David Ly Khim is Growth Product Manager at HubSpot. This article is adapted from his talk at Product-Led Summit, London, 2019.
"We're going to talk about growth strategy.
Imagine. You’re looking at a chart. It might be traffic, it might be leads you're generating. Whatever it is, you want it to be going up. But, it's looking depressingly flat.
You're wondering, what's happening here? I need to do something. I might get fired. My company's not going to succeed if this continues
Last week you read some blog posts that said you needed to run some experiments. And you're thinking, 'I've got to start somewhere. There's this button on my website. I want people to sign up. What if I made that red?'
So you run it. And a couple of weeks later, your graph still looks like a pancake.
A lot of those blog posts said, '90% of what you’ll do is going to fail. So, you just need to keep pushing on until you succeed.'
You keep running experiments. You're still getting that same chart week after week and you start to wonder, 'Maybe these experiments are the wrong experiments.'
With experimentation, people tend to focus on the tactics and solutions before really understanding what the problem is.
What are you trying to solve by changing that button color? The problem is not the button color. It’s the fact that people aren't converting not your website.
We always need to start with problems. Maybe you get a lot of traffic but no one's signing up or becoming a lead. You might get people who sign up for your product, but they never end up using it. You might get people that will use your product, but they don't pay you. These are all problems and when you start with them, that opens up a world of different solutions.
We're going to go through why you should run experiments, how to best run them, our obsession over documentation and how they help with communication. We're also going to talk about some real experiments that we ran at Hubspot."
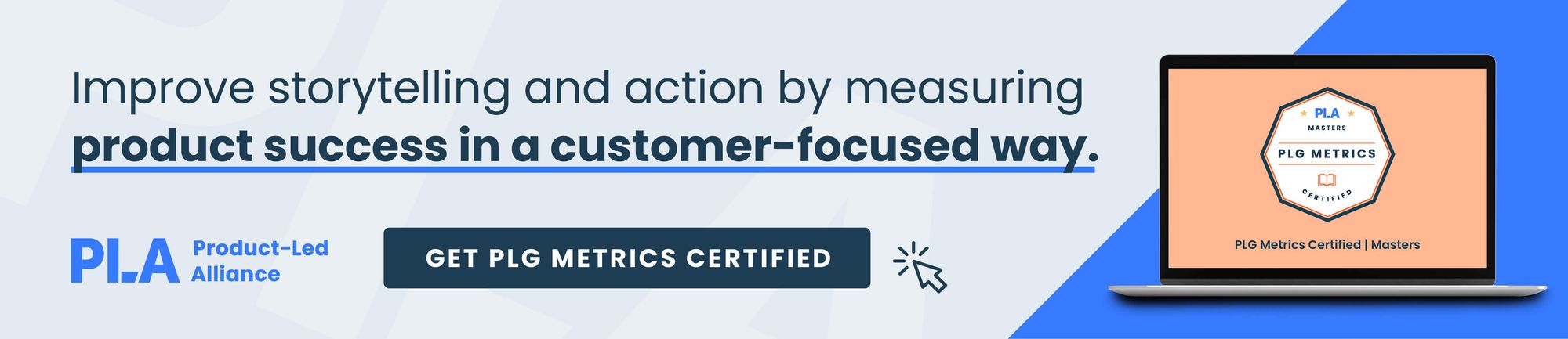
Growth Tactics
"The challenge with growth tactics is that anyone can do them. Change that button from green to red. If you think about any sort of tactic, they go through a life cycle from when someone discovers it, everyone starts doing it, and then when it eventually stops working.
Think Email marketing. Back in the early 2000s. Email marketing was probably getting 60-70% open rates. But, now you have enormous email inboxes with 10,000 unread emails, no one wants to open these emails anymore. You get lucky if you get a 20% open rate.
Back when pop-up forms first started, they were hailed as having a 70% conversion rate. I don't know about you, but the moment I see a pop-up starting to appear, I look for the X button immediately. Some websites even resort to cheating tactics where they hide the X button and then make it white on a white background. These are silly tactics that provide a bad user experience.
So, how do you avoid this lifecycle of growth tactics and instead do things that help users? It's about having a process and strategy for why you're doing those experiments in the first place. What makes us think our user is going to click a red button versus a green button?"
Documentation is King
"To avoid growth hacking at HubSpot, we focus very strongly on documentation. That's how we create a really fine filter for high-quality experiments. You're going to run a lot of things that don't work, but you're going to have a few experiments that do succeed.
There's going to be a certain point where you say, 'Hey, we ran into something like this six months ago, it didn't work, what makes you think it's worth running this time?' You can continue filtering out things that might not work and focus on it things that will.
Let's run through all the documentation that we've completed at HubSpot during an experiment."
9 Steps to a Great Experiment
1) The Hypothesis
"Every experiment should start with a hypothesis. The mistake that people tend to make with the hypothesis is they say it's a prediction. 'If I do x, then y will happen.' That's a prediction. A hypothesis is just a statement of what you believe to be true about your users or customers. It serves as a guardrail for brainstorming experiment ideas.
