In today's tech-driven era, the buzz around artificial intelligence (AI) is undeniable. From enhancing customer experiences to automating complex tasks, AI has made its mark across various industries. But as businesses race to integrate AI into their products and services, a critical question arises: Is artificial intelligence truly the best solution for you as a product manager?
In this article, we'll explore the key considerations you need to weigh before diving headfirst into the AI bandwagon. By the end, you'll have a clearer perspective on whether AI is the right fit for your specific product needs.
Understanding the AI hype
Before delving into the nitty-gritty of AI integration, it's essential to grasp the extent of the AI hype. AI has indeed revolutionized many sectors, delivering impressive results in areas like predictive analytics, natural language processing, and image recognition. However, it's vital to distinguish between the promise of AI and its practical application. Not all problems can be solved with AI, and not every product benefits equally from its implementation.
Assessing your product's needs
The first step in determining whether AI is suitable for your product is to evaluate your product's unique needs and goals. Consider factors like your target audience, industry, and competitive landscape. Are there pain points that AI can address effectively? Will AI provide a significant advantage over traditional solutions?
For instance, if your product revolves around data analysis and pattern recognition, AI may offer a substantial advantage. Conversely, if your product requires minimal data processing, AI might be overkill.
Costs and resources
AI implementation is not without its costs, both in terms of time and resources. Developing and maintaining AI models can be resource-intensive. You'll need a team of skilled data scientists and engineers, along with the necessary computing infrastructure.
Additionally, AI models require continuous training and monitoring to remain effective. Consider whether your budget can accommodate these ongoing expenses and whether your organization can commit the necessary time and expertise.
Data availability and quality
AI heavily relies on data to function effectively. Assess the availability and quality of the data you have access to. Is your data clean, comprehensive, and representative of your target audience? Lack of quality data can hinder AI performance and lead to biased outcomes. Moreover, consider data privacy and compliance regulations, as handling sensitive data comes with legal responsibilities.
User experience and acceptance
AI can significantly enhance user experiences when applied thoughtfully. However, it's crucial to gauge whether your users are ready for AI-powered features and whether they will positively perceive these additions. An overly complex AI interface or overreliance on AI may alienate some users. Conduct user research to understand their preferences and expectations.
Alternative solutions
Finally, explore alternative solutions to address your product's needs. Sometimes, simpler and more cost-effective methods can achieve similar results. Don't overlook traditional technologies or approaches that may be more suitable for your specific use case.
Time to ask the professionals…
We posed these questions and more to experts in the field to get their advice.
- Dan MacKenzie, Head of Product, Altruistiq
- Chris Butler, Lead Product Manager at Google
- Megha Rastogi, Group Product Manager at Okta
- Alessandro Festa, Senior Product Manager at SmartCow, and Co-Author of K3ai
- Deepak Paramanand, Director of Artificial Intelligence at JPMorgan Chase & Co
Main talking points:
- Why do orgs sometimes implement AI unnecessarily into their product?
- How can PMs verify and validate results to justify the use of AI/ML?
- How would you measure whether AI is truly improving the user experience?
- Do you have any tips for convincing non-technical leadership when AI is not appropriate or needed?
- What are the best ways to predict the cost of AI/ML solutions?
Why do orgs sometimes implement AI unnecessarily into their product?
Dan MacKenzie: Amongst several reasons, the primary cause is often a hope that it will be a panacea to solve other problems with the product. This goes back to the “if your only tool is a hammer, every problem looks like a nail” proverb - really worth checking before investing heavily in hammers if your problems are actually nails that need hammering in.
How can PMs verify and validate results to justify the use of AI/ML?
Dan MacKenzie: This is a super important task, especially when working with AI/ML approaches that are more towards the “black-box” side of the spectrum. Verification checks that the assumptions and calculations within the model are being carried out correctly, so we can look to make sure that the model is producing expected results when given known inputs.
Do this across several different dimensions (including known edge cases), with the aim of not just confirming positive results, but also finding negative results from areas where the model is expected to break down. Starting with simple inputs and working up in complexity is key here.
Validation checks that the model is accurately representing real-world behavior, so we can use known inputs and outputs to test end-to-end model-to-reality correlation for this, either through using real data from past situations where possible, or manufactured scenarios that we can test and model in both the real and virtual worlds.
