This article is adapted from Jamie Norman's talk at the Product Led Summit, London 2019.
AI: the Bad Guy.
"Hollywood and Artificial Intelligence have shared a long and arduous relationship. Reflect on these movies. What's the common theme?
Terminator, iRobot, The Matrix, Ex Machina, Avengers: Age of Ultron.
If you've lived under a rock for the past twenty years and haven't watched any of them, spoiler alert, AI's the bad guy.
Historically, within both literature and film, the rise of a technology that can act of its own accord has proven a malevolent plot device. Frankenstein's Monster, set on destroying that which created it, is something that has stricken fear into mankind for centuries.
The unknown, all-powerful 'AI' is the enemy. The human is the hero. Now, let's picture the widespread horror when AI actually begins to become a part of our reality.
The past ten years have seen the industry explode. You can't go to a marketing trade show or a tech trade show at the moment without seeing AI plastered on billboards and on posters.
The movie Blade Runner, made in 1982, was actually set in November 2019. It's now a period drama. To be honest, I'm disappointed that I don't have a flying car. But, we do have digital billboards now. Small victories.
It's fair to say that popular culture doesn't paint the best picture of AI and the unscrupulous use of data. So, we are faced with a bit of a credibility problem. Whether that derives from its fictional representation, the nefarious use of personal data or the truck loads of privacy legislation that come hand in hand with this, it is obvious that people value transparency when dealing with AI.
Even words like ‘algorithm’ now have a negative connotation.
We see headlines like this: ‘Apple Card algorithm sparks gender bias allegations’ and we worriedly wonder, 'Why are tech companies using our information against us?'
Working in tech, we've got a lot of work to do to clean up this reputation.
I'm going to share our journey at RedEye, a marketing automation service, as we tried to introduce new, potentially daunting technologies to our customers.
Machine learning versus artificial intelligence
First, let's clarify the terminology that we'll be using in this article. The terms machine learning and AI are often used interchangeably. You might have wondered where the difference actually lies.
When you're talking to the developer, it's machine learning. And when you're pitching to VCs, it's AI.
That sums up where the market is positioned. AI is becoming somewhat of a bandwagon. It's a powerful trend.
So, is there a real distinction or not?
Artificial intelligence is a technique that enables a machine to mimic a human. We see some companies cynically using 'AI' as a marketing strategy because anything that you're using as an automation, could be argued as AI.
Machine learning is a subset of AI principles that allows you to apply statistical techniques to enable machines to improve with experience, whether that's supervised by a person or not. This is where we get into matters of 'deep learning'.
I'm going to focus on machine learning and outline how exactly we've implemented it in our business and, more importantly, made it accessible for our customers.
1) Accuracy Versus Explainability
Different machine learning models: there's a lot to choose from. And, there's pros and cons for each one.
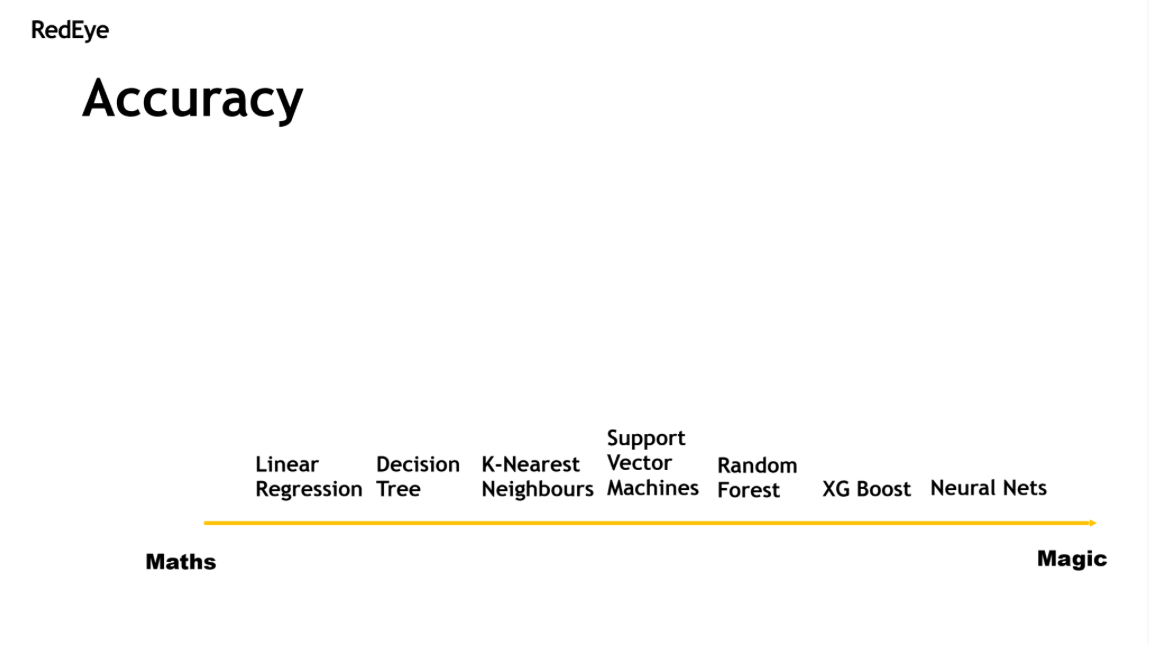
Let's put our models on a continuum like this. On the left, are the less accurate ones. The more you move to the right, the more accurate they get. But, the more accurate they become, the greater the weight of data that needs to be in place to support them.
On the left, you can describe the model in terms of pure maths. Basic, explainable. On the right, it functions differently. Even people working with neural networks can explain generally how they work, but can't explain exactly how they get there and what they do and how they're doing it. Remember, these are people that are academically working in this field.
