In this article, I’m going to dig into a really important topic, a topic that I'm personally very passionate about: the responsible development of AI products.
I developed an interest in this topic during my undergraduate and graduate years at MIT. I’m now fortunate to engage in this line of work in my day job as a product manager at Microsoft.
In this article, I'll be focusing on the following areas:
- A brief history of ethics in AI
- The need for responsible AI today
- The role of the product manager
- Technology and society
- Bias in AI
- Considerations for sociotechnical systems
- Responsible AI principles
- Responsible AI pipeline
- Responsible AI in practice
But first, a bit of background information on the history of ethics in AI and why it’s so crucial in building products today.
A brief history of ethics in AI
A lot of the crucial developments in AI began in 2012. This year saw the advent of AlexNet, which was the 62 million parameter convolutional neural network.
An experiment called Moral Machines was conducted in 2014 by researchers at the MIT Media Lab. The experiment aimed to crowdsource a human perspective on moral decisions that are being made by machines.
The history of responsible AI usage is only a very recent one, and we have yet to see the application of a lot of these principles and frameworks in real world scenarios.
The aim of this article is not just for you to learn what responsible AI is, but rather to walk away with some level of foundation for asking the right type of questions when developing products and driving product execution.
A quick thought exercise
This is a well-known ethical study: You're in a self-driving car, going down the road at 60 miles per hour.
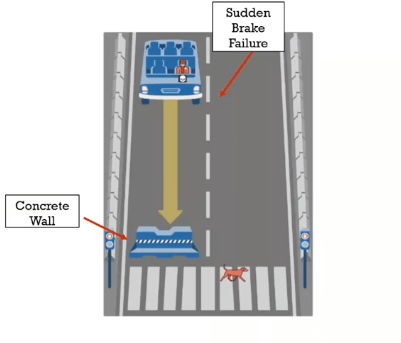
At a distance, you see a huge concrete wall. Suddenly, you notice that your brakes don't work. Now, if you don't do anything, you will unfortunately crash into that concrete wall and not survive.
But you realize that you can intervene, swerving left and driving into that adorable puppy that you see there. Stopping the car will spare your life, but it will do so at the expense of that puppy.
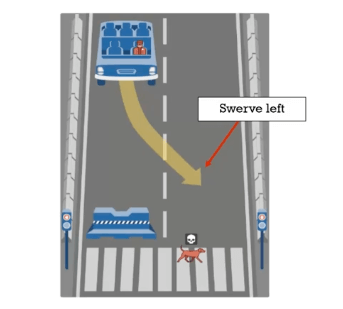
We conducted a poll where we asked, “how should the technology behave? What's the right thing to do? What's the ethical thing to do?”
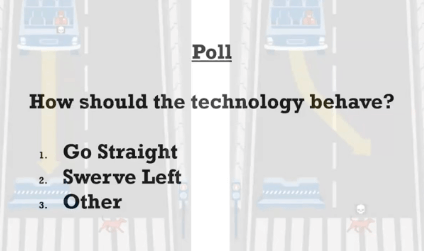
The results were an interesting split:
- 12% said, ‘go straight,’
- 48 % said, ‘swerve left,’
- and 40% said, ‘other.’
Those in the minority may be arguing that it’s not necessarily ethical for us to be actively intervening. Maybe those who argue ‘go straight’ also believe that the car should be programmed to prioritize and protect the life of those in the vehicle.
On the contrary, we also had a pretty decent majority who said that we should swerve left. I'm sure the argument here is something along the lines of, “we should value the life of a human over the life of an animal.”
Finally, we have those who are idealists, who believe that we should try to protect all lives. Of course, this is an ideal scenario, but it may be unrealistic.
The need for responsible AI today
Today, of course, this is no longer an exercise that can exist simply in our thoughts. The reality is, we do have self-driving vehicles, and these ethical decisions have become essential when designing such systems.
Unfortunately, they're not as simple or ideal as the ones that we just explored. So consider this potential scenario where perhaps we might be considering the ultimate utility to society of the individuals involved.
Do you forgo the life of a doctor over an executive? How are you assigning value to human life? And just like that, the scenarios grow exponentially in complexity. These are incredibly complex scenarios that we're leaving to a computer to decide.
As we’ve discussed, self-driving cars are on the road. Whether it be engineers, product managers, or executives, those who are designing these systems are forced to think about and define what a car should be trained to do in these types of scenarios.
