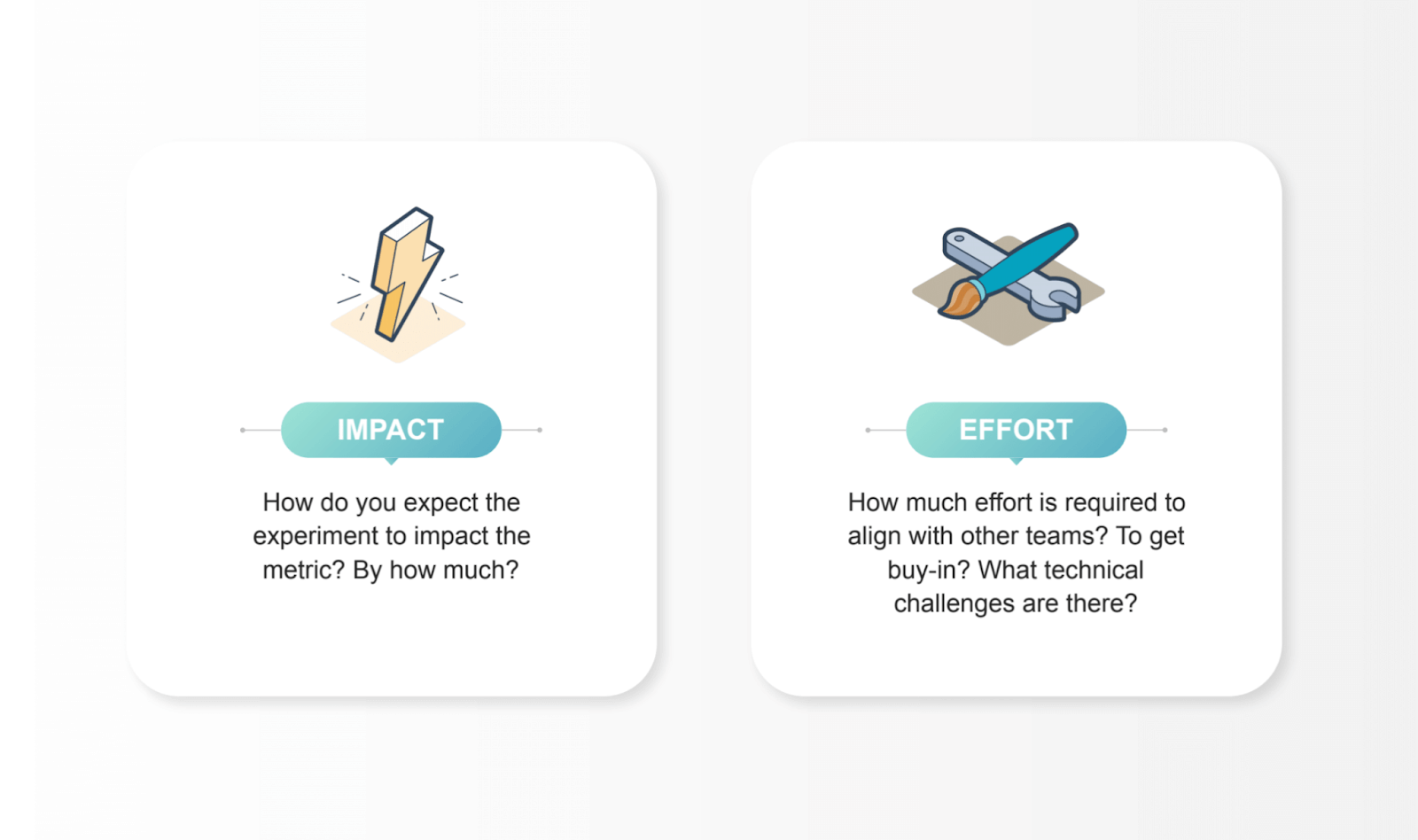
If you've ever used the PI framework to think about prioritizing ideas, it's very similar.
Impact
You think about how much impact you think this solution or this experiment could have on your metrics.
This is a very squishy concept, it's really hard to predict what's actually going to happen. But it makes you start reasoning why you think it will be impactful and actually have a strong argument for it.
Effort
For effort, you're thinking both technically, and also, when you run an experiment, you might affect other teams, you might decrease the number of leads potentially, or you might increase it a lot.
You might want to tell the sales team you should expect a decrease or an increase. We don't know yet. But how many teams you need to talk to, to let them know this is going to happen as well.
We love two by two matrices so we have this chart:
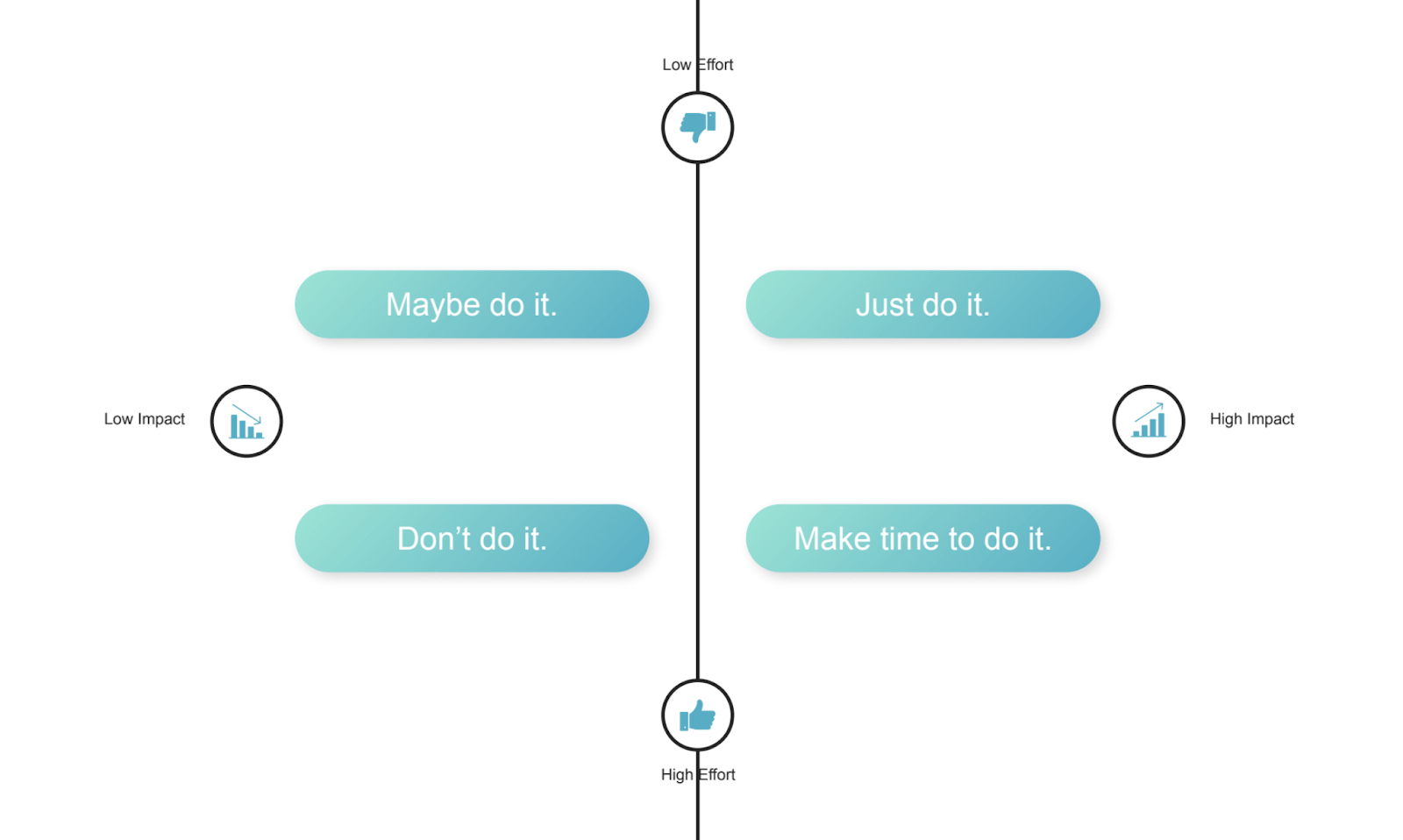
- Things that are high effort low impact, potentially don't do it, don't waste your time on that.
- There are things that are low effort, low impact, which you maybe do it.
- There are things that are high impact and high effort, which are going to be really big things that you want to tackle and you need to make time to do.
An example of this is redesigning your homepage, for instance, you might realize it's old, no one's touched it in a while, no one's tested anything on it, maybe you should make time to actually do that and go through the process of redesigning it.
- Things that are low effort, high impact you should just do.
What we like to do is we'll get a whiteboard, we'll draw out this two by two matrix, and it will have ideas on stickies. We'll pull up a sticky note, there might be a room of four or five people and say, is this low impact or high impact potentially? Is this high effort or low effort? And we'll put it up.
By the end of the session, we'll have some quadrants that are more full than others. We might start double-clicking on some and saying:
- Is this actually low effort?
- Or is it high effort?
- Should we revisit this?
- Or do we actually think it's here?
- Should we dig into this further?
Objective statement
Once you decide to move forward with one of your ideas for an experiment, this is one of the most straightforward things, but having an objective statement is super important.
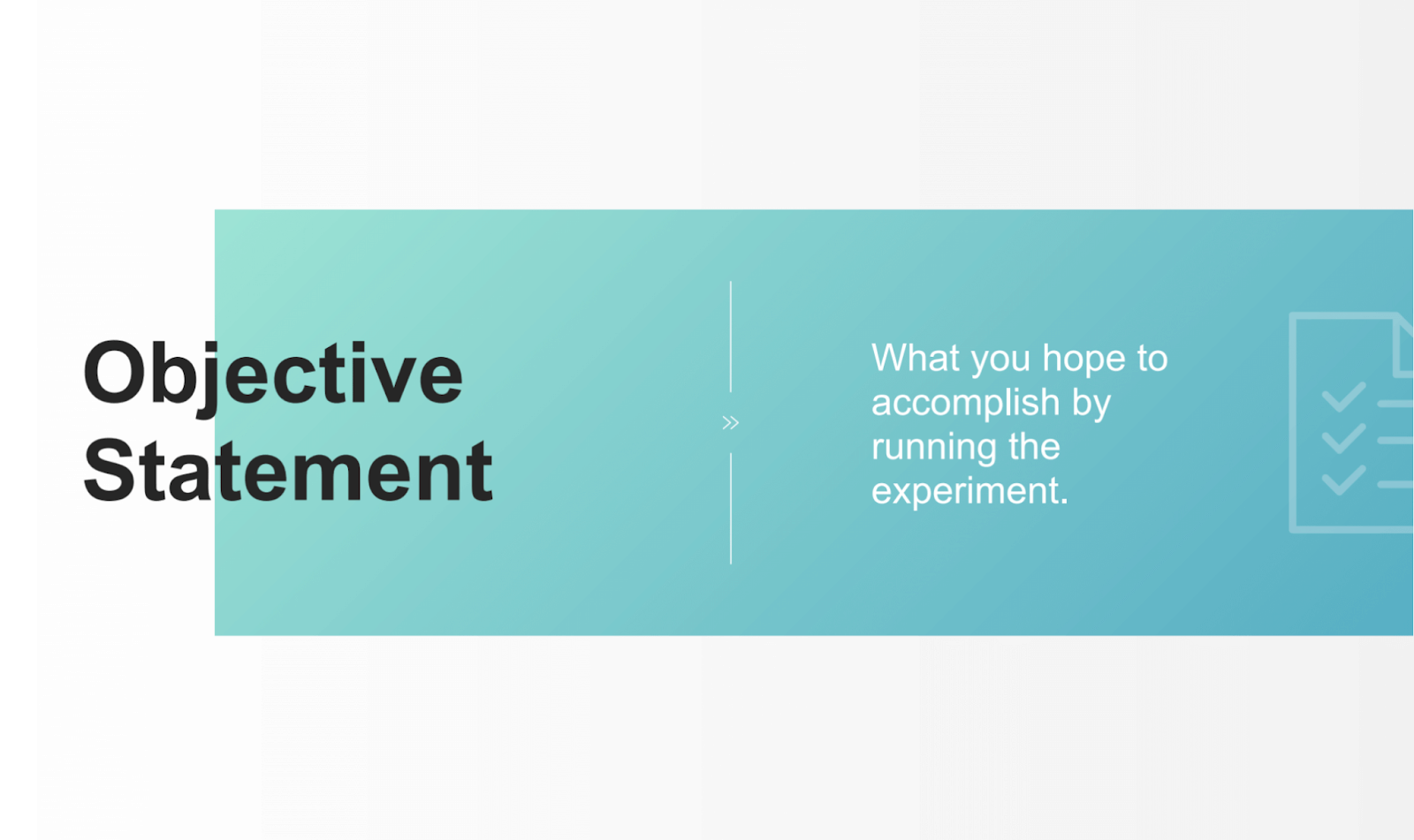
It's just stating what you want to accomplish with the experiment. Some people might say, isn't that kind of useless or redundant?
But the thing is, once you start having a meeting where you're discussing details and very granular things, or implementation, you might realize that everyone's completely lost sight of the goal of the meeting or the experiment.
No one knows what to say, because you don't want to be that asshole that says, I think you're talking about something completely unrelated.
When you have an objective statement stated, you can actually say, if our goal is to do X, to improve signups, does talking about this really specific thing actually help? Let's take a couple of steps back and refocus on what we're trying to achieve here.
Generally, when someone does that, and we do that often at HubSpot, everyone says thanks, let's talk about this other thing later.
Example objective statement
An example of our objective statement was that we want to increase signups.