Chris Butler: Usually through qualitative means first. If we don’t focus on the interaction of the non-deterministic technology (AI/ML) with the people that they are impacting we will build solutions that are in the area of “abuse” for users as Parasuraman discusses in his seminal 1997 paper “Humans and Automation: Use, Misuse, Disuse, and Abuse.
After the impact of the systems on people you can judge if the system is performing within expectations using traditional metrics. There is value in collecting more machine learning-specific metrics but I think that should be left to the model engineers. As product people, we should have a tradeoff discussion with those engineers about what it means to have more false positives than negatives.
Deepak Paramanand: The first thing, like in any product scenario, is to test first. There’s a whole new era of prototyping, design, sprint, etc, that’s come through and is even more crucial in AI products. The simple logic is AI-first - test first. Even before you go there, for example, when you’re trying to predict the success and engagement of customers, let’s say you draw that up. You have a customer with a five-month history. Let’s say you get the data and you chart up the end state saying - if I generate synthetic data, if I build AI, then I’m going to say more caucasian males, less black females are going to use it.
Now test it out. How does that sound? Does that sound good? Does it sound right? Does it sound appropriate? If it doesn’t, reword it, just to get your morality and your compass right. Evangelize across your team, explain what you’ve thought of, why you’re not comfortable with this, and what you’ve thought of as the substitution and why.
Show them the bad and the good, but also show them the journey. That way, you get to tell people in the company to rally around and say - your good is not good enough, let’s be better. Then testing comes in - go test it with your customers and ask them - what if we say that about you? Are you comfortable? Even if you don’t want to talk about it, can I go and engage you in any way?
Then you get to the best state. So I’d say always keep testing. Test the end state, test what you want to come out of it, etc. As PMs the messaging is important. How am I perceiving it? How am I messaging it? How am I hearing it? How am I telling it? So test that messaging and the end state and then work backward. If you want to predict customer engagement but the messaging leading to it is not good. Either shelve the problem or go after a different problem and try to solve that.
How would you measure whether AI is truly improving the user experience?
Dan MacKenzie: This question can be abstracted from AI in many senses, the set of approaches and tools we think of as “AI” are all merely levers that impact the experience of users in our product. Here we can go back to our well-established behavioral-based indicators to measure engagement across a variety of metrics.
Interview and speak with users, understand how changes may have touched their user flows, and iterate from there. AI presents certain challenges from an explainability, understandability, and predictability standpoint, but none of these are insurmountable.
Do you have any tips for convincing non-technical leadership when AI is not appropriate or needed?
Megha Rastogi: We don’t need to incorporate AI or ML just because it sounds cool. We should instead be clear on solving the right problem. And those solutions may not be ML, so that’s something that you should be open-minded on. For ML problems, you want to focus on problems that would be hard to solve with traditional programming, or rule-based programming or heuristics, etc.
ML is a learning and adaptive system and thus, can adapt to changing trends as new data inputs keep coming in. So distinguish between automation problems and learning problems. ML can help automate your process. But not all automation problems require learning. It’s important to always keep that in mind.
Dan MacKenzie: It’s imperative to first understand the rationale behind why AI is being considered - if we map against Marty Cagan’s 4 big risks (valuable, usable, feasible, viable), which areas does an AI-based approach offer significant advantages over other approaches. (N.B. we also need to define more specifically what we mean when we’re talking about “using AI ‘’ right at the beginning of this process).
Once the conversation is framed in these terms, it provides a useful structure to discuss and debate where an AI-based approach may win out over other approaches, for instance by showing that a certain swathe of approaches may lack viability due to the engineering constraints around available data needed for certain insights.
Chris Butler: The biggest problem I see in the adoption of AI is the lack of the necessary amounts of good data. I’ve personally observed people wanting to use AI but the amount of data they have already collected or could collect in the near future isn’t enough to train a model. If the task you are trying to automate is very rare and there isn’t a chance to collect it in the wild very often you will have a really hard time training a model that helps rather than hurts.
I’d always start first with really simple attempts at solving a problem. There are benefits to complexity but it should be evolved over time, including using machine learning, rather than from the beginning. As Gall’s Law states: “A complex system that works is invariably found to have evolved from a simple system that worked.” You can’t build a complex system from scratch.
What are the best ways to predict the cost of AI/ML solutions?