But the most crucial questions are:
- Who should be determining the behavior?
- Who's ethical principles are they reflecting?
- Are we taking into account some of the cultural or religious or geopolitical considerations?
Ultimately, when we work towards thinking about some of these considerations, we're working towards some notion of responsible AI.
Transforming the 4th Industrial Revolution
Just like we're seeing with the shift in transportation, with the advent of autonomous vehicles, AI is undoubtedly driving the Fourth Industrial Revolution as we learn to build with it.
This transformation has specifically been enabled by two things: one, a lot of the massive improvements that we're seeing in computing technologies, and two, how this is coming into play with the large amounts of data now available.
We're seeing AI being used to solve critical problems across a wide range of domains. We can see this evidence in the large investments that corporations are making in AI research and development. Below you can see the large growth in AI related patents. 👇
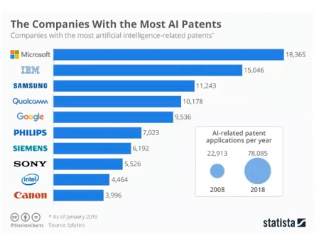
This isn't just for the big players, we also see a lot of startups that are using AI to transform industries such as commerce, robotics, healthcare, and cybersecurity. The list goes on.
The role of the product manager in AI
So, how relevant is the product manager to AI ethics? Well, since the PM is responsible for the success of the product, whether users feel hurt or marginalized by the product is obviously pretty important.
Effective product management
The role of an effective product manager has been well studied and well defined, and you see some of the core competencies and capabilities listed here:
- The ability to work with a cross-functional team of designers, marketers and engineers.
- The ability to understand market insights.
- The ability to highlight a customer’s needs.
- The ability to develop a vision for the product.
And then the job is still not done. There's an iterative process of validating that product testing and then evangelizing the product.
Three principles of product management
the ability to develop a product that sits at the intersection of three essential criteria, as seen below:
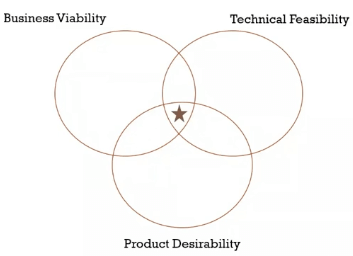
This centre point becomes the North Star for a PM. Once these three criteria are working in unison, that’s when you’re where you need to be.
In AI, we’re now seeing the ability to build even greater products with:
- Greater scale and impact.
- The ability to have better personalization at each individual level.
- The ability to show performance gains.
As this shift continues, the product manager is playing a key role in that journey. Now more than ever, it's important that we exercise some of these responsible development capabilities.
Technology and society
With great power comes great responsibility.
Did you know that no women were on the teams that built the first crash test dummies for cars in the 1970s? I'll repeat that, no women.
As a result, the dummies that were used to test car safety, were built according to the shape and the size and the stature of your average European male. A 2019 study from the University of Virginia found that for female occupants, the odds of being seriously injured in a frontal crash, were 73% greater than for a male occupant. The takeaway here is that bias in technology is not new.
Now more than ever, responsible development of technology is important, and it really requires a systems-level perspective and approach.
Clearly, when testing crash dummies, they were considering a user, but not considering some of the responsible AI principles of fairness and inclusiveness.
Case studies
I think it would be valuable to explore some progressive AI case studies. What you can see below is a 2018 study from the MIT Media Lab, which showed that commercial face recognition technologies from companies of the likes of Amazon, IBM, Microsoft, were shown to have gender and skin bias.
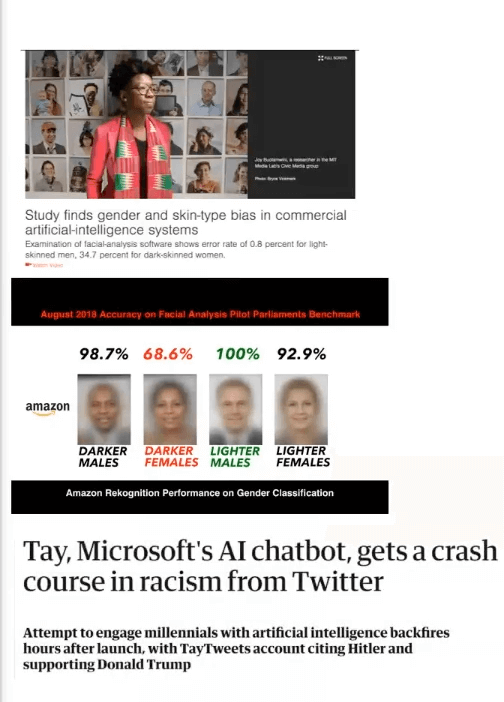
The face analysis software showed higher error rates for dark skinned women than it did for light skinned men. When you think about the ramifications of this, considering the applications to healthcare, there's serious cause for concern.
