As a product manager, the value of embedding analytics is clear. It provides your product with a key point of differentiation in the market, directly integrates helpful analytics tools so that your users don’t have to go outside of your software for it, and ensures your users derive more value.
But today’s line-of-business users are spoilt for choice when it comes to their data and analytics.
What are product management analytics?
Product management analytics is the practice of collecting, analyzing, and interpreting data to make informed decisions and drive product development strategies. It involves gathering data from various sources, such as user behavior, market trends, and product performance, and using specialized tools and techniques to extract meaningful insights.
Product managers use these insights to understand customer needs, track the performance of their products, identify opportunities for improvement, and make data-driven decisions throughout the product lifecycle.
Product management analytics plays a crucial role in optimizing product features, prioritizing development efforts, and ultimately delivering products that meet user expectations and drive business success.
What is embedded analytics?
Embedded analytics features are like the hidden gems inside your software or applications. They're the power tools that allow users to access data insights and analytics without ever leaving the platform they're using. These features seamlessly integrate data visualization, reporting, and analytics capabilities directly into your product, making it easier for users to make data-driven decisions on the spot.
Embedded analytics features are like having your own data analyst on call, as they provide real-time access to relevant data and help users uncover valuable insights within the context of their workflow. Whether it's tracking performance metrics, monitoring customer behavior, or evaluating business outcomes, embedded analytics features bring the magic of data right to your users' fingertips.
After 20 years of using standalone dashboards and reports (built mostly by IT), a wave of modern embedded analytics solutions has empowered many to build reports, explore their data themselves, and make analytics feel like a core part of the applications they use for work.
However, with this leap in new analytics options brings a new challenge: Users expect more.
Embedded analytic features
The increased accessibility of sophisticated analytics features, from automated monitoring to AI-generated insights, means dashboards and reports on their own no longer cut it in the search for insights. It also means your product’s analytics now require more to stand out from the pack.
While not every product needs to have the latest analytics features to realize success, gaining a competitive advantage, keeping future-proof, and increasing revenue in this area require being up-to-date. If dashboards are the extent of analytics your product offers today, now is the best time to recognize there are opportunities to expand your capabilities and meet the new baseline.
Embedded analytic tools
Embedded analytic tools play a crucial role in empowering product managers with valuable insights and data-driven decision-making capabilities. These tools seamlessly integrate into the product management workflow, allowing professionals to analyze and interpret relevant data without the need for external platforms or complex processes.
By embedding analytics directly into their work environment, product managers can effortlessly track key performance indicators, user behavior, and market trends. This accessibility enables swift and informed decision-making, as product managers can quickly identify patterns, assess the impact of new features, and optimize their strategies in real-time.
Moreover, embedded analytic tools foster collaboration within cross-functional teams, as stakeholders can easily access and comprehend the same data, fostering a unified understanding of the product's performance. Overall, these tools contribute to a more agile and responsive product management process, helping teams stay ahead in the dynamic landscape of today's markets.
The constraints of an ad-hoc approach to embedding analytics
For some vendors, analytics, in general, is never made a priority consideration. The focus may have been on building the initial Minimum Viable Product (MVP) and getting it market-ready, or there’s an intention to add some form of analytical capability later. But typically, it’s left to developers to add in analytical features piecemeal, and to the users to request them.
The problem this ad-hoc approach creates is you may not have an accurate understanding of your product’s current analytical offering, or of what both groups need to make adding a feature worth it - or possible, depending on the state of your data structure and integration capability.
Using the previous example, your product may have had some form of reporting added on over time, but there’s a big difference between adding on a data export feature for users to pull data and use it in another external analytics tool, and a fully embedded analytics module that sits alongside your core product experience.
Without some formalized process and effort made to guide the introduction of analytics at every phase, your product’s analytical maturity could be at an earlier, less sophisticated stage than your team believes it to be, and your users may ultimately not be getting the kind of reliable data and discoverable insights they expected.
Understanding analytics in your product stands today
Of course, understanding what a new analytics feature can bring to you as a product manager, or when you’re ready to make a move, or even how capable your product’s analytics is today, isn’t always simple.
This is why we made the Embedded Analytics Maturity Curve, a strategic framework and visual roadmap that helps formulate your analytical development, adoption, and long-term strategy.