There's a famous quote from Arthur C. Clarke, British Sci-Fi writer.
‘Any sufficiently advanced technology is indistinguishable from magic.’
The more accurate a machine learning model is, the harder it is to explain. The more ‘magical’ it becomes.
Give me the magic product...right?
We can’t forget how important the notion of trust and credibility we were talking about earlier has become in the tech industry. How can we realistically expect customers to adopt these principles that can't be explained to them?
Transparency is paramount, and the more explainable something is, the more transparent it is.
There's a trade off between how accurately you want the model to perform, versus how explainable you need to make that for a customer.
2) Start on familiar territory
If you start from a place of familiarity, you've got somewhere to ground your feature and the customers will come along with you because they've got that frame of reference. At RedEye, we were looking to introduce predictive models to our product.
We were thinking 'How can we help our customers better predict the behaviors of their customers?'
This is very new tech. It's difficult for people to grasp what's going on. So, we needed to lead them along the way. Our own awareness of this necessity actually arose from challenges we had previously, where we went too far in terms of where the customers were in their own understanding. We knew we had to simplify and that it would prove valuable to start at a familiar place.
We had a lot of discussions within the business around how we built the interface for this predictive tool. We did a lot of work with our analyst team and our data scientists with testing the models outside of the tool with some of our customer data. The business wanted to turn this into a flagship product. It needed bells and whistles.
We had a lightbulb moment in terms of how we would introduce this technology to the product. We really needed to know, 'Who are the people that fit this profile?' This must be something they're already familiar with and it must relate to the work they’re doing right now so they have a previous understanding to ground them.
So, we started with just adding a new segment rule. It was really familiar territory because it was part of the tool they were already using, in a way they were already using it. We added one predictive model into that tool, which was
'prospects likely to purchase in the next 30 days.' A user can go to the segment builder, and create a rule that says ‘people with a score that is greater than x.’
They were using machine learning, but they were using it in a way that was familiar to them. It wasn't scary and it got results. It also put them in control. They could layer other segment rules on top of this. They weren't at the behest of a secret black box.
When we started talking about this, we were saying 'You could just throw all of the data into a machine learning model, and it will just tell you who the people are that you need to target.' But, remembering how important explainability is in this industry, it just wasn't going to work. It was too too big a stretch.
3) Real World Outcome
We also wanted to build to a real frame of reference that applied to the customers and their goals. But, when we build more models and add more depth and detail to this, how do we still keep frame of references to them?
We ended up building six models that we mapped specifcally to a customer journey. These included
- Probability of making a first purchase within the next 30 days.
- Probability of making a second purchase within the next 90 days.
- Probability of becoming a VIP.
- Predicted lifetime value over the next 12 months.