We've also seen interesting behavior where a chatbot released by Microsoft experienced data drift and ultimately became, racist, homophobic and sexist. This was because of the degradation in the actual AI models that were powering the chatbot as it continued to interact and engage with society and real people.
We see social media platforms like Facebook, and the recent Cambridge analytical scandal. These incidents have been shown to have serious repercussions on society and political stability.
In the legal domain, we have AI system tools that are being used to help with sentencing. We've seen that most of these tools show disproportionate biases against people of color. On the right of the image below, you’ll see that a white male with more past defences will be deemed lower risk than a black male with fewer prior offences.
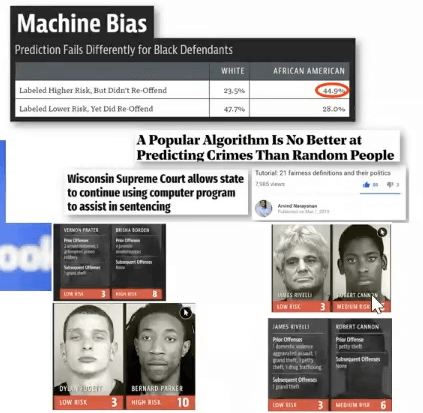
This is the prime example that we see, where previous data is simply reflecting institutional or discriminatory practices that existed in society. We also have the canonical example of self- driving cars, and the concern around safety.
In the financial sector, AI can play a very critical and concerning role around denying consequential services to everyday people.
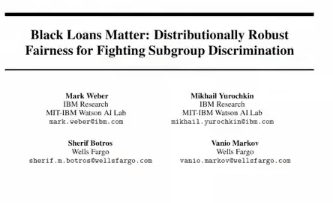
Also, with daily products that we use, such as language translation systems, we see biases in how certain roles such as ‘doctor’ are automatically mapped to male, whereas certain roles, like ‘nurse,’ are mapped to female.
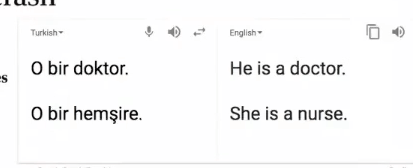
All of this doesn't even touch on some of the business impacts of AI and automation. Let’s touch on how certain blue-collar jobs are being automated away to technology and AI. There's also the concern around the role that AI plays as a gatekeeper to the jobs that are still available.
We’ve seen Amazon scrapping the tool for recruiting because of biases that it showed against women. This was due to inherent biases in the training data that was used to develop the system.
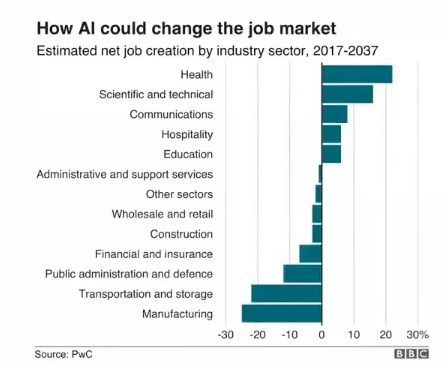
Lastly, we have privacy concerns coming out of China, where we see surveillance being actively used in contact tracing.
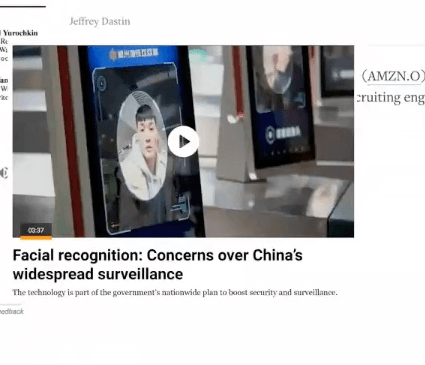
Bias in AI
So, what can we do? How can we actually work towards mitigating some of these scary consequences? It's important to start off by identifying the different types of bias that exist in technology. These are defined in the literature in three broad categories.