Our hypothesis highlighted that people are overwhelmed by all the questions in our signup flow. We asked a lot of questions. We realized we needed to change that."
2) The Idea Brainstorm
"Someone starts at the homepage at HubSpot. They click to get started. They learn about our products. They decide to sign up. That first screen is asking for their name, email password. Then we ask for information about their website, for some more information about their role. And finally, they get into their product.
You can begin brainstorming ideas and make predictions based on what you think those ideas would result in.
Idea 1: 'We can combine the first two steps so that it feels like there are fewer steps and there's less effort.'
Idea 2: 'What if we just removed a step?'
Idea 3: 'What if we can ask this question later on?'
Idea 4: 'What if you just show social proof to reassure the user?'"
3) The Impact Vs Effort Debate
"You want to map these ideas in terms of impact and effort.
How much impact will this solution or experiment have on your metrics?
Unfortunately, this is a very squishy concept since it's really hard to predict what's going to happen.
For effort, you're thinking technically, but also how your ideas might affect other teams.
To measure the effort and impact, we use a two by two matrix and plot our ideas on it."
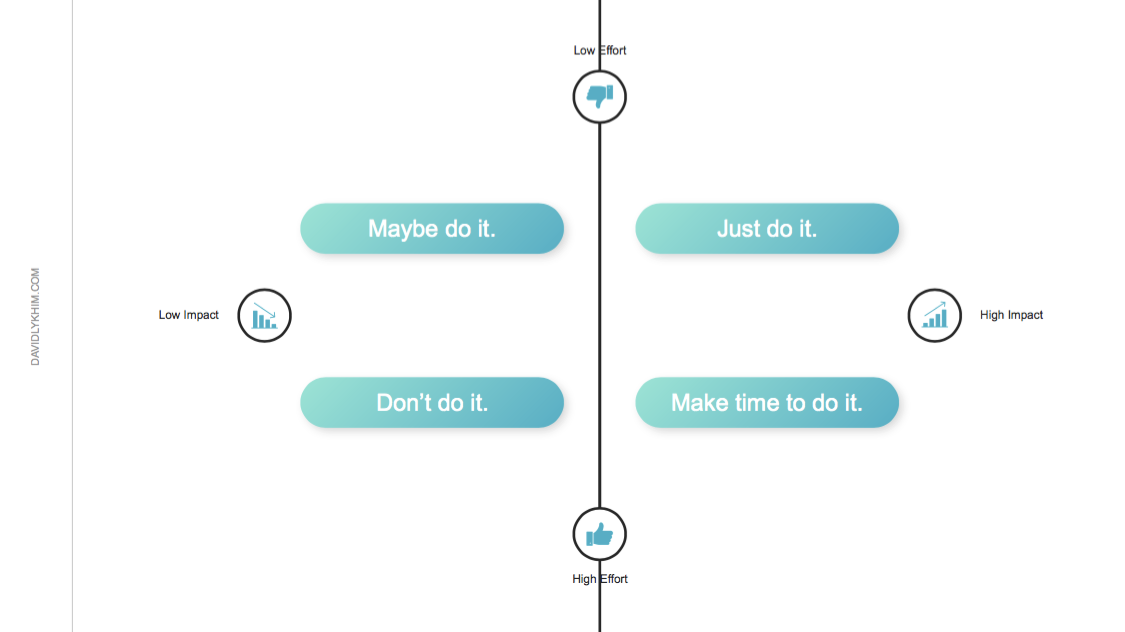
"We take a whiteboard, draw out this two by two matrix, and then have ideas on stickies.
There might be a room of four or five people and we’ll say, ‘is this low impact or high impact?’ At the end of the session, we'll have some quadrants that are more full than others."
4) The Objective Statement
"What do you hope to accomplish by running the experiment?
'Isn't that self-explanatory?' Yes.
But, once you start having a meeting where you're discussing details and very granular issues, you might realize that everyone's completely lost sight of the goal. The objective statement helps you to refocus.
Our objective statement was that we wanted to increase signups."
5) The Design
"The next step is designing the experiment. We need to make sure that we get all the data that we want, and enough of it.
- How many variants will you test?
- How will the experiment variant(s) look?
- What sample size is needed?
Before we tested it out, the signup page was blank.
From there, we had three different variations we wanted to test.
- Included a testimonial from Shopify.
- Included logos of companies we thought would be recognizable around the world.
- Included a bunch of awards that we've won.
We decided to run the experiment for 14 days, get at least 20,000 views to see if that got us to statistical significance."
6) The Predicted Outcome
"You can start as simple as,
'We predict that the completion rate will improve by five percentage points.'
But, we map it all the way down to revenue.
‘If the percentage increases by five percentage points, that will translate to 500 or 300 new signups per week. Assuming that the upgrade rate is 10%, the average sales price is $20, which results in $600 in more revenue per month.'
This helps you really decide whether or not the experiment is worth running."
7) The Peer Review
"This is a meeting with your immediate team and potentially a few other stakeholders.