Alessandro Festa: It depends a lot on what kind of use case you’re solving. For example, most companies these days are focusing on NLP, digital assistants, or whatever you want to call it. So there’s this idea that if you build in this digital system, you basically place it as an automatic chat on the website, and when the customer goes there, you can engage them and create the lead.
This is pretty simple because you’re typically using a pre-trained model. What people typically don’t know is that even if it is a pre-trained model, you still have to refine the model, you still have to plug the results of the chat into your back-end system, etc. And it’s a big cost.
That bot runs somewhere, so this is a cost for you. Also, it becomes a critical role for your business because a customer expects to have this bot up and running all the time. So you need to work out the high-level architecture. The second thing that you have to consider is that AI is a competitive market. There are still very few roles and specific skills out there, so that means those people can be quite expensive.
Companies can assume that when they have someone great with numbers, that person can just be a data scientist now. It doesn’t work this way. You need to end up hiring someone and evaluate what you need.
If you’re building a product where you’re also building the model, then it’s even more expensive. In the early days, people assumed that running on the cloud was less expensive. These days, these approaches change. One of the things that we observe is that it doesn’t matter where you run your code, it matters how you run your code.
There are different approaches - you can have a super-powerful machine, so you try to cut off the training times. You have to keep in mind that when you’re training something, it goes for hours, if not days, continuously. So if you’re on a cloud or pay per minute, pay per hour, then it becomes really expensive. And this is an iterative process, so it’s something you have to do every time.
Last but not least, you have to realize that you cannot run something else on that machine, it’s a dedicated machine. So those are the things that you need to evaluate. My struggle as a PM for AI, at least in my experience, is that those calculations are not easily done. Especially at the beginning, and the first time you develop a product, you have no idea what kind of hardware or software you’re going to use, what kind of infrastructure, etc.
You need to have a clear vision of your product, explain this to engineers, and then work out the high-level architecture with them. That will give you an eye-level cost. And again, you need to use the stakeholders to set expectations properly.
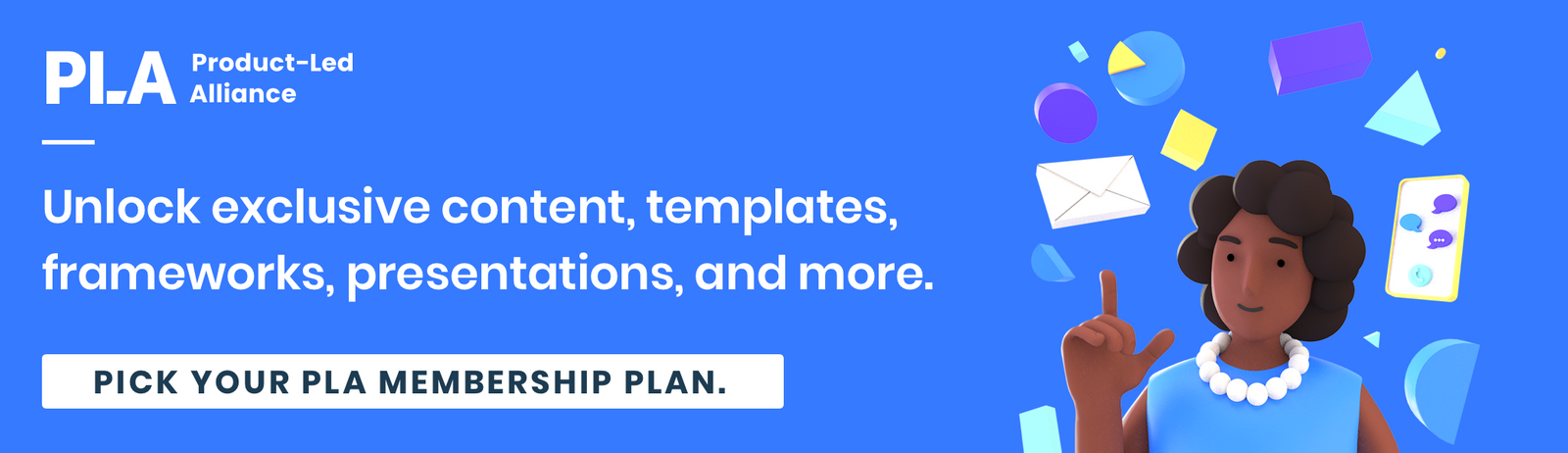