Pre-existing bias
This really finds its roots in social institutions and practices. It reflects the organization that's responsible for determining the requirements, or the specification for the product.
We previously saw how an AI sentencing tool reflected the biases of society. We saw how they became an outlet for the inherent institutional biases that previously existed, which perpetuated into the actual tool or system that was being developed.
Technical bias
Technical bias arises from technical constraints. The prime example here is self-driving cars. You may have a limitation on the number of cameras and sensors that you can have. This may ultimately result in accidents or casualties resulting from these limitations.
Emergent bias
This is arguably the most concerning and most difficult to deal with. It’s the result of a deployed system going out into the wild and learning on its own.
Oftentimes, this results in a ‘data drift,’ meaning new data coming in that’s not actively monitored is resulting in degradation of the model that's powering the core product or service. We call this ‘data drift.’
A prime example here is ChatBot. It was released for the function of being a conversational Chatbot, but as it continued to engage, the new data that was coming in was ultimately shifting the core function of Chatbot.
Considerations for sociotechnical systems
Keeping in mind the different types of biases, it's important to think about how they play a role in sensitive-use cases. Sensitive-uses can be thought of as having a broad impact on people's lives, resulting from some AI powered decision or recommendation system. As product managers, it’s helpful to break this down into the three levels you see below.
The individual level
This really encompasses thinking about the types of physical harms and safety concerns. Also, with the example of self-driving cars or health care, you can think about some of the concerns it poses to the legal or financial sector.
But how does it ultimately affect financial health or ensure equity and fair treatment? At an organizational level, you can think about the impact on financial performance. You see AI being used for automating financial processes such as algorithmic trading.
Organization level
It's important to think about both the financial and non-financial performance impacts at an organizational level. You also have reputational integrity concerns as it relates to the use of data, potential breaches of privacy.