You’ll talk through all those different items in your document. This is when people can really tear it apart.
What makes you think this is true about our users?' 'Why is this your hypothesis?' 'Do you have data to back that up?'
They'll say, 'maybe your design should be different?' 'Have you considered these different designs?' 'I can help you with that.'
They might even ask, ‘Is this even the right thing to focus on?’
There’s still an opportunity to say this experiment is not worth running.
It's heartbreaking because you've put a lot of time into researching, pulling data and designing everything. But if someone just catches one thing you missed, your experiment is nullified.
The peer review works as a filter."
9) The Go-Ahead
"We can finally begin running the experiment.
We generally run it for at least two weeks, because we want to consider that there might be fluctuations in traffic samples."
9) Post Mortem
"After all the write-ups and the analysis, we have another meeting with the immediate team.
'Why did this experiment end up the way it did?' 'Why was our hypothesis or prediction right or wrong?' 'Why do we think it succeeded or failed?'
At this point, with the HubSpot experiment, our hypothesis was that people were overwhelmed by questions. Our objective was to increase the number of people signing up for our products, and the idea was to social proof. We had a prediction of what we thought the outcome would be.
The results?
The data was inconclusive.
As someone with a product marketing background, I was stumped. Social proof is supposed to psychologically influence people to make decisions. What happened here?
We had to go back to the drawing board. We did some digging, not just from a marketing standpoint, but from a tech standpoint and from a positioning standpoint."
The Problem
"We learned some disappointing information. That, in fact, the signup app took five to 10 seconds to load. It was a legacy product that was built by two different teams, which meant they had two different back ends that didn't talk to each other. In some cases, the second part didn't even load, so people couldn’t finish even if they wanted to.
We also realized that the design didn’t match the website. If someone goes from your website, click Sign up, you'll see a completely different page. It's really confusing. You wonder if you're getting redirected to a page that's trying to hack your details."
Back to the Start
"So, we ran a different experiment. Our hypothesis was that people are getting fatigued when signing up. There's a lot of confusion. And there's a lot of steps. It takes so long to load.
Our objective is still to improve signups, but this time, it's to understand if improving load time and making it look better will improve conversions. The prediction was that, if we improve load time, more people are going to sign up because the app is going to work.
The results of the experiment showed a five percentage point improvement in the sign-up rate. Considering how much traffic the HubSpot website gets, this was a huge improvement.
Reducing the load speed, adding visuals and reducing the friction resulted in more people signing up."
To take from this…
"I talked about how we have a fine filter for running experiments. We're always working to improve our experimentation process. I started off with a failed experiment. And people don't really like talking about failures. But by focusing on what didn't work, you continue to learn and figure out what does work in the future.
At HubSpot we actively avoid something called ‘Success Theater’, where all people talk about are things that work. It makes it seem like everything's working. People then feel awful when experiments don't work, when really that's the reality. We open these discussions and allow people to feel more comfortable pitching new ideas in the future.
In growth it's not just about winning or losing or succeeding or failing. You’re either winning or you're learning. You learn and you learn and learn. And you keep trying new experiments until you win.
In marketing and product, you need to slow down to go faster. If you reflect on what you're working on, and continue thinking about why things succeed, or why things fail, you will continue to grow."
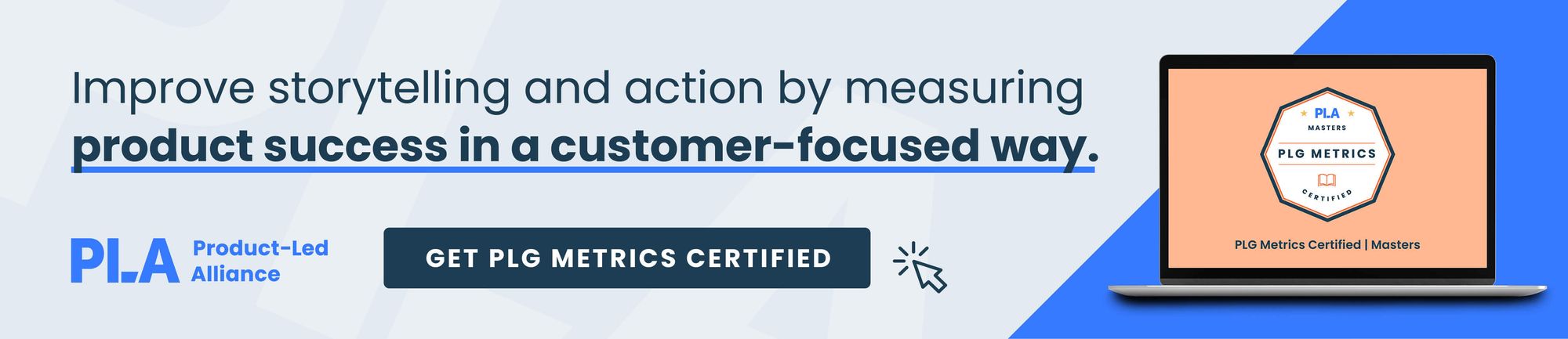