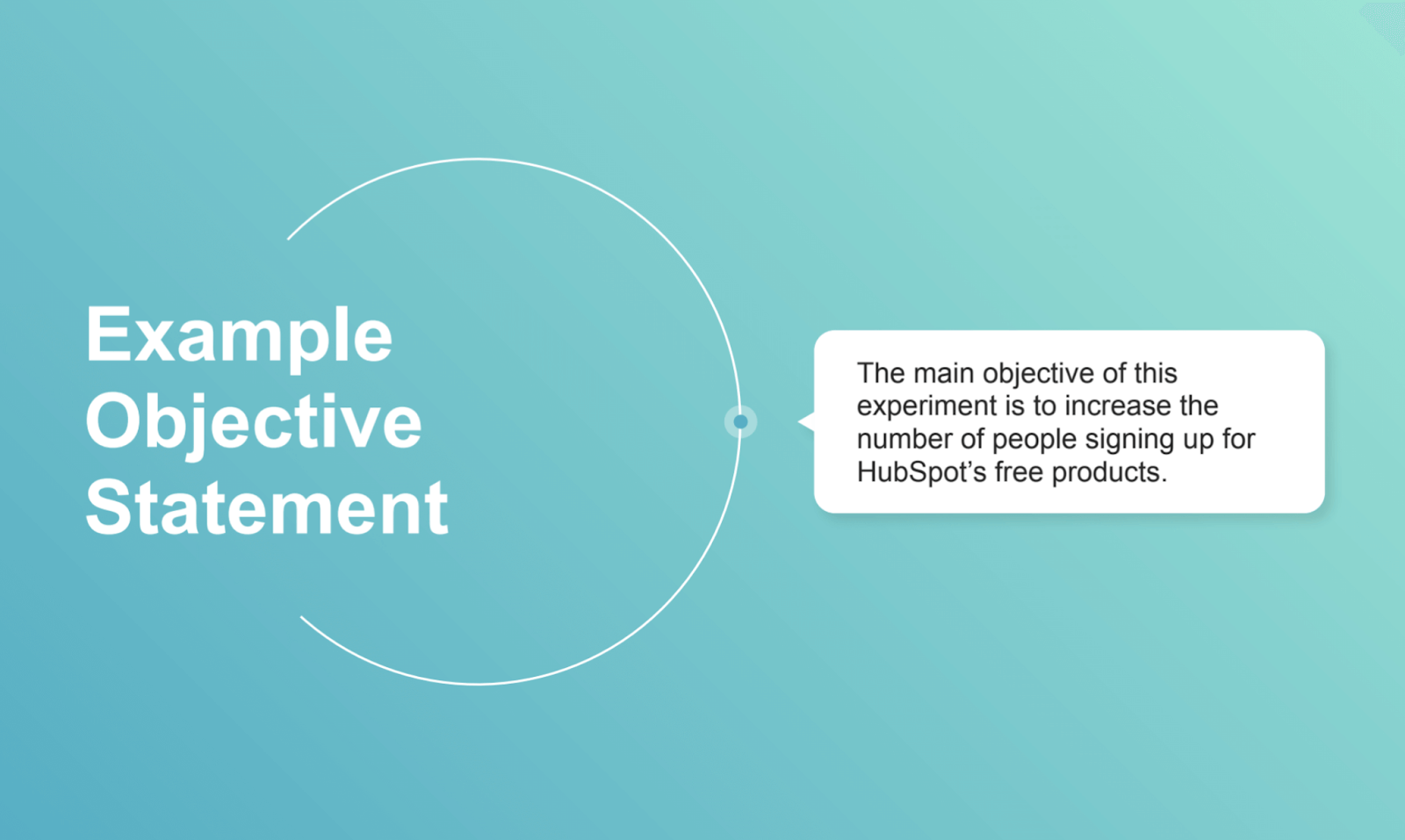
Once we move forward, we decide if we want to do this, let's start designing the experiment.
Experiment design
The goal of this is to make sure we get all the data that we want, and enough of it, we know what variants we're going test, and whether or not those variants will answer the questions we have about our users.
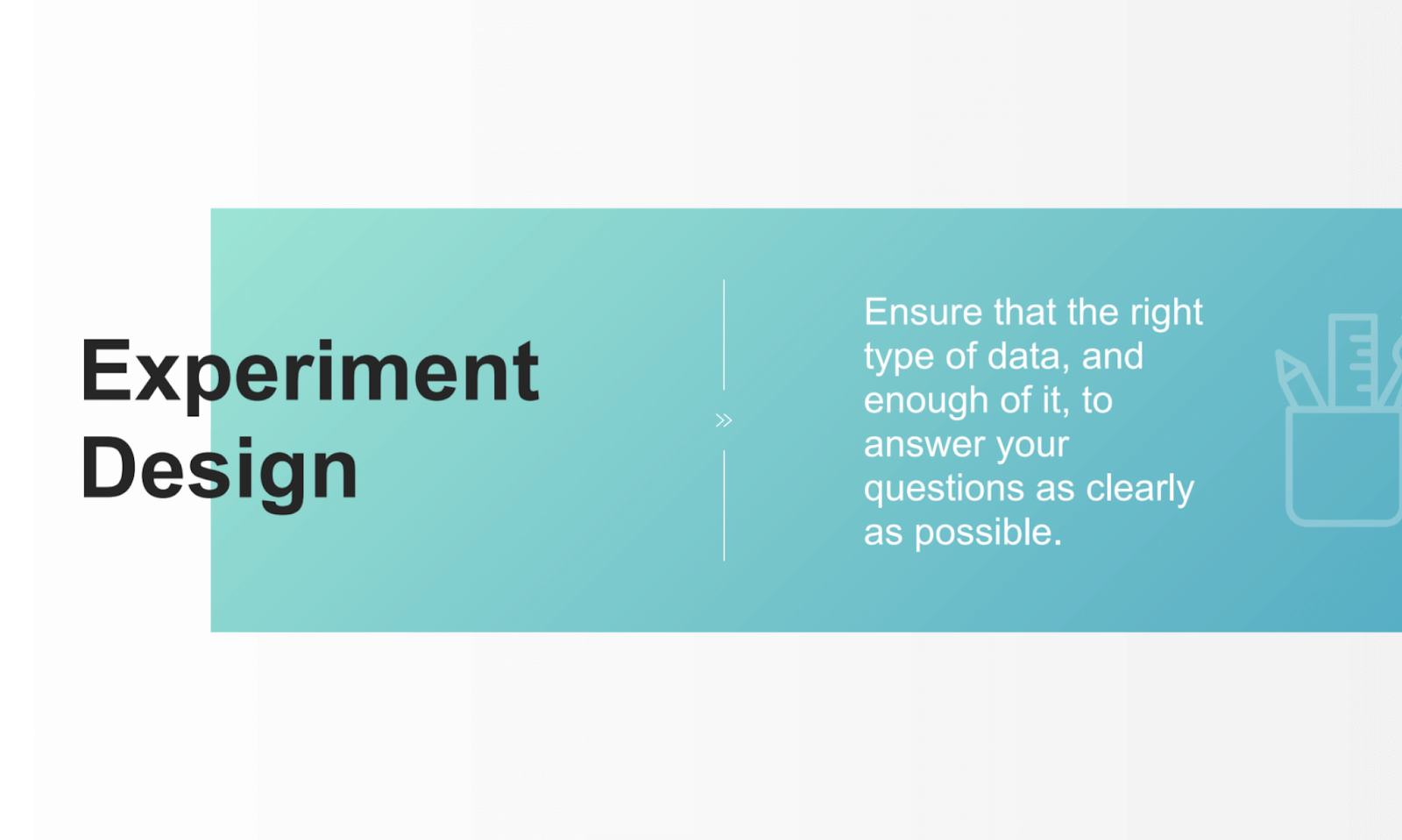
This is not an exhaustive list, but experiment design includes:
- How many variants you test - maybe you want to test three or four, which means it'll take longer than an AB test.
- You might want to actually talk about what the variants and designs look like.
- You want to know how many samples you need - that will define how long you'll have to run the experiment for.
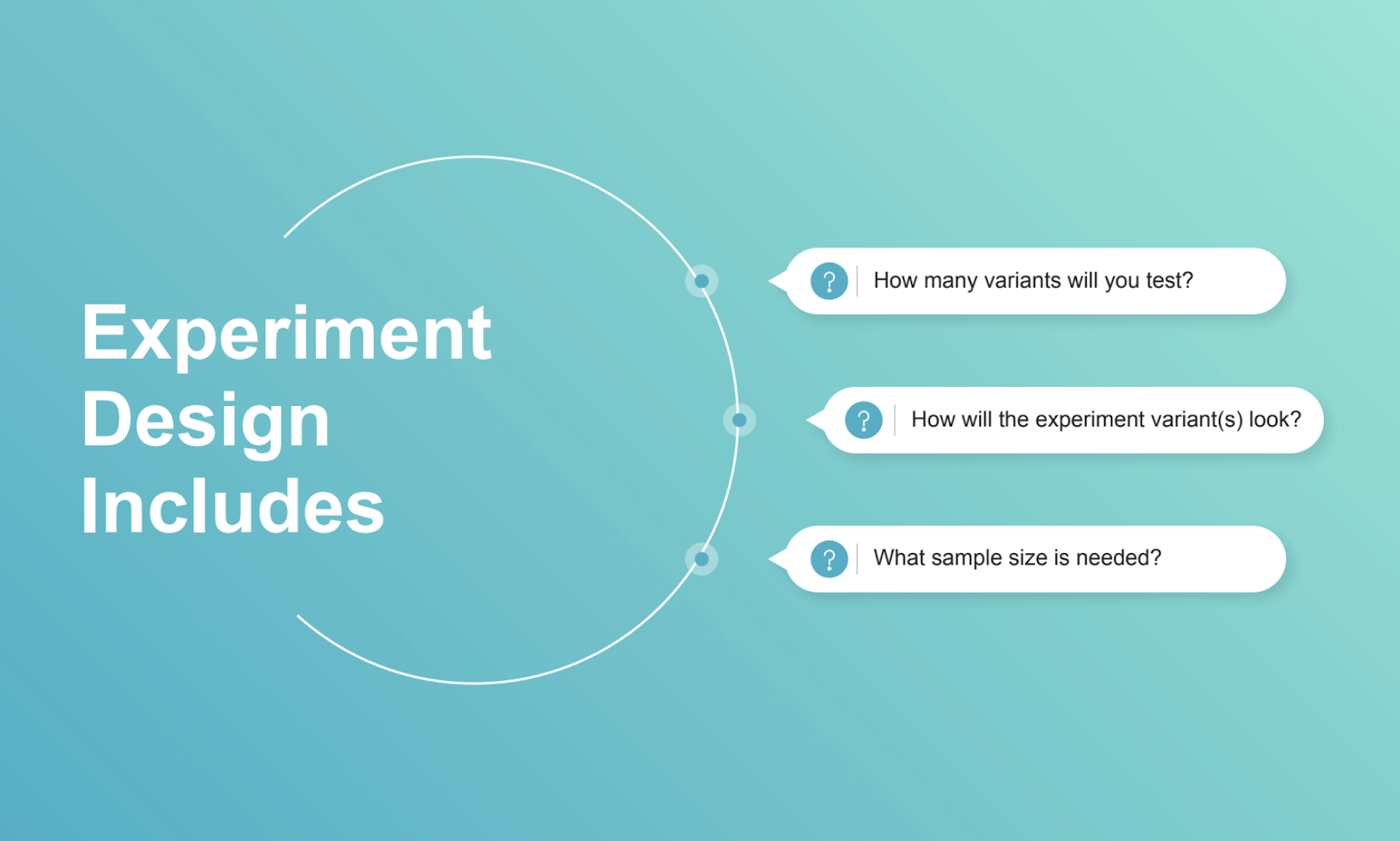
This is an example of what the signup used to look like before we tested it out. That's the control, very simple design, just a signup form.