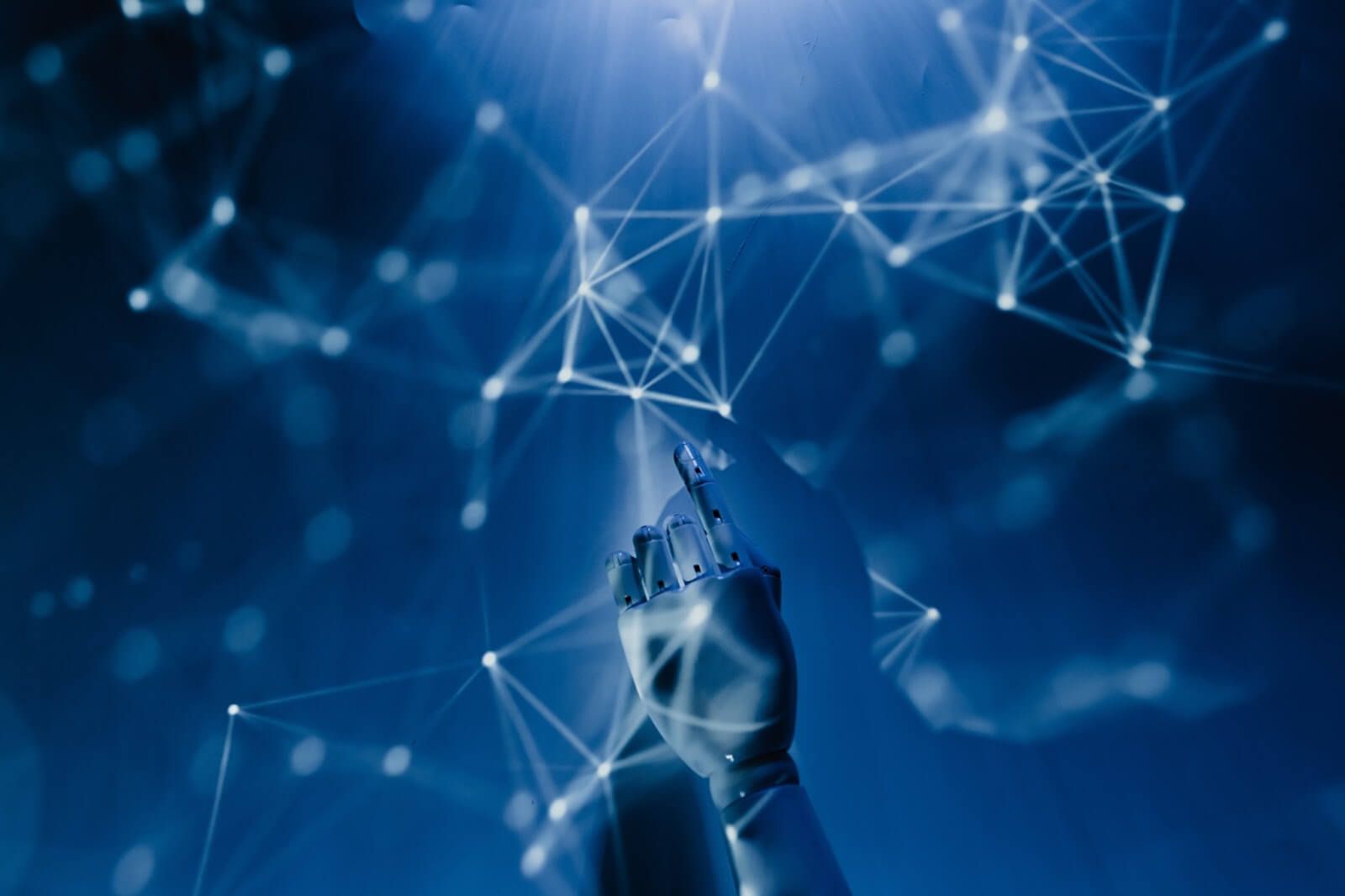
Societal level
Consider the role of social media. The role that these platforms can play in actually destabilizing economies and governments, how they pose a concern to the primary functioning of a country's democratic system.
We haven't even touched upon AI power technologies, such as advanced weaponry and drones, that have really redefined how we're thinking about national security. When developing and deploying products, it can be really helpful for a product manager to weigh up these three levels.
Building with AI: Responsible principles
So, what are some of the principles we can implement? There are more than 300 responsible AI principles, frameworks and regulatory initiatives.
This list is intended to capture some of the salient and most important value-based principles. These are principles that you can implement into the actual design and development of the products and services that you're building as the product manager.
Ideally, when you contend with these principles, you achieve more responsible AI.
Ethics
On the ethical front, we need to be able to distinguish between right and wrong. We need to think about whose ethical principles are being reflected, and consider the broader cultural and geopolitical factors when making these decisions.
Let’s look at the example of self-driving cars again, a decision is being programmed into a machine. This isn't an objective decision, and they must reflect the right ethical and moral values.
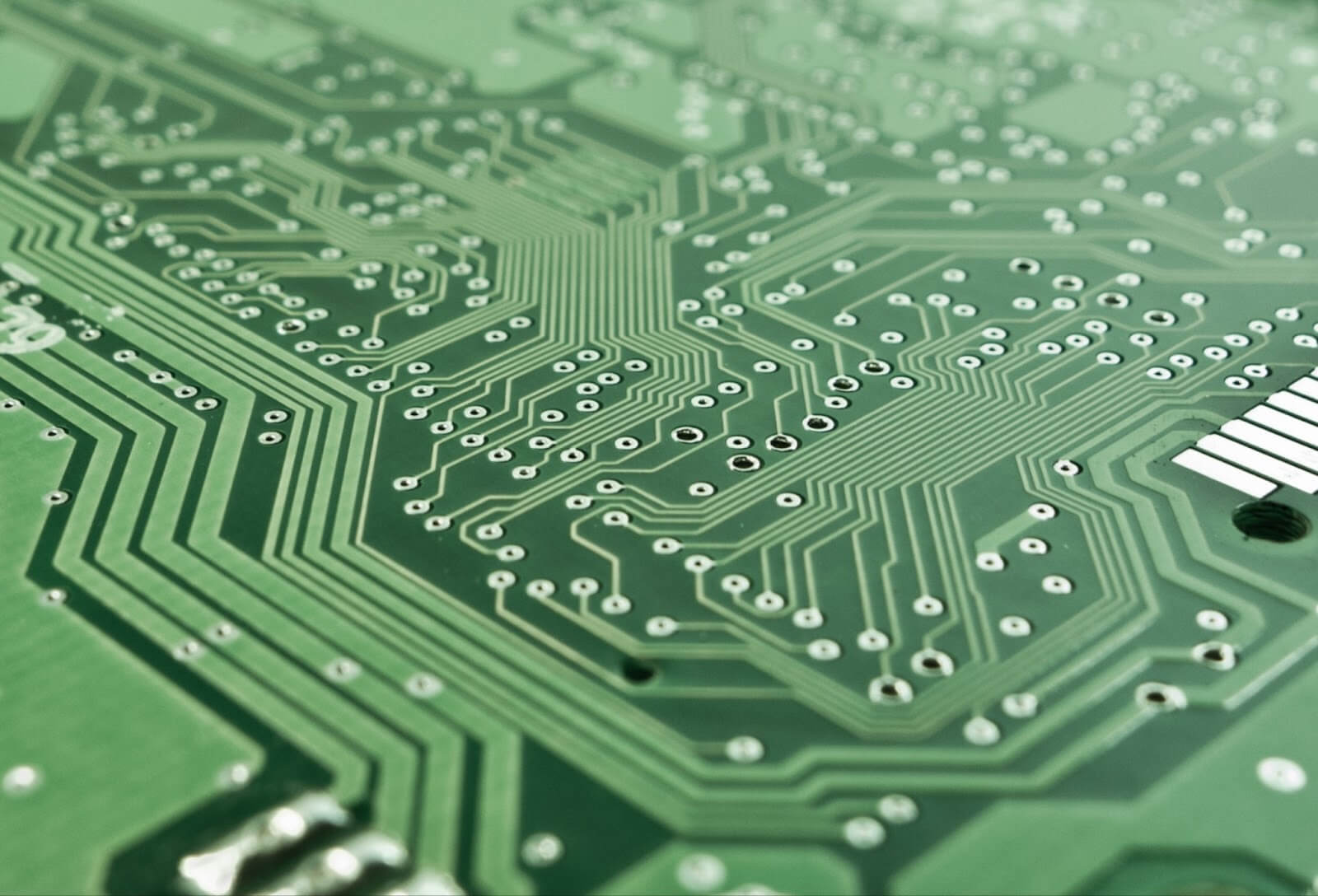
Fairness
Fairness is a very interesting and widely discussed topic. There are different notions of fairness, but are you thinking about the subsequent impact on an individual level? Now, there are many definitions of fairness, much like there are many ways to interpret ethics.
You can work towards fairness by equalizing accuracy rates across different groups that you have. Or, you can equalize error rates, false positives and negatives. Again, there are many different ways to define fairness, which makes the task of having a standard on fair AI systems difficult. It’s also very context-dependent.
Inclusiveness
We’re talking about inclusiveness in the design of AI technology, but also the output in the decisions that it's making.