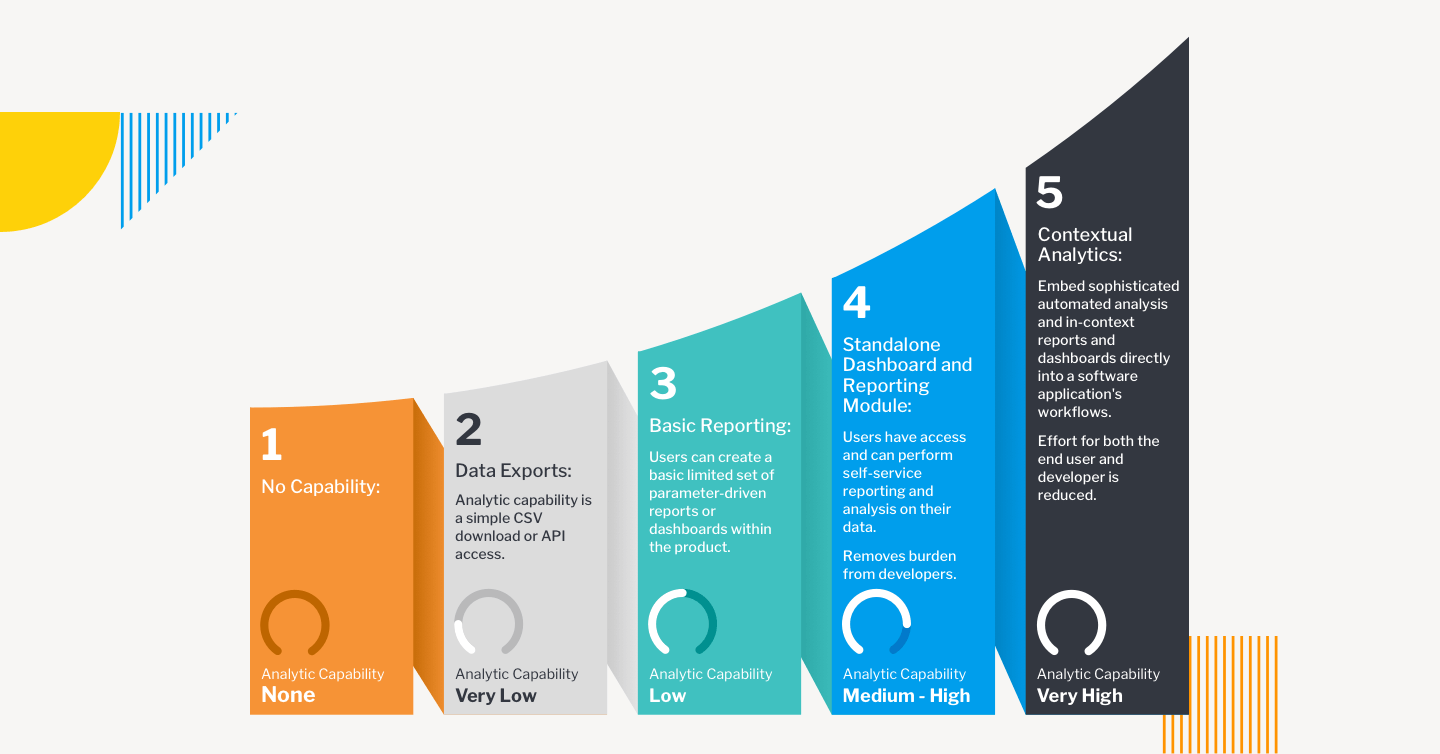
The Curve exists to take product teams through five defined phases of the overall product journey, based on our extensive knowledge gained from helping our customers, to help you understand the requirements to action the most effective and efficient introduction of embedded analytics into your software product possible, whether it is existing software or a new product.
Embedded analytics for SaaS
The benefits of using a progress model, for your product’s analytics pipeline, rather than approaching analytics as an ad-hoc add-on, are numerous, and aim to assist teams to think about and assess key areas that may be overlooked or neglected. Some examples include:
- Identify your data access and structure: Does your product offer users easy access to their data? Is it cleansed and usable to support self-service consumption? Analytics starts with data, but it’s common for product teams busy with other priorities to not have the full picture of what their data actually looks like, how it is structured, or where it’s captured and stored. Using the Curve helps your team understand it’s not simply a case of offering features, such as data exports or basic reporting, that determine whether your product offers analytical value, but also the readiness and reliability of your data assets to allow your users to derive accurate insights.
- Understand what user insights look like: Are users exporting their data from your product into another to derive value? Can they explore their data and find answers when using your product? The Curve assists in defining the differences in what insights look like in each stage and can help you pinpoint what data wrangling is required to meet the requirements for more accurate, sophisticated decision-making and reporting before you can move to your target state.
- Evaluate future analytics scenarios: What typical priorities or roadblocks does the product team need to address before adopting a new analytics feature? What kind of challenges do you face when updating your software with the latest features? The Curve breaks down the many nuances of each stage of maturity for a clearer picture of what to expect, what to address, and whether a move to the next stage is appropriate right now, to minimize rushed transitions.
- Know when you’re ready to update - and why: Your data model is highly performant, and you have the internal expertise to embed analytics the right way. Great! But why should you update? The Curve covers the many reasons you should consider moving from one stage to the next - from users struggling to build reports to customers churning to competitors in their search for a better option for analytics consumption - that may not be immediately clear to your team.
How product managers can use analytics
Many product teams still lack a full picture of the state of their data, users, and analytic technologies, but pursuing and implementing meaningful updates to your analytics can only match up to the new, modern expectations the self-service analytics revolution has brought can only be done when you understand and can articulate your current and target capability.
If you need help in getting started, the Embedded Analytics Maturity Curve can provide valuable guidance in what, when, and how you can mature your software’s analytical capabilities, and even be a much-needed wake-up call for the rest of the organization to get on board.