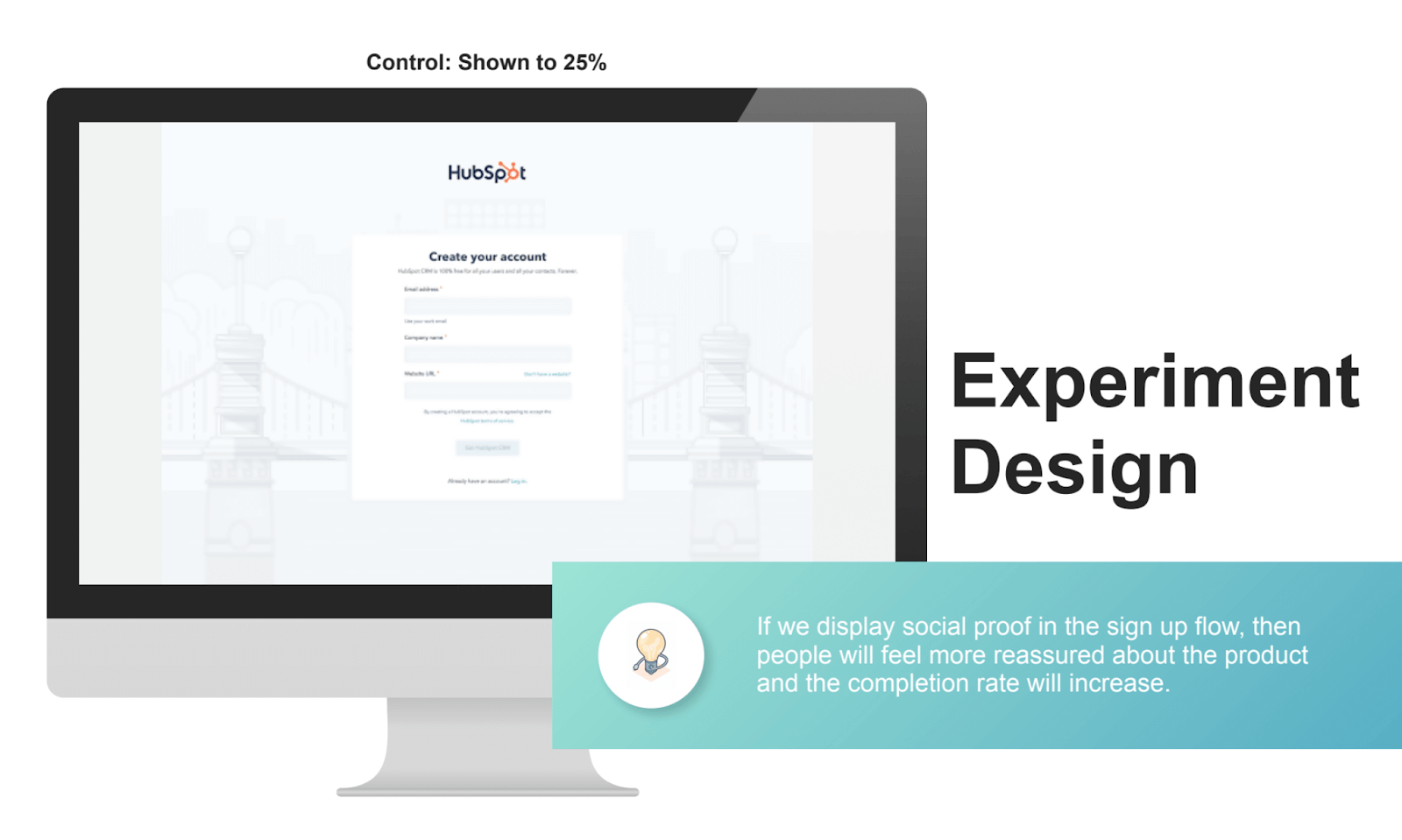
We said we want to put social proof on it. From there, we had three different variations we wanted to test. The one on the far left is a testimonial from Shopify, the middle one shows a bunch of logos of companies we thought would be recognizable by companies around the world, on the right was a bunch of awards that we've won.
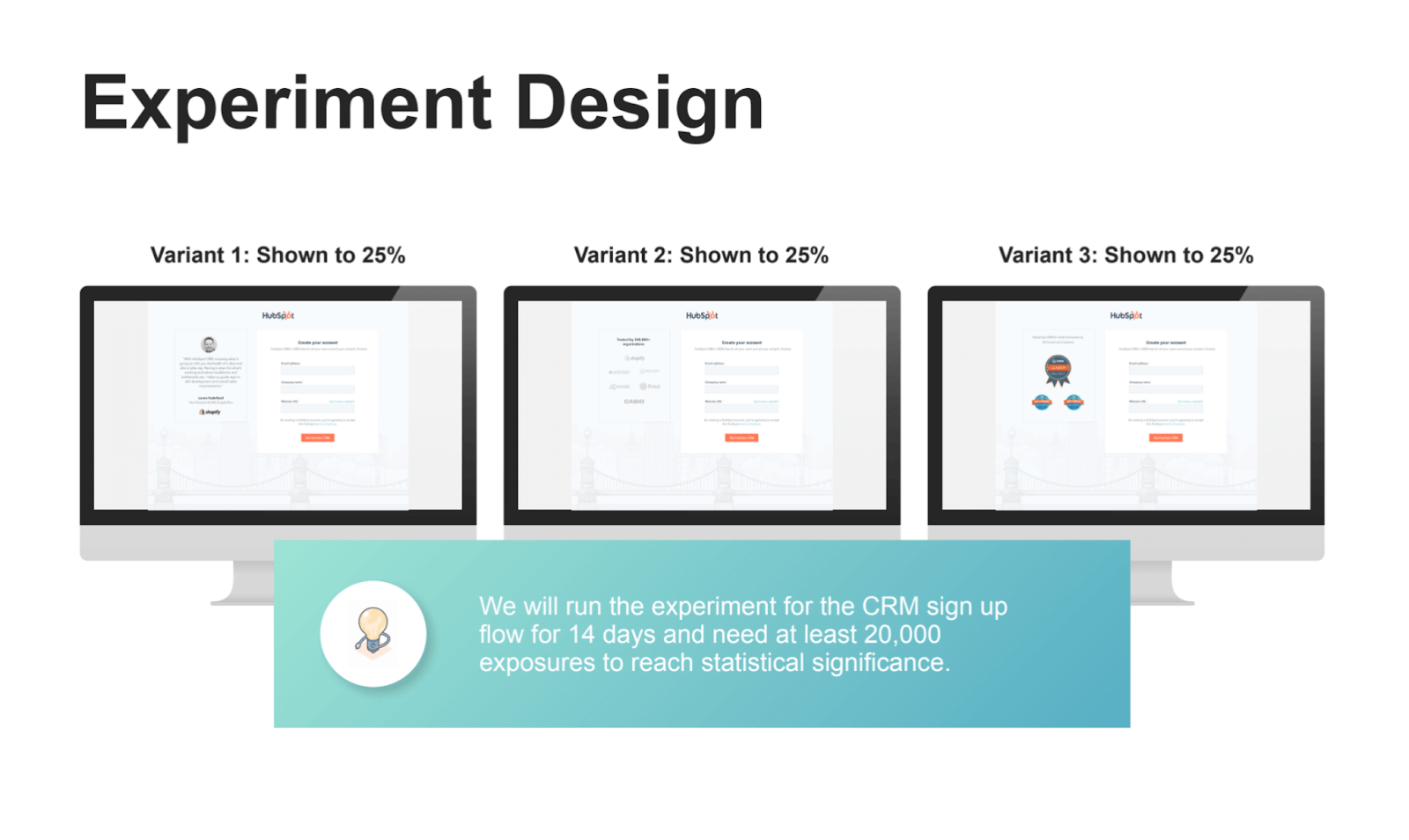
I'll talk about which one of those won later on.
These are fake numbers but we decided to run the experiment for 14 days and get at least 20,000 views to see if that got us to statistical significance.
Predicted outcome
Once you have the idea, and you've talked through it and the potential design, you want to talk about the predicted outcome. This is the if-then statement.
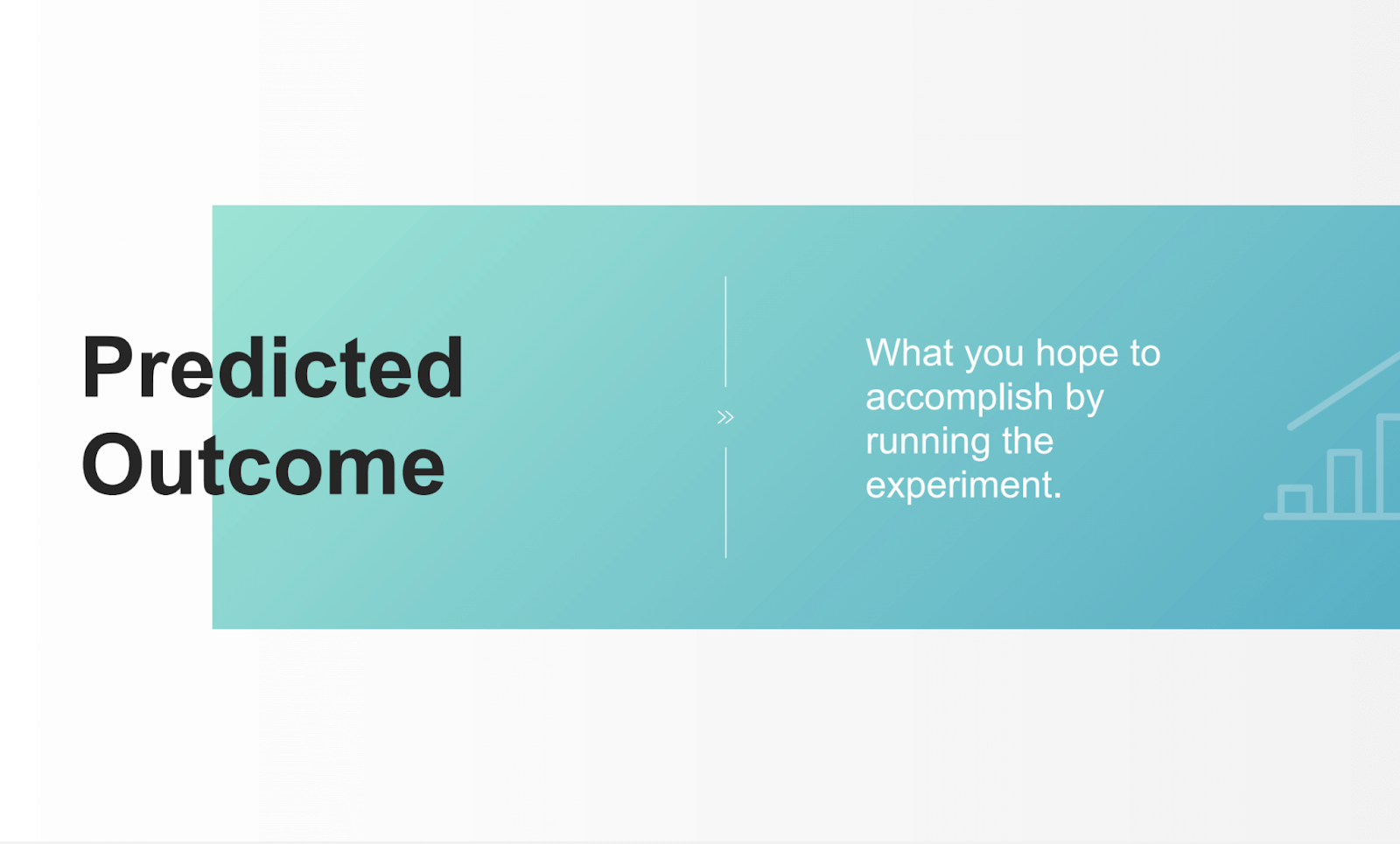
Example predicted outcome
You can start as simple as we predict that the completion rate will improve by five percentage points.
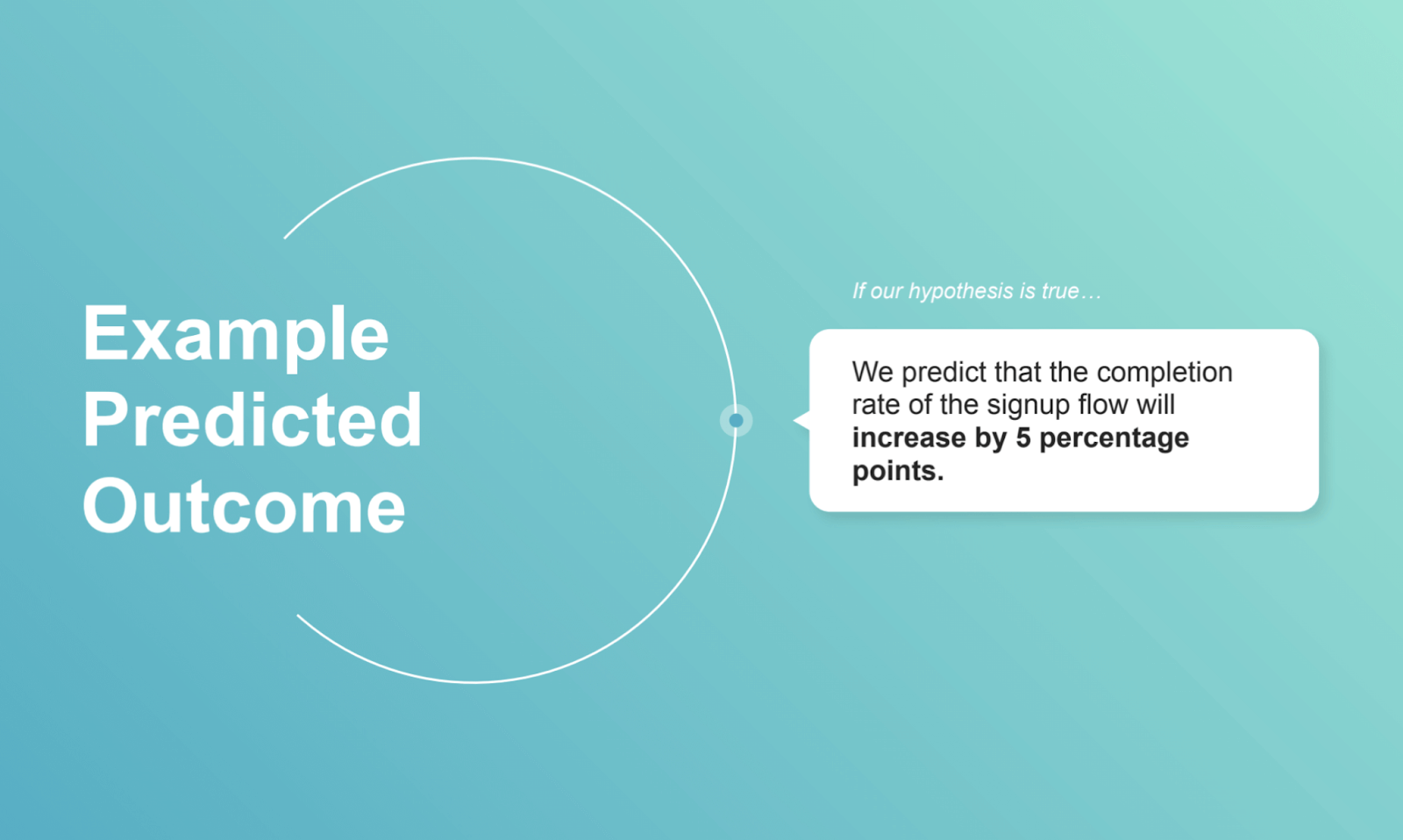
At HubSpot, and we encourage other companies to do this as much as possible, we map it all the way down to revenue.