Going back to the crash-test dummy example, we saw a prime example of how not including women on the team was responsible for developing this. This resulted in the product not being inclusive for 50% of the world population, and for disproportionately injuring or impacting those that are in that sub-population.
Transparency and explainability
This is about thinking of AI as more than a black box in which you simply input information. It’s about applying conscience to the technology and understanding why a decision was made.
Accountability
Who owns responsibility over the actions of an AI? On the positive side, if an AI makes an amazing piece of art, who has the IP? On a more meta level, do robots have human rights?
When things become progressive, how do we think about the self-driving car killing pedestrians? Who was held accountable? Is it the data scientist? Is it the engineer who designed the model? Is it the product manager? It's really important to consider who is held accountable for any actions.
Reliability and safety
How robust is the system? How susceptible are you to data drift? The Chatbot that went wild wasn't robust. Its design resulted in model degradation that resulted in unforeseen and harmful applications.
Privacy and security
Lastly, we have the privacy and security aspect. You need to think about data governance, and most importantly, ensure that you're complying with privacy standards that exist across the world.
Arguably, the most important one is the general data protection regulation, which is a framework for guidelines on the collection and processing of personal info for the EU.
Responsible AI pipeline
We talked about the different types of biases, we talked about sensitive uses on the individual, organizational and societal level. Next, we want to explore the key responsible AI principles to consider as a product manager. But how can you actually apply these in the context of AI product development and deployment?
To do that, you must consider the different stages of the AI design pipeline, from concept exploration to the actual deployment stage.
Design
Starting off in the design stage, it's important that you really demonstrate the ability to articulate the value proposition that an AI driven feature will have. A question that you should be asking is, should this problem be approached as an ML task?
If so, are there potentially other methods for solving this problem? If not, what are some of the socio-technical implications and potential types of harms? We need to remain thoughtful about transparency, interpretability, ethics and fairness.