- Probability of becoming a lapsed customer.
- Probability of unsubscribing within the next 90 days.
Marketers are interested in understanding, 'How do we make sure we optimize every step of the customer journey?’ The compartmentalized six models provided that unique understanding.
4. Build trust and iterate.
There are different types of people who have different working styles. Don't forget this.
Some of them want to know what's under the hood. Lots of our customers began asking for more detail and we gave them that data to work with. We would guide them on the way and answer their questions. We would usually start quite small, showing them the best practice and not overwhelm them. It was a growing process.
Other customers were just not interested in how it worked, but were only concerned that it did.
'Is it working for me?' '‘Yes?’ ‘Okay, great, move on.’
Most importantly, to each customer, we gave enough information to be transparent and so people felt like they were in charge.
5) Don't be evil
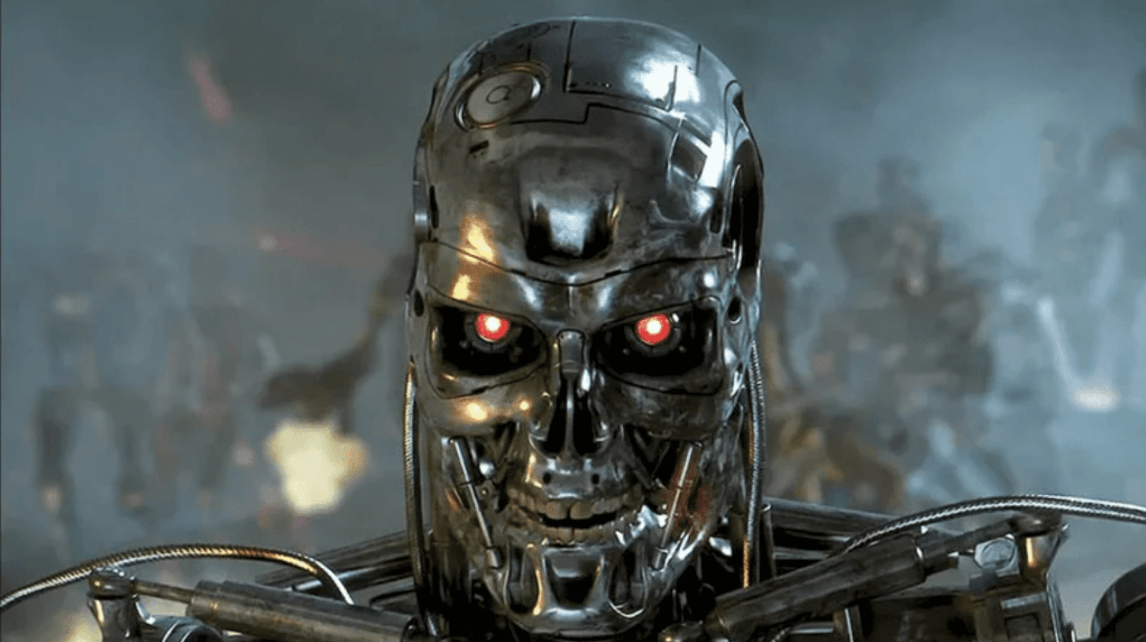
There's a number of challenges facing AI in terms of ethics and the application of it.
Have you ever been talking about, or even just thinking about a product. You click online, and 'Oh!' There's an ad for it on your homepage. It’s creepy. People begin to distrust the technology.
AI has the power to transform tech and computing, but the ethical questions concerning how it’s utilized are going to come under a lot more scrutiny. Jeff Goldblum said it best,
'Just because you can, doesn't mean you should.' (Dr. Ian Malcolm, Jurrassic Park)
There still remains a difference of people's perceptions regarding AI. Some see that this augmented intelligence is an enhanced tool that helps them do their job. Others back away from it, dubious of the free thinking machine. We need to work on providing an AI that is accessible to all and we must help it to be widely understood as an enhancement to our working lives.
As a technology that facilitates better information sharing and decision making, it doesn't have to be scary and it definitely doesn't have to evil. But remember, it's up to us to make that happen."
Jamie Norman is the Head of Product for Red Eye, a marketing automation platform working in the retail, financial services and travel and leisure industries.