We'll say if the percentage increases by five percentage points, that will translate to 300 new signups per week, and assuming that the upgrade rate is 10%, the average sales price is $20, which results in $600 in more revenue per month.
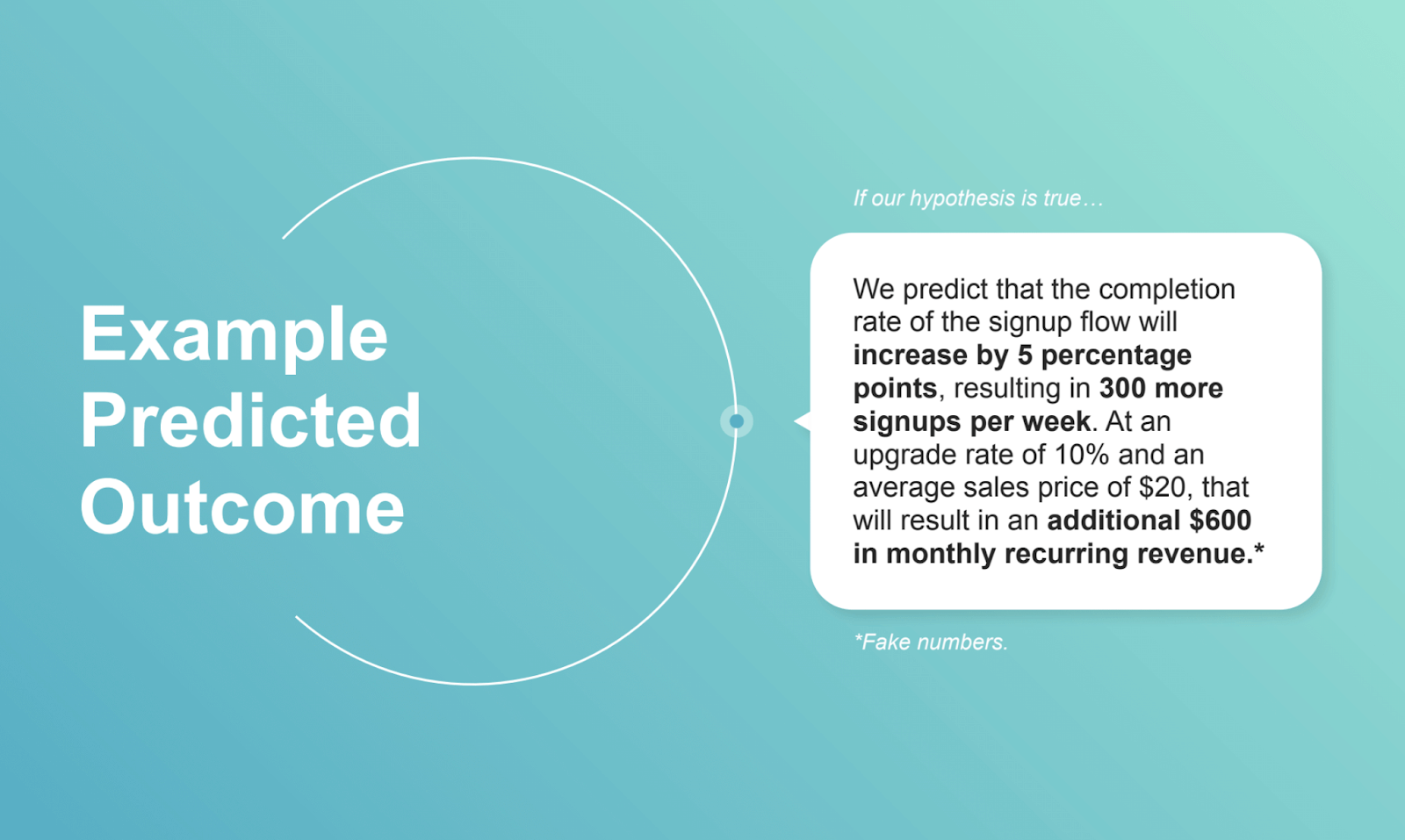
It's tough if you're a smaller company to map out this funnel. But if you do, it helps you really decide whether or not an experiment is worth running. Sure some people might change those numbers a little bit to start arguing that something's worth running when it isn't. But that's why peer reviews are so important.
Peer review
A peer review is a meeting with your immediate team that's talking about running an experiment and maybe a few other stakeholders are involved.
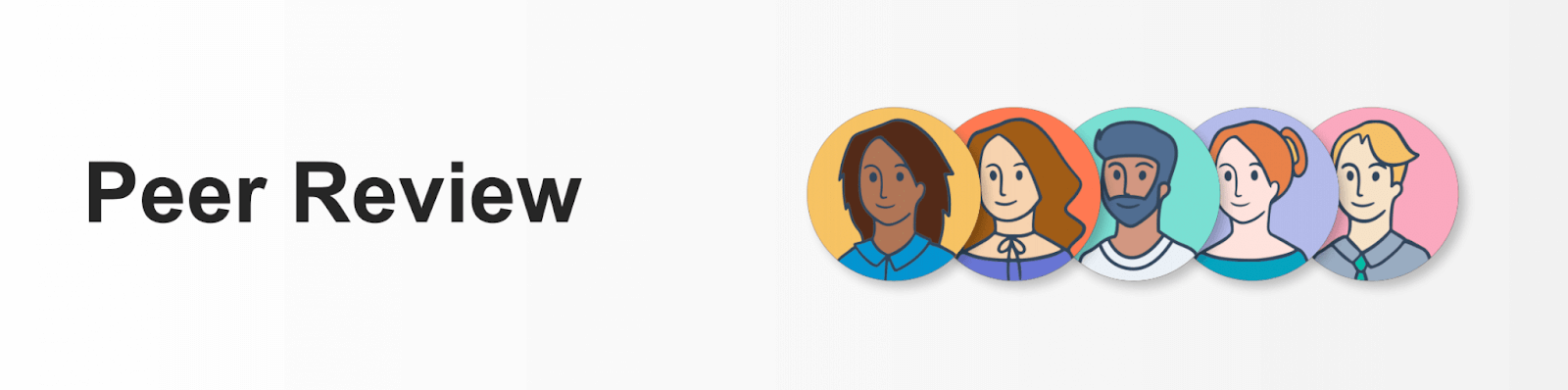
You'll talk to all those different items you walk through in that document. This is when people can just tear it apart. People will say:
- What makes you think this is true about our users?
- Why is this your hypothesis?
- Do you have data to back that up?
- Maybe your design should be different.
- Have you considered these different designs?
- I can help you with that.
- Is this even the right thing to focus on?
When it gets to the peer review, there's still an opportunity to say this experiment’s not worth running, which has actually happened.
It's kind of heartbreaking because you put a lot of time into researching it, pulling data, designing everything, then if someone catches one thing you missed that completely nullifies your experiment idea, you have to start from the beginning.
In some cases, you might realize your hypothesis is actually something else or there's a different way to test the hypothesis and then you have different ideas. But the peer review is what helps us have a really fine filter for running experiments.
It's when you have an open room and discuss very candidly whether or not something is worth moving forward with. For example, if you run an experiment on your homepage, which is very valuable real estate, that means you can't do anything else on that homepage for the time that experiment's running, which means it's a very valuable thing to do.
The experiment goes live
Once you do the peer review, hopefully, you get through it, you start running the experiment, whether it be for a week or two weeks.
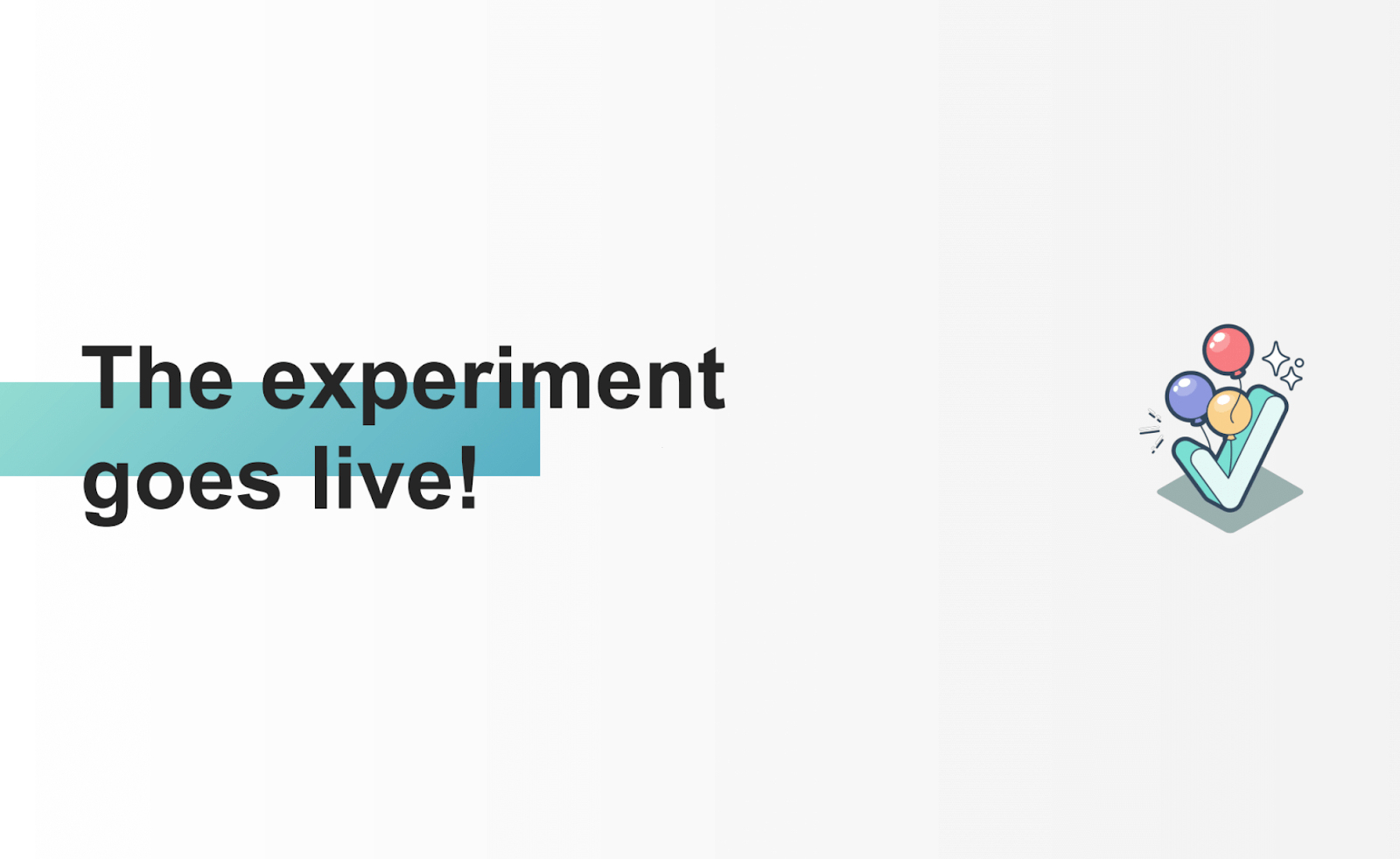
For us, we generally run it for at least two weeks, because we want to consider there might be fluctuations in traffic samples. If we run it just on one half of Wednesday, we don't want to just run through the next Wednesday.
Instead, we want a full two weeks in case it's a really strange week and there's a holiday or something like that.
Post-mortem
Then we have the post mortem
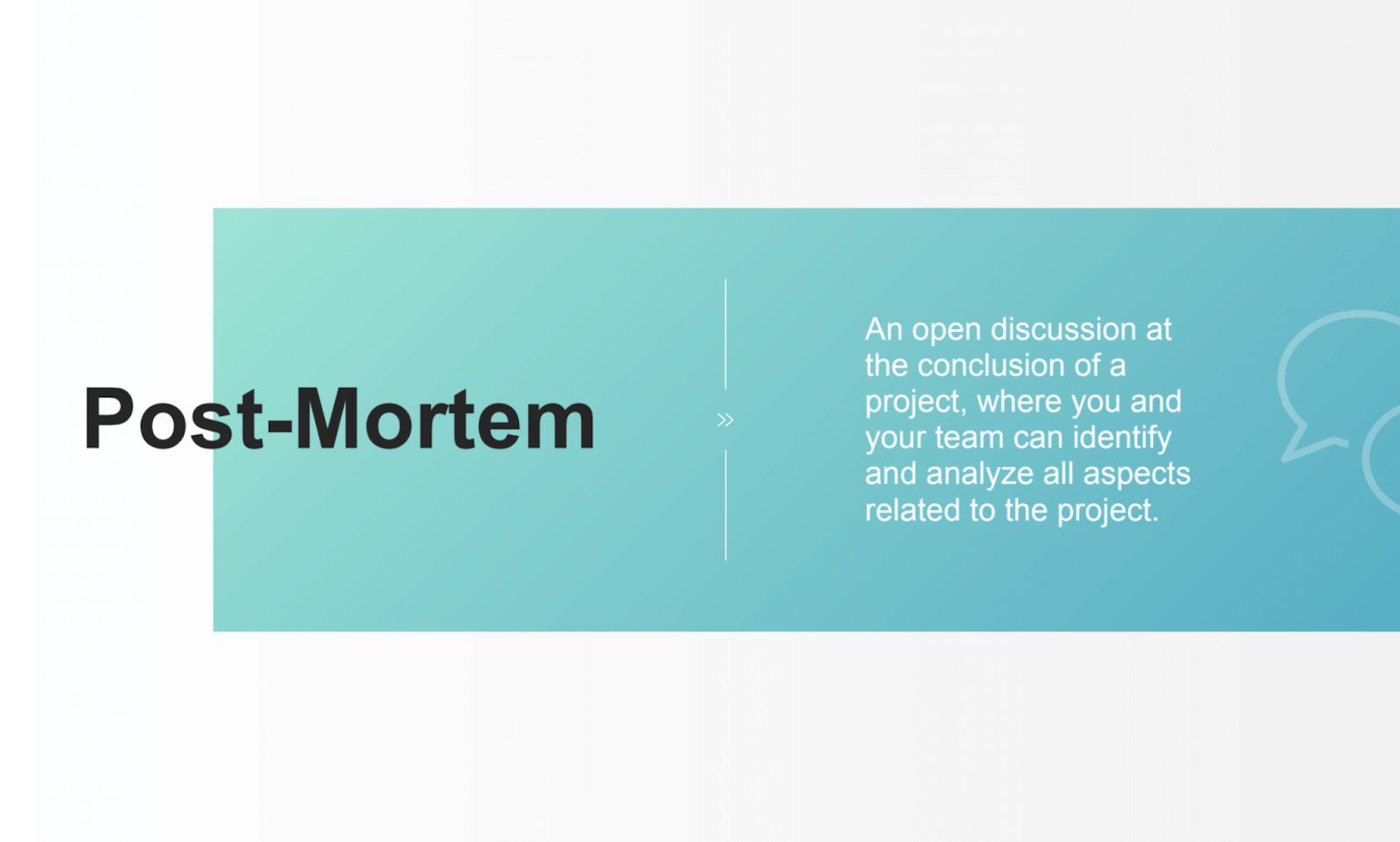
After the person who owns the experiment has done all the write-ups, the analysis, and shared what they learned we have another meeting with the immediate team and we talk about:
- Why did this experiment end up the way it did?