Data collection
Next, you get into the data collection stage. It's important to consider, is the data available? If not, is it representative of the real world?
Do I have enough data for the required model? Is my data clean or noisy? How do these different types of limitations or constraints ultimately influence the model's behavior? This is also an important stage to consider some of the broader privacy concerns.
Model deployment
You really have to consider the transparency of your model.
Beyond that, metrics are an important part of the job of a product manager. Are you measuring success by:
- The accuracy of your model?
- The fairness of your model?
- Do you understand the trade offs you're making across different metrics?
Here’s a potential crucial trade off: Your model may be more accurate, but proportionately unfair to a wider demographic. Or, your model might be less accurate, but proportionately more equitable.
Deployment
But wait, the job’s not done yet. Just because you finished training your model doesn't mean you're done for the day. During deployment, you really need to think about how the system is interacting with people and society at large. You need to ensure that you're monitoring your model and ensuring the upkeep of the model performance. You don’t want to be falling victim to data drift and model degradation.
This is a shift in how you approach product. It’s a broader ongoing process that really requires some introspection.
Responsible AI in practice
The takeaway question is, what are the things you can do within your organization? What are the technical and tactical measures you can take?
Here’s a quick summary of some of the things you can do:
- Establish diverse teams.
- Ensure inclusiveness of different subject matter expertise.
- Strategize implementation of the principles throughout the AI development lifecycle.
- Consider some of the socio-technical context of your AI product or service.
From a technical point of view, there are also measures that you can take:
- Ensuring representative data sets.
- Ensuring that your data sets are documented and your models are documented.
- Defining whatever your performance metrics are, accurately considering the most appropriate trade-offs.
- Stress testing your system.
- Actively monitoring the deployed system to prevent any degradation in model performance.
There are a lot of important considerations and questions to ask. Now more than ever, we are seeing some of the real and concerning consequences of these technologies on our lives.
Within the world of product managing, we really have folks that are responsibly driving product development. 💪 They are implementing these principles from the start to ensure that we're able to achieve more responsible AI.
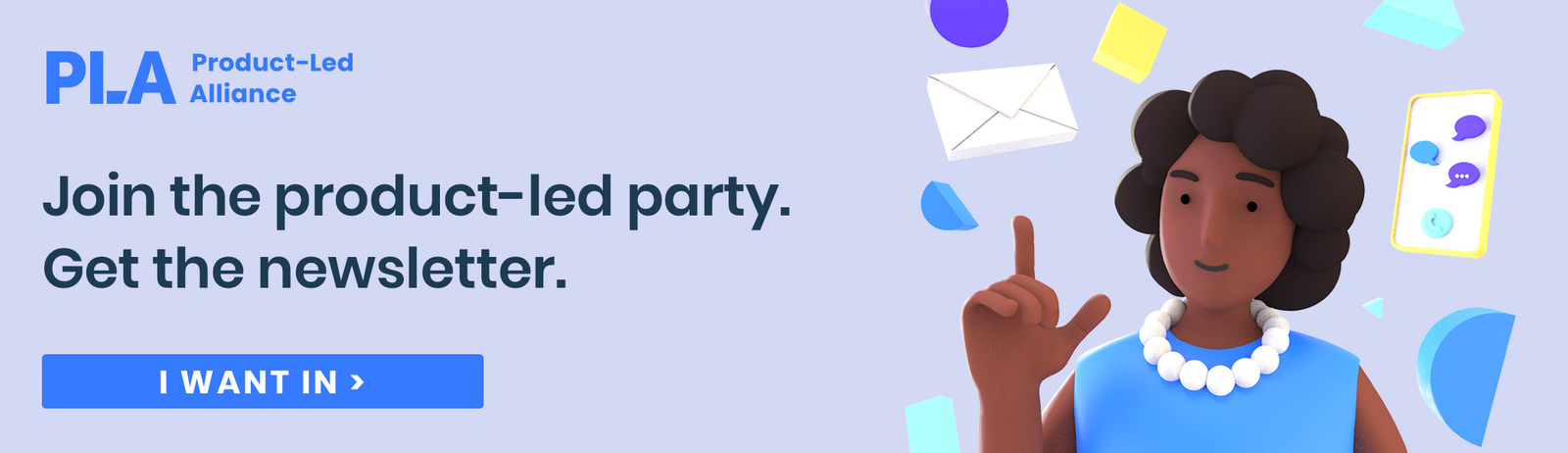