- Why was our hypothesis or prediction right or wrong?
- Why do we think it succeeded or failed? And,
- What are the follow-ups?
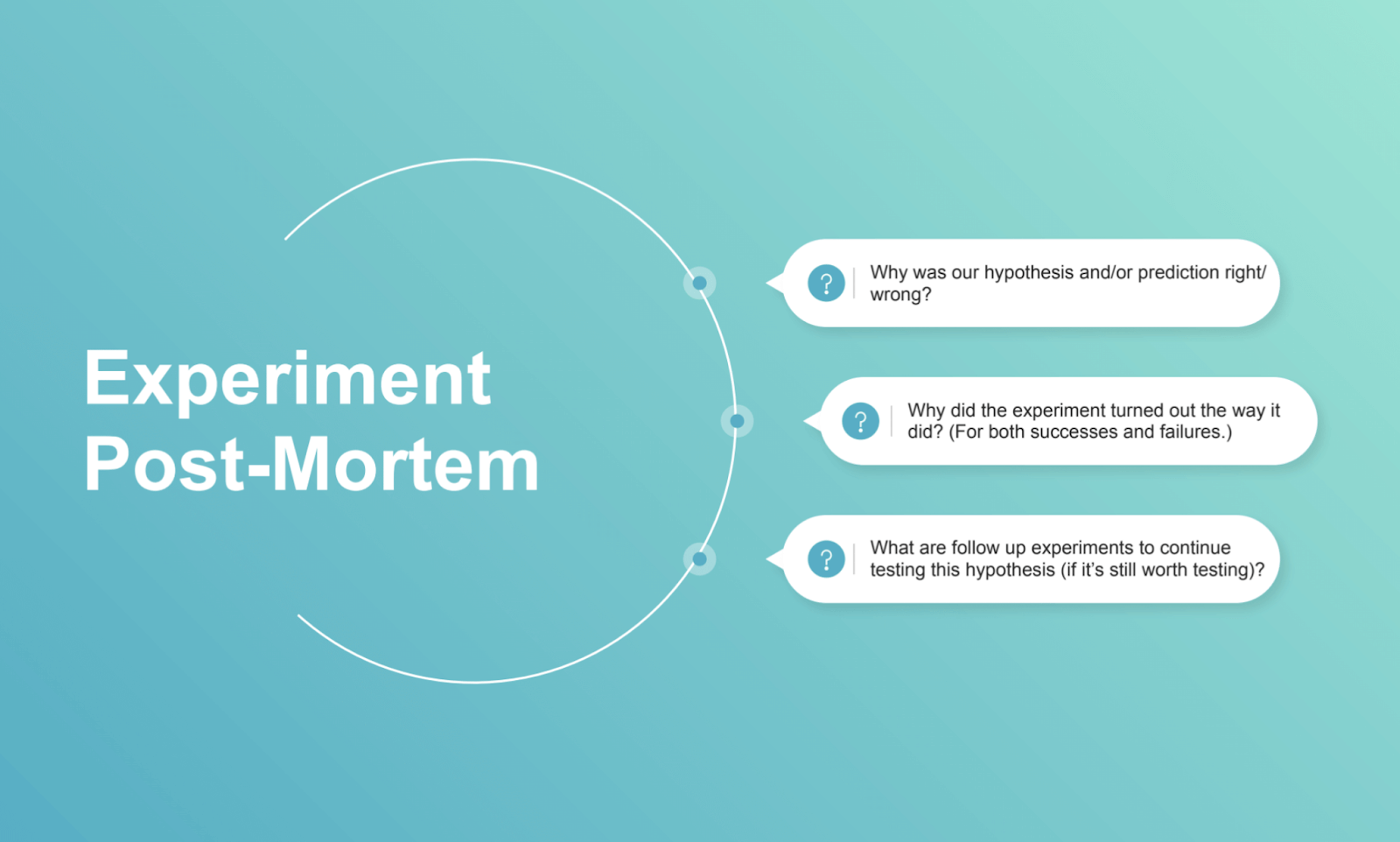
Let’s talk about some experiments
Let's talk about one of the experiments we actually ran. At this point, we've walked through an entire experiment doc of what we did for the social proof experiment.
Hypothesis
Our hypothesis was that people were overwhelmed by questions.
Objective
We had this objective of increasing the number of people signing up for our products.
We decided we want to test social proof of all the ideas that we had.
Prediction
We had a prediction of what we thought the outcome would be.
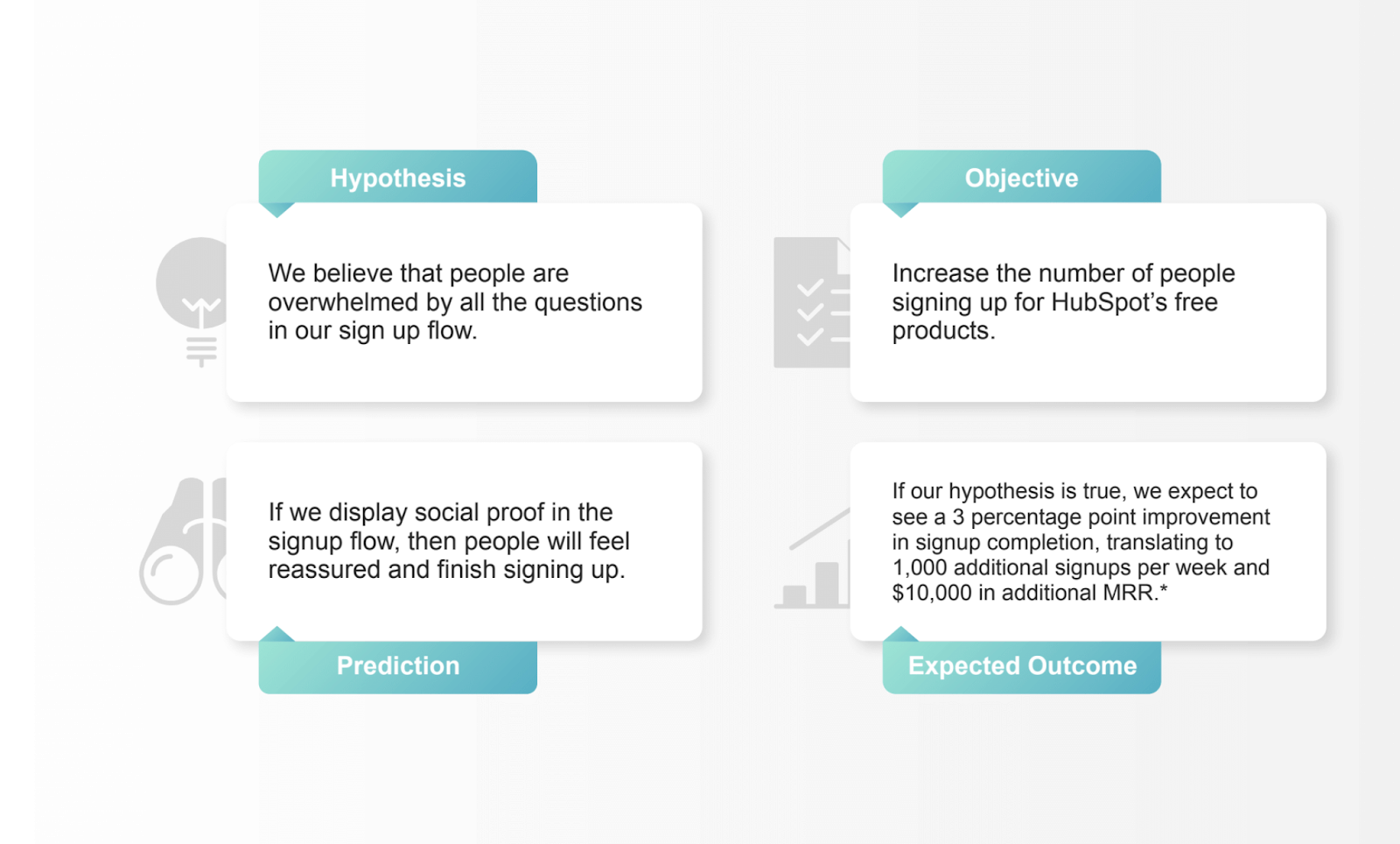
Experiment design
Again, this was the control, we had three variants; testimonials, logos, and awards.
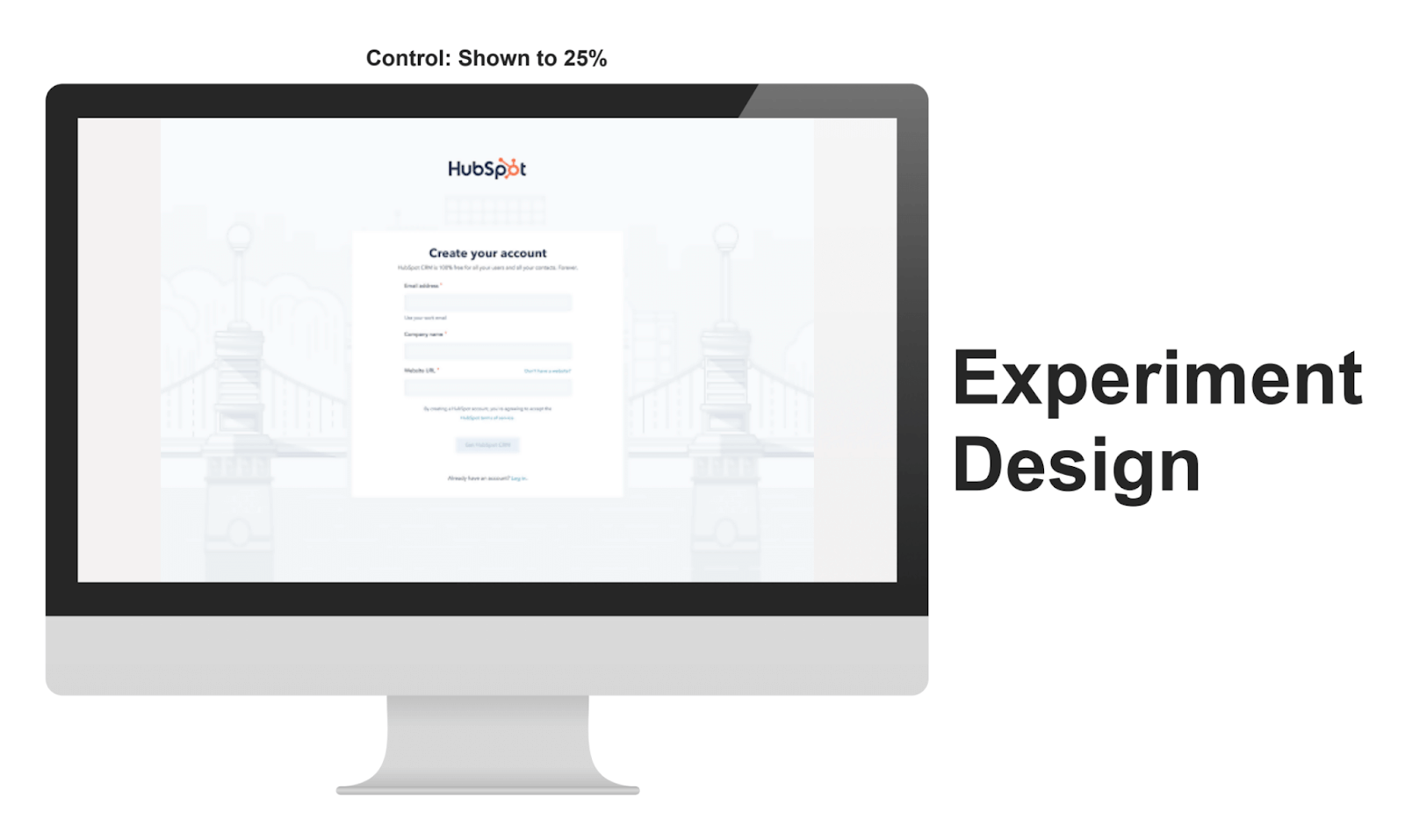
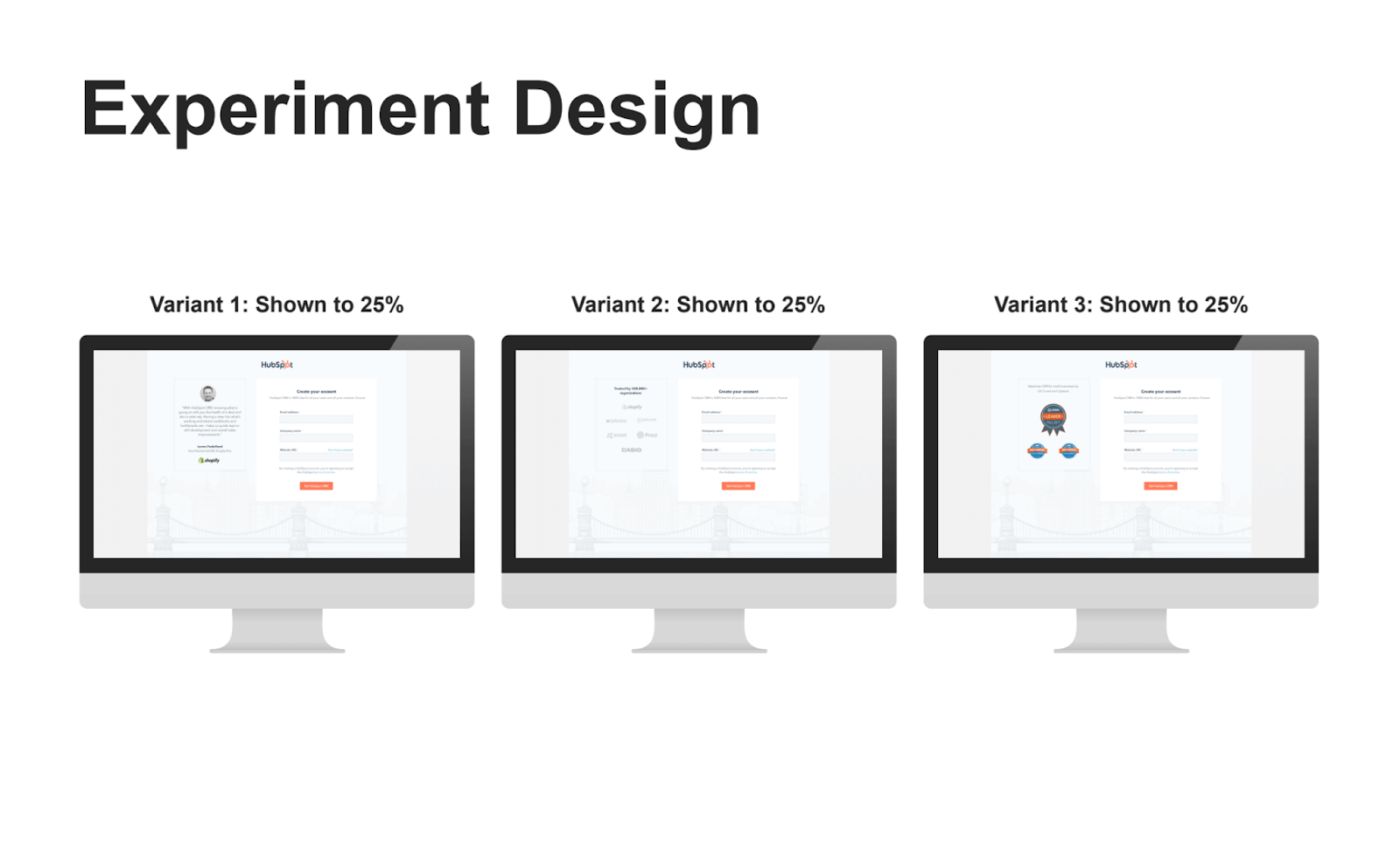
Results
The data was inconclusive, it actually looked like the control which had nothing on it won and as someone with a marketing background, I thought that's weird because social proof is supposed to be one of the strongest things in psychology that influence people to make decisions.
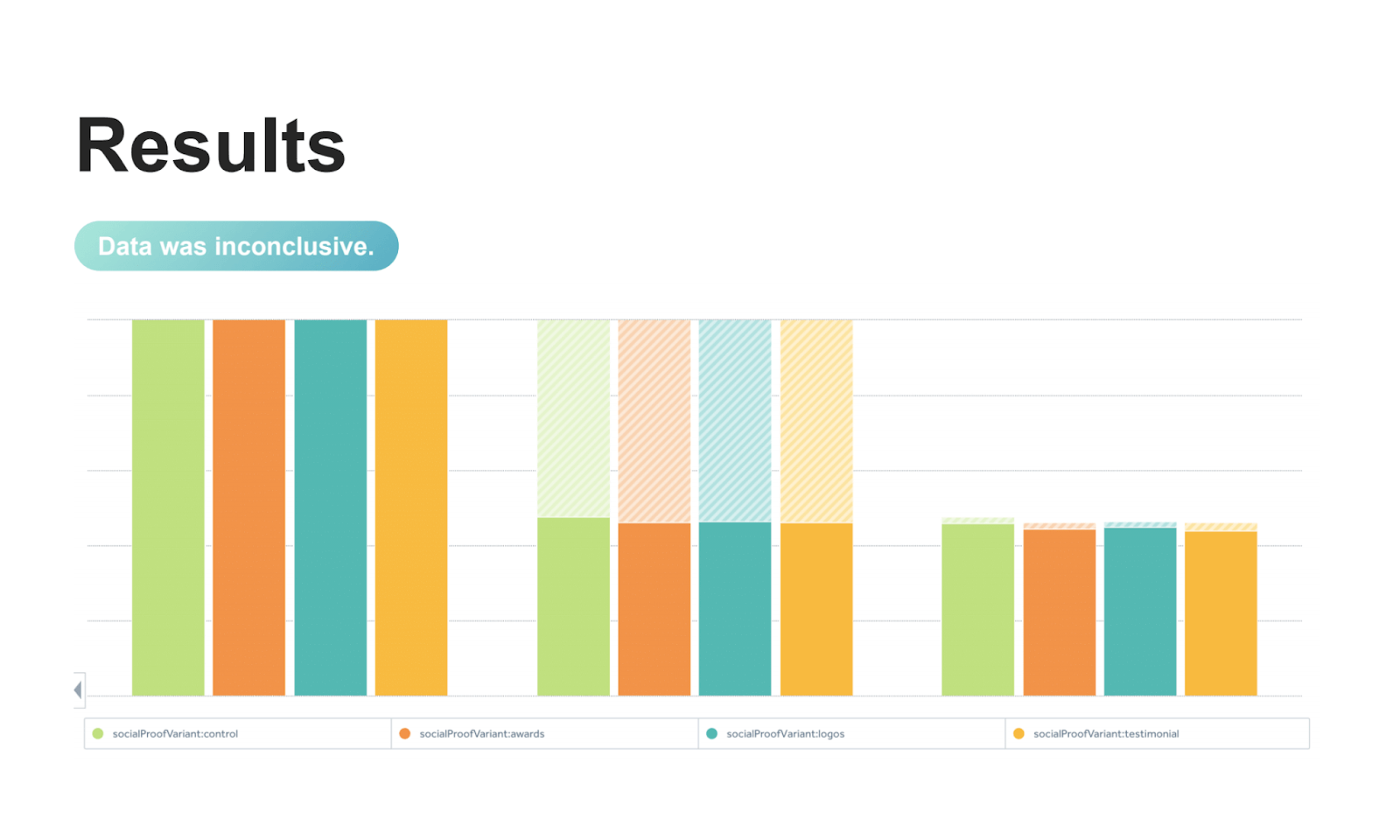
What happened here?
Maybe it was the way we set up, maybe it was a social proof that we showed, but we were really confused by these results.
Learnings
We had to go back to the drawing board but we wrote up our learnings, and we just said social proof and the way we set up this experiment didn't work, we should probably think about how social proof works in different regions.
Maybe if we look at all global traffic, there may have been a net-zero improvement. But maybe in different regions, social proof works differently.
We also asked, maybe there's something else we should be focused on, maybe it's not worth exploring this further.
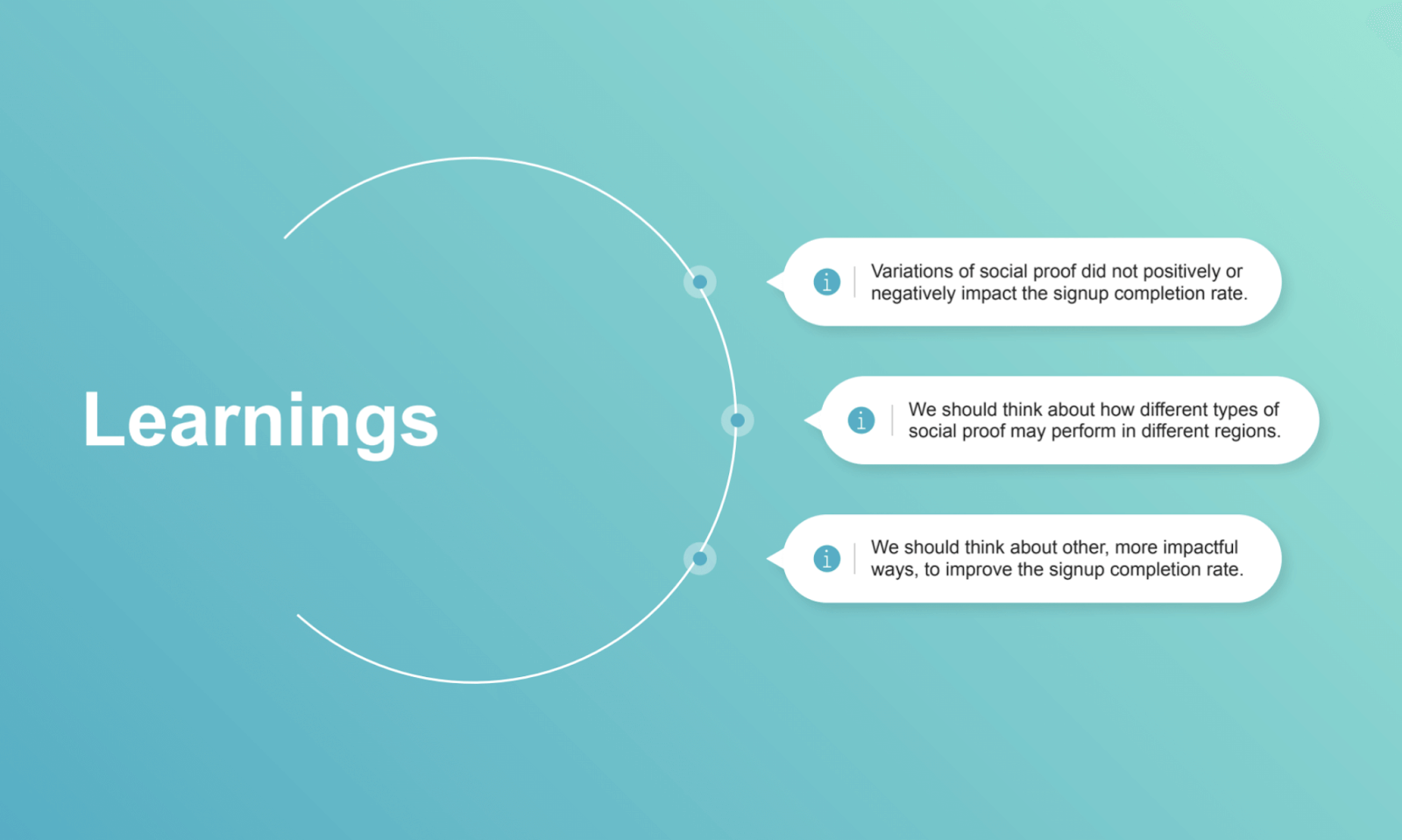
Next steps
We went all the way back to our problem of people are not completing signup flow. Why is that?
We did some digging, not just from a marketing standpoint, but from a tech standpoint, from a positioning standpoint, as well. We learned a few things that were very disappointing.
- We learned that the signup app took five to 10 seconds to load because of tech debt - no one had touched it in years.
- We learned the signup app was a legacy product that was built by two different teams, which meant they had two different backends that didn't talk to each other. In some cases, that second part didn't even load, so people could not finish it if even if they wanted to.
- We also realized that the design does not match the website, if someone goes from your website, clicks Sign Up, and sees a completely different signup page it's really confusing. You wonder if you're getting redirected to a weird page that's trying to hack you or something.
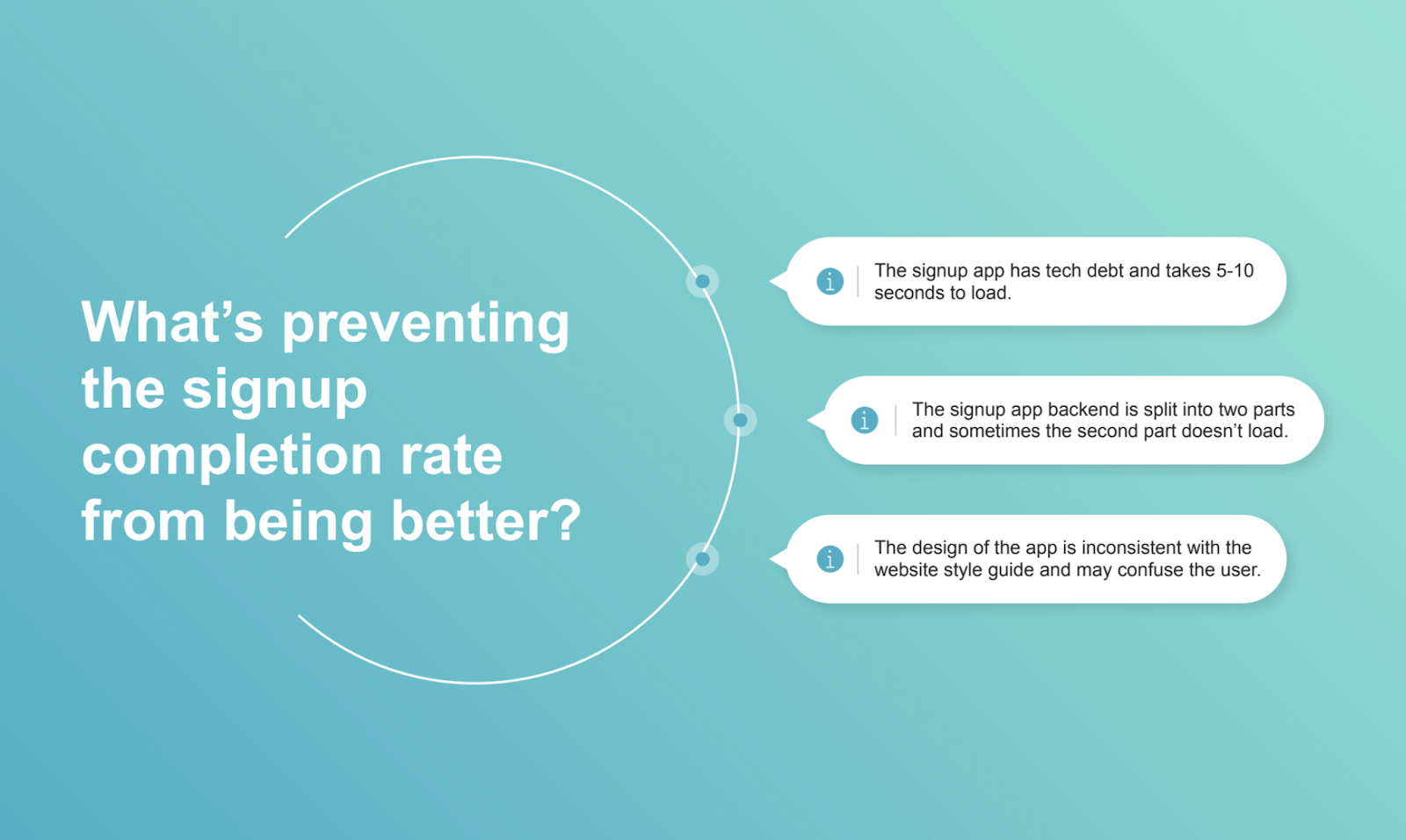
Starting over
We thought let's start over, let's rewrite this document, and run a different experiment. This was a larger design experiment here, it wasn't just adding some social proof, it was actually a very broad change to the signup flow.
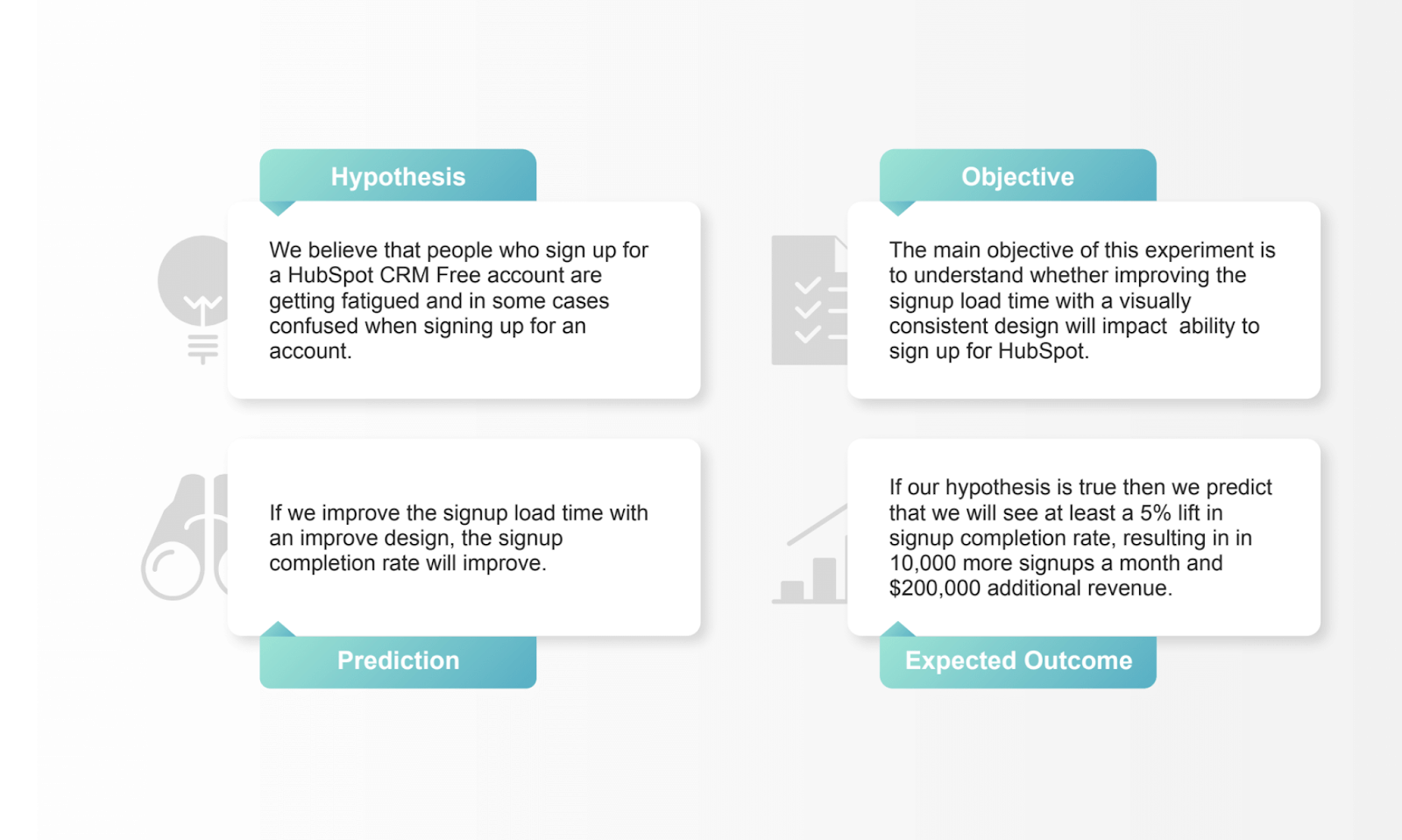
Our hypothesis - people are getting fatigued when they're signing up, there's a lot of confusion and there's a lot of steps and it takes so long to load. People don't want to wait.
Our objective - still to improve signups but this time is to understand if improving load time and making it look better will improve conversions.
The prediction - if we improve load time, of course, more people are going to sign up because the apps actually going to work.
The expected outcome - some sort of revenue.
Experiment design
This is what the signup used to look like.
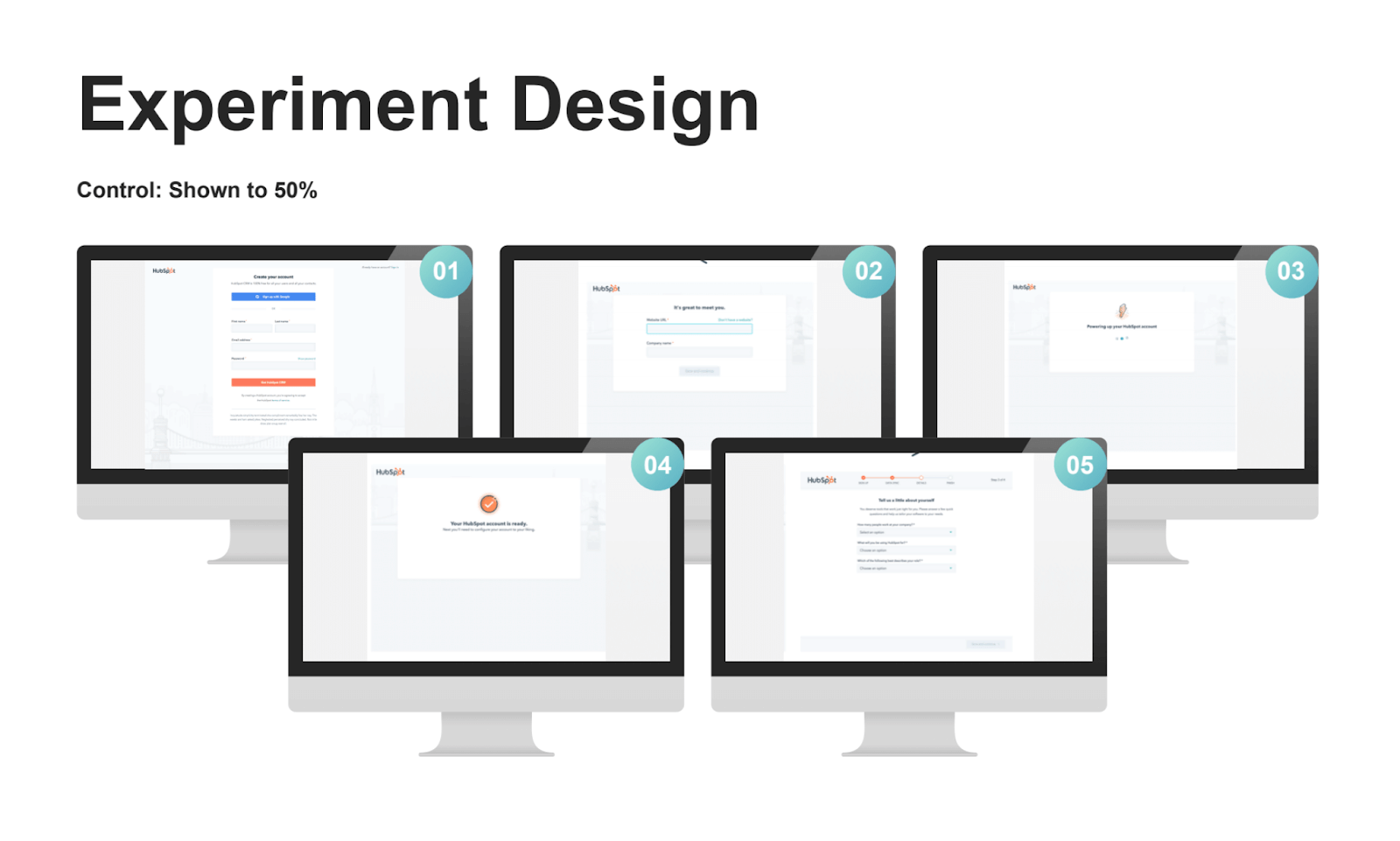
Very minimal, as some people would like to say. This was what the new version looked like.
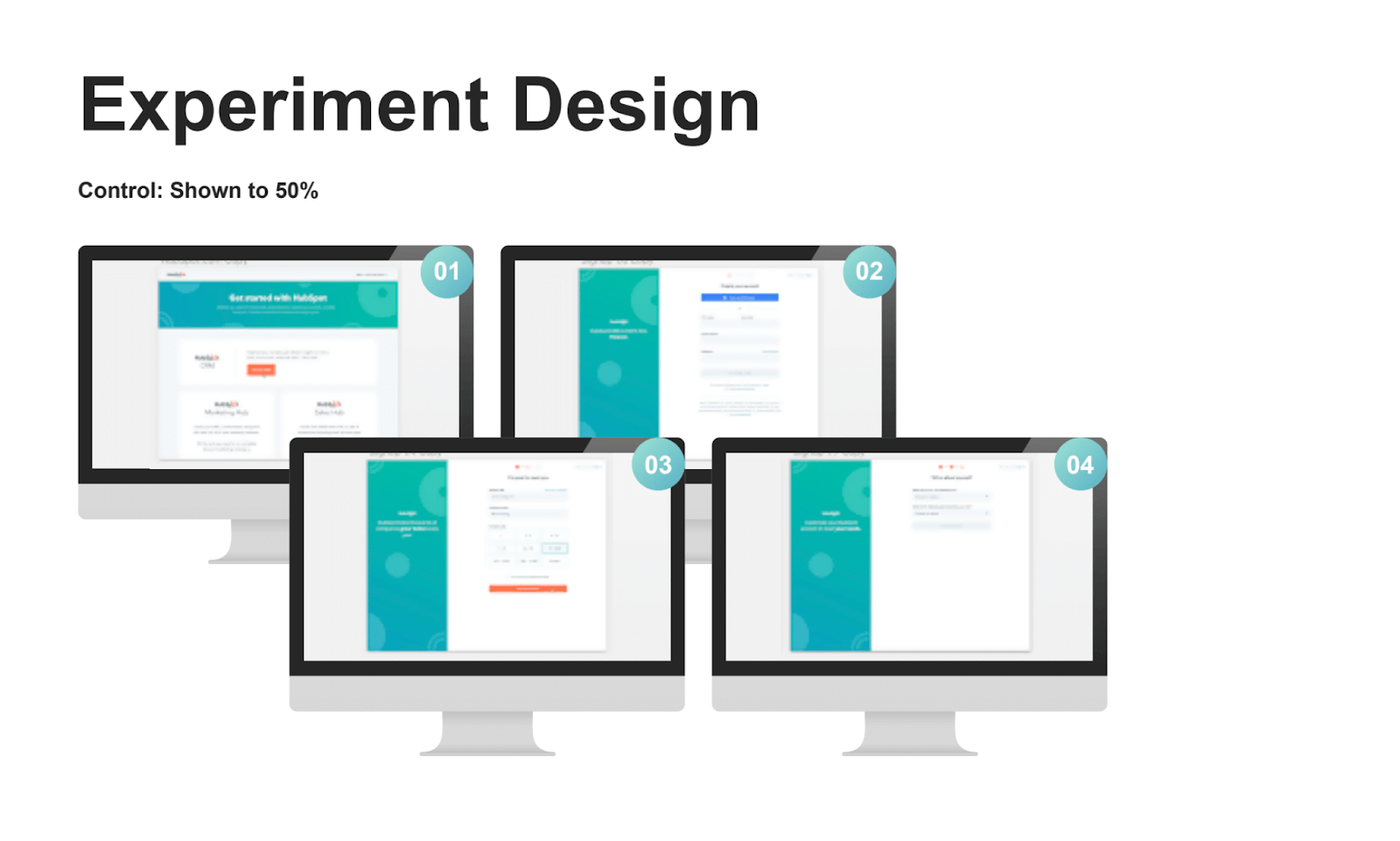
Results
Control
This is what the control performed at…
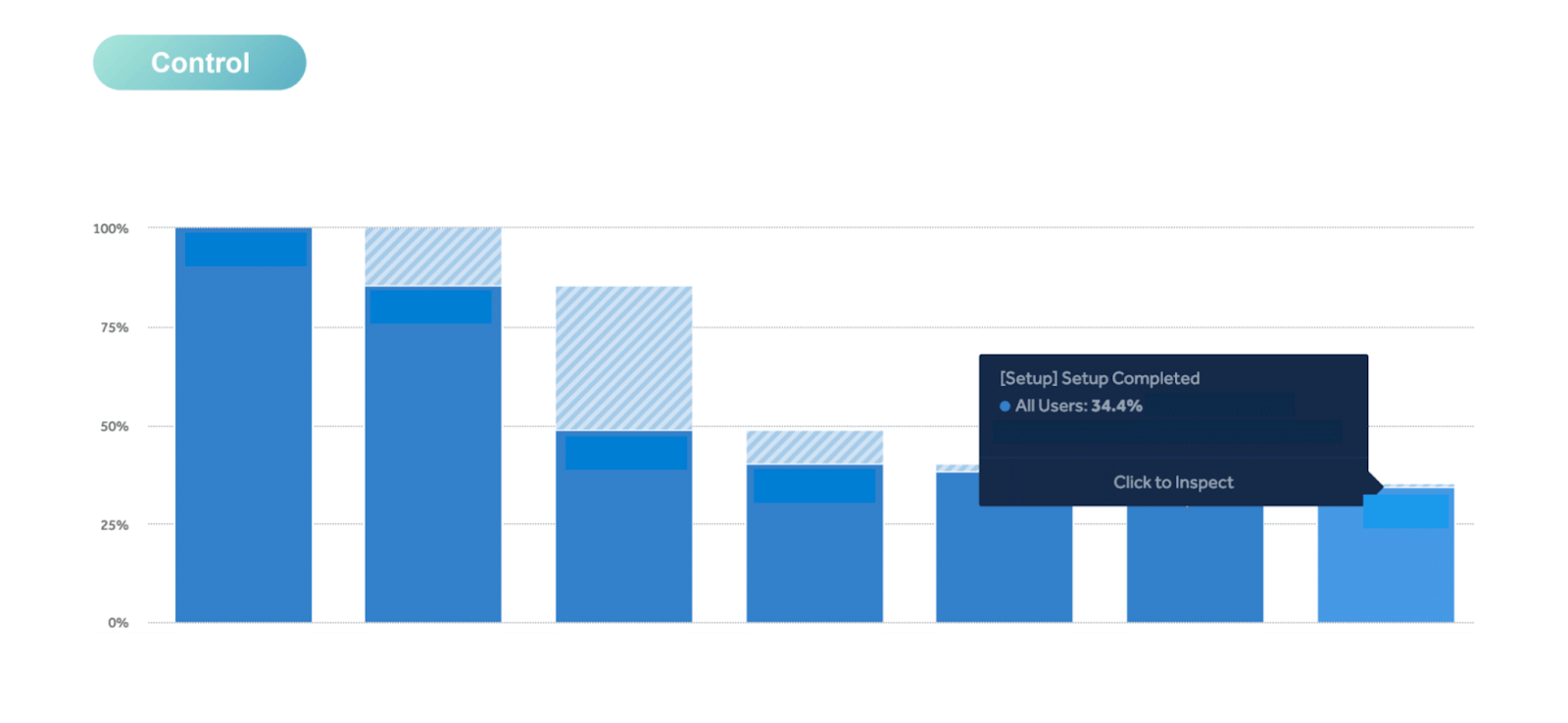
About 34.4% of people completed the signup flow.
Variant
With the variant, we saw a 39% completion rate. It was about a five percentage point improvement.
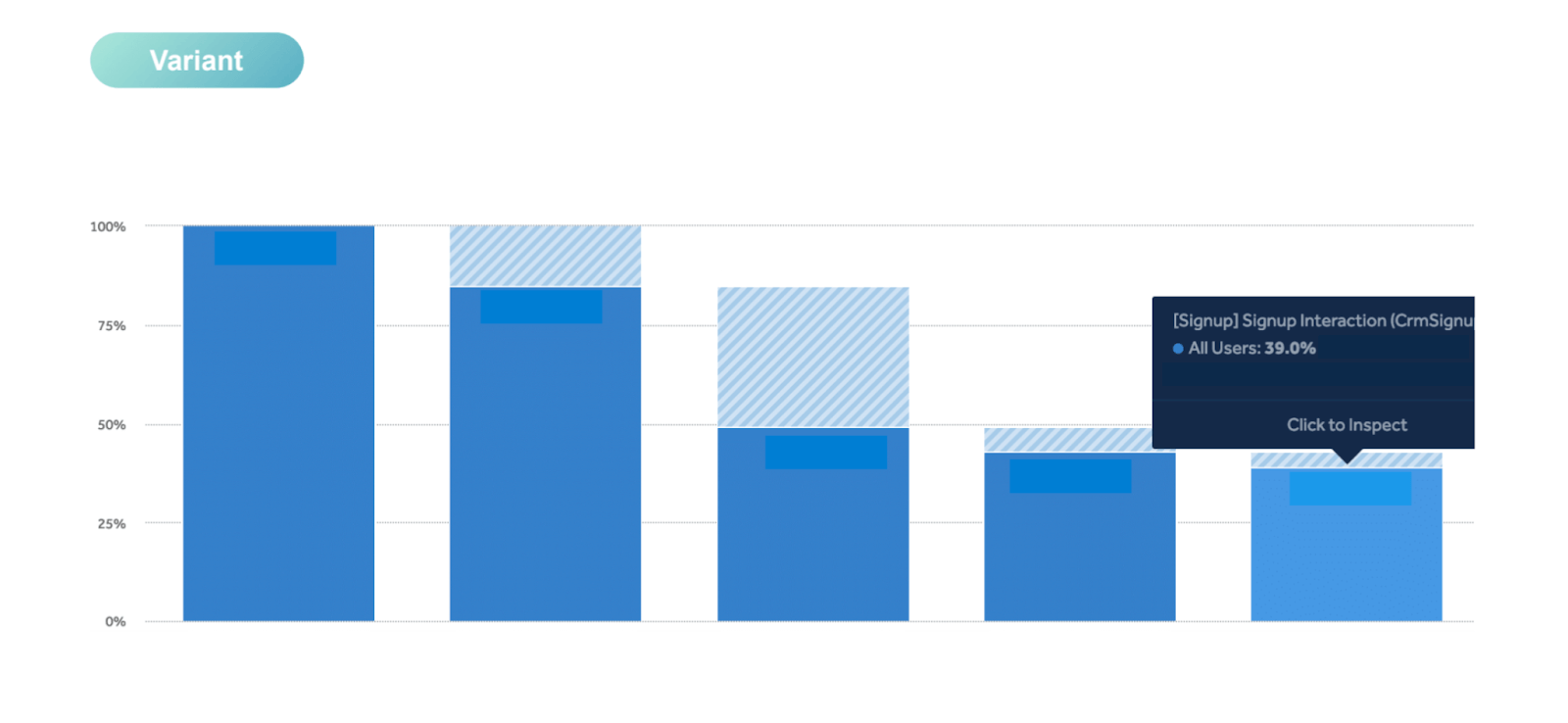
Considering how much traffic the HubSpot website gets and our signup flow gates it's a huge improvement for us. We productized it, we rolled out the new signup flow across all our different products and we saw some good results there.
Learnings
The learning that we still had to write down even though it's obvious was that reducing the load speed, adding visuals, and reducing the friction resulted in more people signing up.
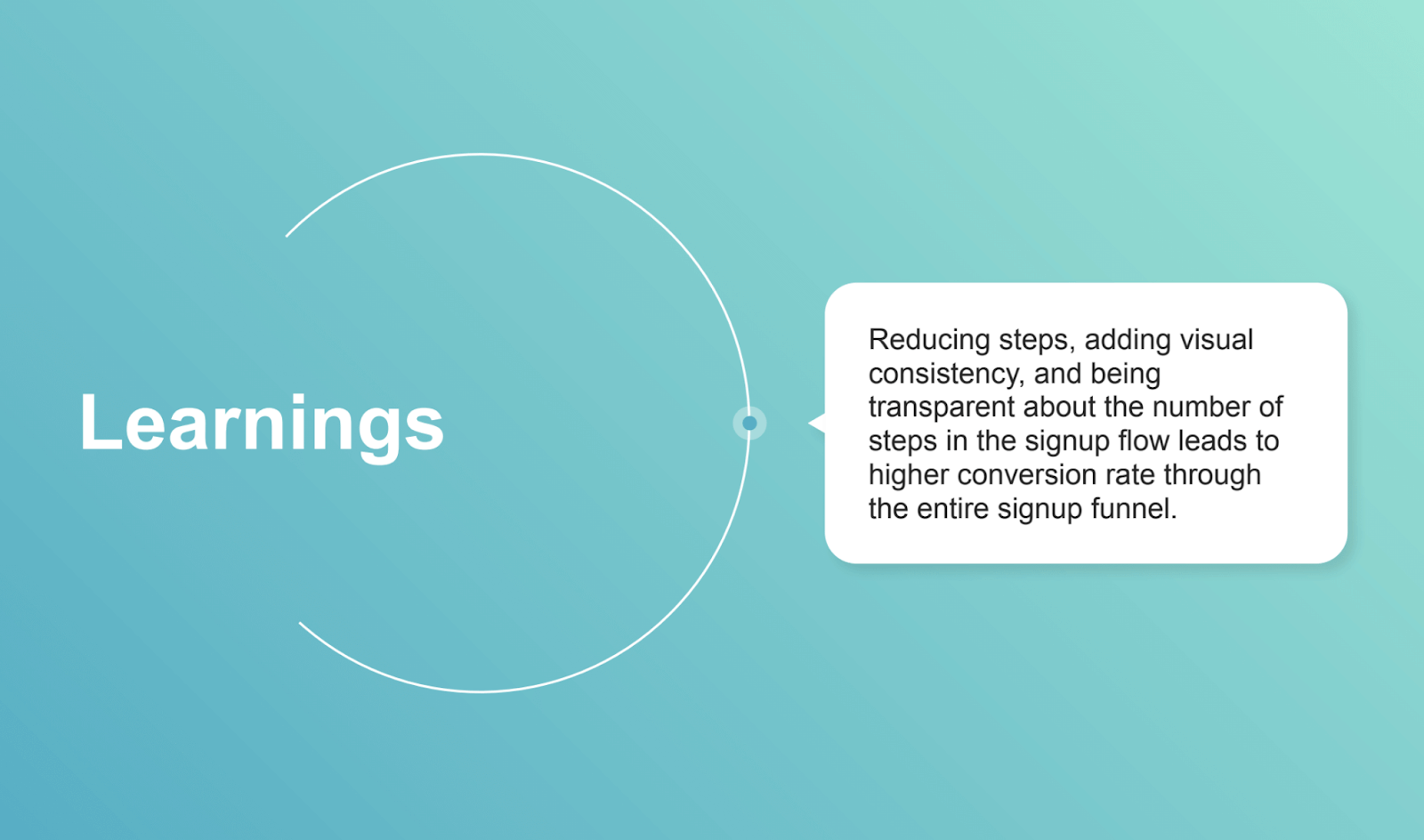
Key takeaways
We improve our experimentation process and refine our filter
I mentioned we have a fine filter for running experiments and we're always working to improve our experimentation process.
I started off with a failed experiment. People don't really like talking about failures but by focusing on what didn't work, you continue to learn and figure out what does work in the future.
In growth, it isn’t about winning or losing… succeeding or failing
At HubSpot, we like to avoid this thing called success theatre, where all people talk about are things that work, which makes it seem like everything's working and people feel shitty when things don't work when that's the reality.
Most things won't work. Having these discussions of things that don't work allows people to feel more comfortable pitching new ideas in the future as well.
The thing that I want to drill into here is, in growth, it's not just about winning or losing or succeeding or failing.
You win or you learn
It's always a focus on either you're winning, or you're learning.
You learn and learn and learn and keep trying new experiments until you win. There's this weird expectation in growth and marketing and product that you have to keep trying a bunch of different things and not thinking about it, you just have to move on to the next thing.
And you grow
When really, you need to slow down to go faster. If you reflect on what you're working on, and continue thinking about why things succeed, or why things fail, continue to learn, that means you continue to grow.
Thank you.